Warren Hatch on Seeing the Future in the Era of COVID-19 – #50
Corey: Our guest today is Warren Hatch, President and CEO of Good Judgment Inc. Good Judgment is a pioneering firm in the field of political, economic, financial, and now, public health forecasting. Previously, Warren was a portfolio manager at Morgan Stanley, later a principal at Catalpa Capital/McAlinden Research. He has a Dphil in Politics from Oxford, an MA in Russian and International Policy Studies from Middlebury Institute of International Studies. Warren has a BA in History from the University of Utah. He’s a licensed CFA. Welcome to Manifold, Warren.
Warren: Thank you, Corey. How are you today?
Corey: I’m doing all right. It’s been five years since the publication of Philip Tetlock’s book, Superforecasting, and 15 years since the publication of Tetlock’s book, Expert Political Judgment, two books that sparked what I call the forecasting revolution. So, I would like to get in to what’s been happening since those books were published and your leading role in developing the commercial arm of the project.
Warren: Okay. Yeah. Going back to the meso-time. So, Good Judgment Project was a research initiative led by Professors Philip Tetlock and Barbara Mellers, who were at the University of California Berkeley and then moved to University of Pennsylvania in Wharton during the research project. So, both universities were involved in the research project for that team. They were one of several teams in a tournament sponsored by the US government, the US intelligence agencies.
Warren: What they wanted to find out, the US government, was is there a way to improve on the wisdom of the crowd when we’re thinking about uncertain events. They launched this research project at the time when they were doing some soul-searching about some policy and intelligence forecast that they had made that did not show them at their best, weapons of mass destruction, 9/11. Those are pretty significant intelligence failures, and they wanted to learn from that.
Corey: So, Warren, I just want to stop for a second because I think this is worth emphasizing, right? People do worry about whether the US government really took seriously the failures of those two serious intelligence debacles. This whole project came out of the recognition that something went seriously wrong and need to be improved. Is that a proper statement?
Warren: Well, I wouldn’t want to speak for them and what their motivators were, but it is clear that they were doing some soul-searching and they were looking genuinely for ways to improve their forecasting skills. It was an open question, “Can you do better than the wisdom of the crowd approach to get accurate forecast?” because it does work. We see it in many places. There’s a long literature on wisdom of the crowd. You see it in Who Wants to be a Millionaire. Always ask the crowd, don’t phone a friend. We’ve seen it in other spheres as well.
Warren: So, it became a testable proposition, “Can you do better?” They sponsored a research, the US government, that’s very speculative in nature. They genuinely did not have a preconceived notion of whether something useful would come out. Indeed, for the other research teams, they failed to reach the goal set by the US government to improve on the accuracy of the wisdom of the crowd. Some did and came close in the first year, but by the second year, none of them had.
Warren: So, what the US government did was they said, “Okay. Team Good Judgment is clearly onto something. Let’s consolidate resources there.” What was that something? The something was to take a very empirical evidence-based approach.
Steve: Sorry. Could we describe the mechanics of the term because I think our listeners maybe aren’t even aware of what a prediction market or a prediction forecasting tournament is like, which is just the basic mechanics, which I think you’re saying Good Judgment won?
Warren: Okay. So, the tournament that US government sponsored invited a number of teams affiliated with the universities and other organizations in the private sector to come up with ways to improve on the wisdom of the crowd. They were free to deploy any tools or techniques that they thought would work, and they’re required to do is at the end of every day deliver a forecast on each of the open questions that were set to the different tournament institutions. So, they’re all forecasting on the same questions, “Who will win this election? Will there be an outbreak of violence in the South China Sea?” these sorts of things on a certain date. That was the one requirement was every day, submit a forecast.
Warren: Some teams thought that prediction markets were the way to figure it out. Other teams have different ideas. What team Good Judgment did was tried them all. They tried an individual condition where if you’re a forecaster, you’d get the question and you’d be left to your own devices to come up with a probability estimate. You would not be able to compare your forecast with others. You would not be able to compare your rationale with others. So, that was one research condition. That was a tough one. Some really smart people came out, but the thing is that that research condition was inferior to the others, two other main ones.
Warren: Next one is prediction markets. That’s where if you have a view about an event, you can go and bid on it. So, if, say, the US election outcomes is trading at 60 cents on the dollar in favor of the Republican and you think the Republican is going to win and that that’s a low price, you’ll buy it. If you’re corrupt at the end of the period, you’ll get a dollar if it occurs, and if it doesn’t, you’ll lose it all and go to zero. That’s the way prediction markets work.
Warren: They also allow you to trade during the time that the question is open. So, if, for instance, I see that 60 cents and I think that the crowd and the prediction market has, for some reason, overreacted one way or another in this event, I may bid against it. Quite distinct from what my own prediction is about the election outcome, if I think the crowd at this moment in time is mispricing it, I’ll go trade against it and make some money on it.
Warren: So, in that case, my incentive is to retain any information I have and not share it. In fact, I might even be inclined to share disinformation if I think it will help my position. We see this on prediction markets.
Warren: The third version, which I think is where the magic really happens, it’s not what I think, it’s what we know, it’s in the data, is to have teams, teams of forecasters working together and collaboratively. In this case, the output is not my individual forecast, it’s a team-based forecast. I’ll have my own, to be sure, so I want to beat my team members, but, really, my team, we want to beat all the other teams, right? We want to be more accurate.
Warren: So, now, the incentive is to share information. This is really an important feature, especially if you have a team with cognitive diversity. We’re all coming at it with different pieces of information or consider a forecast question as a mosaic, and we need to fill out the mosaic with our forecast. If we each have different tiles to contribute to the mosaic, that does a few things.
Warren: It means if I have a tile, you don’t need to contribute it, it’s already there, and vice-versa. So, we accelerate the process by sharing information, pulling our limited information. Also, if we have different perspectives, that means you may be bringing a tile to the mosaic that would never occur to me. So, I’m benefiting from that cognitive diversity and accelerating the learning process for us all. It turns out that that’s a great way to generate accurate insights.
Corey: So, on the one hand, you’re arguing that the real power of the approach is combining forecasting into teams and sharing information, but at the same time, you recognize a certain people recognize as superforecasters on their own. So, how do people distinguish themselves if they’re sharing information with others in a team? It seems like it’s pretty to copy somebody else. Was there a case in which the studies ran people individually and they got superforecaster status by themselves or were they always parts of teams when they became superforecasters and would always take advantage of information that was out there, essentially in the small public that was on the platform?
Warren: Sure. So, the superforecasters in the research phase came from all the different research conditions, and including individuals when they’re just working completely on their own. There are some very talented superforecasters who came out of that condition. You could see that by their individual scoring on these forecast questions. So, there were some in that individual condition who definitely did better than the rest in that individual condition. That’s how they identified the superforecasters. There are some people who were just consistently better than the rest.
Warren: So, for the individual conditions, there were some who were consistently better than the rest in that condition, but they had their hand tied behind their back. They underperformed, the general forecasters, as well as the future superforecasters, in the other research conditions, where that same dynamic started to show up in the research.
Warren: So, that became a new research question. It wasn’t part of the original design. They go, “Wow. What would happen if we identified the best of the best, and then put them together on elite teams? Would they revert to the mean. There were many who thought that would occur or would they continue to get better by being around similarly motivated individuals, and it turned out to be the latter. The superforecasters who were put on that first team in year two continued to get better, and they did the same thing in the following year, and observed that that first cohort continued to do better than the new cohort, all the way through.
Warren: So, it’s a process that as individuals and as teams, we can continue to get better by constantly getting feedback from the forecast questions that are posed. Now, you raised a good question, though. Well, maybe the easy thing to do is, to be a superforecaster, is just to forecast the medium, whether it’s on Good Judgment open with the overall crowd or when joining a superforecasting team just to do the team. You’re absolutely right. Superforecasters themselves are pretty smart and will detect people who are doing that pretty quickly.
Warren: Really, the superforecasters themselves, once they get to that point, if really all they wanted to do was cheat the system a bit, they would have dropped that a long time ago because the motivation really is largely the challenge of getting better being out on the scientific frontier to improve forecasting and learn new things. If you’re a free rider, you’ll get bored pretty quickly and drop away. To be honest, it’s not a problem we’ve observed to be material.
Steve: I think, Corey, you could detect value add per individual, so deviation from median forecast. So, someone who’s just doing the median forecast is not adding value relatively because occasionally, somebody who’s really got an independent way of getting to their forecast will occasionally produce some deviant forecast, which turns out to be correct, right? To what extent did the government actually incorporate these learnings from the original project into what is actually happening at DNI or CIA or NSA, places like that?
Warren: That’s a good question, but first on having people deviate from the median on a forecasting team, that’s really important to be able to have space for people to express their own view, especially when it deviates from the median because this is one of the protections against group think and other risks like that from having a group is allowing people to challenge what might be consensus thinking, and by using the median, in particular, you make space for people to do that. Just a simple choice of a mean versus a median is quite consequential here. If you use the median, people who deviate widely from the group will move the group’s forecast, and the rest of the group might resent it a little bit, but by using the median, it protects the space for people to have different views and express them.
Warren: If they are right, we’ll learn from that, and next time on a question like that, we’re going to pay more attention to them and vice-versa. If over time somebody is pretty far out there and they’re consistently not doing well, well, the rest of the team will pay less attention. It’s also going to show up in their scores. So, they’ll take that feedback on themselves and begin to self-correct. That’s one of the wonderful things about getting good and accurate scores.
Corey: I don’t want to get too technical here, but the basic metric you use for assessing forecasting accuracy is the Brier score, essentially, square deviation from reality. So, forecaster forecasts that there’s a 70% chance it will rain tomorrow and let’s call raining a one, not raining, a zero. If the rains drop at 0.3, you square it, it’s 0.9. That’s the Brier score, right? That divides X into two components, calibration and discrimination. I think discrimination captures your willingness essentially, too, as we go all in.
Corey: Our listeners are pretty sophisticated, so I think they can capture the fact there are multiple dimensions to it, but am I right in thinking that you look for people both are willing to essentially not just track the mean prediction and often forecast it to do very well? You got to make pretty extreme forecasts if you think something is highly likely.
Warren: Right. Yeah. So, the two terms that the researchers have used to capture the two drivers is calibration and resolution. Calibration is like what we see with good weather forecasters, right? So, if they say there’s a 70% chance of rain next week, and they do that over 10 weeks, what are we going to find out at the end of each week? Their 70% forecast align 70% of the time with what the weather actually did, and three times not. So, in that sense, we’re looking if we’re well-calibrated for things to happen at the frequency in which their forecast and not to happen at the frequency where they’re not forecast. That’s very important, and it becomes a problem, too. It’s a challenge out in the world because if I say 70% chance of rain and it’s sunny, well, you are wrong. I shouldn’t pay attention to you.
Warren: That’s what we’ve seen with some of the higher profile questions, too, with Trump and with Brexit where superforecasters and others, too, like Nate Silver, FiveThirtyEight, were fairly moderate in the forecast, but still said in the last election the Democrat would win, and the Republican won. So, you’re wrong, and it was very much the same 70% and 30% split. So, that’s the calibration, though. If you’re well-calibrated, you should expect those events to occur at the frequency in which their forecast.
Warren: Resolution takes it another step. That is when you are justifiably decisive in your forecast, you will move to more extremes in your forecast. So, what’s a one way to think about that? Well, regular forecasters tend to stay close to the 50% line. They might move a little up, and they might move a little down, but roughly, during the length of questions being open for regular forecasts, and these are good, and they’re on Good Judgment open and the like and getting a lot of good feedback, but about half their forecasts will tend to be between 35% and 65%, right? So, close to the 50 hard line.
Warren: Superforecasters don’t like to stay on the 50/50 line. They want to get to the right direction of a forecast question as quickly as possible. So, half of their forecasts are between 15 and 85, which means the other half are below 15 and above 85. So, they’re showing great conviction in their forecasts while retaining their calibration. This is the important part. The forecasting activity that they’re making in the tail distribution, they’re retaining their calibration.
Warren: So, what does that mean? What that means is by getting there earlier, they’re getting an accurate forecast well and advanced of the rest of the crowd. What the research showed was that the superforecasters will get to that accurate forecast of, say, 90% on some of them 300 days before the rest of the crowd. So, it’s that time advantage. That’s the real payoff. That’s what shows up in having better resolution while retaining calibration. It’s what you get from doing a lot of forecasting and getting a lot of feedback, and learning a lot from your own accuracy and how to improve it. Does that help?
Corey: Absolutely.
Steve: The part of my question that we didn’t get to is to what extent the government has actually incorporated the fruit of this project that they sponsor?
Warren: Sure. Well, that’s a good question. I wish I knew the answer myself. We do know that these are ideas, and tools, and techniques that work. We do know that some parts of the US government find value in it and are incorporating these lessons and these tools. They don’t really tell us where and by how much. That’s something we don’t know about. We do know that they’re there.
Warren: We do know that other areas of the US government, as well as governments overseas are finding value in these approaches and putting them into their own decision making systems, making a part of the training for their staff at the government level and the national level, at the state and local level. We also know this is a case overseas, especially the United Kingdom. They’ve been quite ahead on these sorts of things, and other governments, too, Finland, the UAE, and others, too. So, it’s been really exciting from that point of view.
Warren: To answer your question, how much are the US intelligence agencies using the tools that they’re funding helped to define and refine, we don’t know.
Steve: So, one of the reasons I asked is that I have an old friend named Robin Hanson. Probably you’re familiar with that name. So, I’ve known him about 20 years now. When I first met him, he was actually working on the mathematics of prediction markets. So, he has, I think, some well-known papers on this on exactly what the right way to set up a market such that prices properly reflect collective probability, judgments, et cetera, et cetera. Incentives are the right way to surface thoughts and insights and things like this.
Steve: So, he worked on this for a long time, but then when I saw him not that long ago, I would say within probably a few years ago, and I could be misremembering what he told me, but this is my recollection is that he was somewhat dispirited about this because he said, “Well, in the various experiments that I’ve participated in,” and I don’t know how much overlap there is between what he did and what the Tetlock group was doing. Maybe it’s the same thing or maybe it’s different stuff, but he definitely had done some stuff for some corporate entities, as well as government.
Steve: He said that he ended up in a very cynical position where he just said, “Yeah. These things actually work. These mechanisms actually work for getting better predictions, but the leadership, the powers that be don’t have the proper incentives to adopt them. Hence, they generally are not adopted even though they work.” So, that was my last data point on this question from him. So, that’s why I was asking you if maybe you had an alternative view on it.
Warren: Yeah. Yeah. You’re right. There’s a lot of individuals and organizations involved on the research side of these things, and we’re all, in a certain sense, fellow travelers there. Just as far as prediction markets versus teams go, prediction markets are a great way to aggregate the wisdom of the crowd. It’s just that in many cases, two base forecasting is even better, but it really depends on what you’re trying to do and the resources you wanted to ploy to be able to do it. If it’s a short-term horizon, prediction markets are great.
Steve: Yeah. I should have clarified that. My conversation with him was not about the difference between whether predictions markets are better or some team-based forecasting or teams competing against teams, but just whether superior mechanisms for generating better predictions, which involve groups of people are being adopted in places where it can really help. His take was the cynical take, which is that it’s been shown to work, but for institutional reasons or incentives for powerful people. They’re just not adopted very widely. He’d given up that area of research.
Steve: One comment I want to make is that this COVID thing is an example of the hugest possible disaster because pretty obvious, what was happening in East Asia and then also even in Italy, meanwhile, the US government including its intelligence services, which presumably have a biowarfare detection function, right? They’re supposed to be able to detect biowarfare. How could they not detect a pandemic?
Steve: So, it seems like it doesn’t … I don’t see any evidence for good, maybe in South Korea or Taiwan or places like this, but in the US and the UK I don’t see any evidence for good information processing of this stuff for high level decisions. I’m curious what you think about it.
Warren: I have a different perspective, I think. I am deeply cynical without a doubt, but I see promise, and I recognize that this is new, which makes it difficult to persuade people that it’s worthwhile. It can be complex and complicated, which creates another hurdle. It also can be hard to connect the decisions, right? So, why should I pay attention to this? How is it going to make me make better decisions?
Warren: Then there’s also what I thought of as the Broadway problem, and that is if you’re so good, why aren’t you on Broadway? The version of that here is, “Well, if this is so good, why isn’t everybody already doing it?” Those are multiple veto points for people to say, “Let’s not do this.” It makes really tough to get something new potentially complicated, potentially threatening to status quo hierarchies, although that may not be the case, and also to request that resources be diverted from something else to something new, but it’s happening.
Warren: In the very largest organizations, it’s going to be tougher without a doubt, and we see that, I think, in some of the lack of uptake that Hanson was saying, but it is being taken up, and I don’t want to speak for other governments. They’ll speak for themselves, for sure, but I think as the months and quarters unfold, we’re going to see recognition of the value broaden, and I think we’ll also see that occur more in the private sector because there’s been a lot of adoption there, especially in finance, but also in energy, also in pharma, wherever there’s a lot of uncertainty that can be easily quantified to make a better decision that that it’s.
Warren: You’re right that COVID has become a case study, a real world case study where existing methodologies of coming up with forecasts can be improved upon with these other new tools. That recognition is really accelerated in the last, certainly the last eight weeks or so and continue to see that now.
Corey: So, Warren, I want to hop in to your COVID work, but Steve, probably in response to your question, I think it’s pretty well-known that the US intelligence agency was quite aware of the power of these techniques earlier on. I think this is an issue discussed in superforecasting, but there was a CIA study done where CIA agents were answering the same questions that were accessible to the public on Good Judgment Open, and I believe it was Michael Gerson at the Washington Post who leaked the result of the CIA study, correct me if I’m wrong, Warren, but I think the finding was that superforecasters then defined as the top 2% of people on Good Judgment Open outperform CIA agents by 30% accuracy even though they had no access to the vast trove of class by materials and all this internal information the CIA agents had.
Corey: So, they knew by, I think, by around 2014 that this was an extremely useful device. So, do you view this unlike many other federal agency that it does fund very risky projects? So, they were aware, but it’s an interesting question, Steve, is to whether they did anything with this knowledge knowing that this approach was better than their in-house analysts.
Steve: Right. I mean, just to be clear, I’m just relating and, again, I could be getting it wrong, so apologies to Robin if I’m getting wrong what he told, but I’m just relaying his observation, which I think is consistent with what both of you guys are saying, which is that this, if I view this as a technology, this thing actually works, and it’s been demonstrated to work. The question is just adoption by the most powerful people who really make the decision seems to be less than what one would hope for. That’s all I’m saying.
Steve: I’m on the side of promoting this kind of stuff as being good. Everyone should work on their own calibration and estimates of their accuracy and precision, whatever it is, but the question is whether … We can get into this in more detail. If you maybe are not that clever or not very familiar with the little bit of math, you just don’t feel comfortable with using this as a way to supersede your own gut instinct, which got you so far in life, right?
Steve: I can imagine lots of reasons why the really powerful people want to retain their freedom to make their own decisions even in the face of a better forecasting technology, but I hope that-
Corey: Do you think the desire-
Steve: Go ahead.
Corey: Do you think the desire to make money will be successful and override this kind of … or does it matter?
Steve: This is where Robin’s issue of incentives comes in. So, if you’re writing a hedge fund and you’re measured very well on returns, yeah, you do have a very strong incentive to get things right. If you’re running a country, it’s not so clear or running an intelligence service or whatever it is. So, I mean, if they’re not measured well, maybe they don’t have the right incentive to actually implement something like this.
Warren: Well, it is something that is diffusing, the approach and others that are related to it. So, to think probabilistically and to rely on the wisdom of the crowd to better quantify uncertainty, it’s a space that has been sparsely populated. So, on the one hand, you’ve got the hard quantitative types, the big data, purely data driven, right? So, if it’s a mind model, it needs to have numbers in it. Then on the other side, you’ve got people who are much more subjective about the way they think about things.
Warren: In the real world, there isn’t always the data that you want to have to be able to make decisions that are highly subjective. So, people will operate. You used the right word, too, on a hunch. They’ll have a hunch. This is something that I think will work, and they might have experience in it, whatever, but it will be on a hunch.
Warren: What we’re doing here is saying, “Let’s quantify that hunch. Let’s connect that qualitative subjective understanding about the way the world works, and make it measurable, testable, comparable, and do that by using probabilities. What is the probability of this subjective event actually occurring? That’s a way to connect the two. It’s a space that is proven to be very fertile.
Warren: Good Judgment has done a lot of great work there led by Phil Tetlock and his colleagues. Others are there, too. There’s a lot more to be discovered, a lot more to be done, and you’re correct that the large organizations, they’re not usually early adopters. They’re usually late adopters. There are plenty of early adopters, though, where they’re applying these sorts of approaches and finding value in them.
Warren: In finance, for instance, here’s a great example. A merger and acquisition is announced. What is the probability that it will go through? That’s a very important forecast to get right. If you can get even a few percentage points edge on what’s getting priced into the market by your competitors, over time, that is worth a lot.
Warren: It’s the same true for government policy decisions. For instance, if you’re trying to project how many hospital beds you’re going to need, wouldn’t it have been good in January if you have been watching what the superforecasters were saying who said, “In January, when few people are even thinking about it that the case load is going to go into the six digits by March,” and that’s what occurred. So, if you’re thinking of allocations of hospital beds, that can be very useful and, literally, life and death decisions.
Warren: Same sorts of things for when a vaccine is going to be developed or when treatments are going to be widely available. Those sorts of things are very consequential for government decisions. While some of the larger organizations within government may not be adopting quickly, others are, and we’re going to be hearing more and more from them if and as they find utility in these approaches. That’s the way diffusion often works is it’s the early adopters are not the largest organizations.
Warren: Although, I will say that larger organizations often have smaller units within them specifically tasked with identifying and diffusing these sorts of tools. Even in government, there are organizations, units that do that, and superforecasting tools are some of the things that they are looking at as we speak.
Warren: When we’ll hear about them and whether they’ll be adopted, that itself is a forecast, but I’m pretty sure we’ll hear about them long after we’ve heard about other used cases.
Corey: I think that’s a great topic. I don’t want to go on for too far back. I have a little personal anecdote that confirms the lack of incentive for adopting accurate forecasts or this methodology. Back when I was in consulting, I noticed that our firm would make lots of … They’re sort of forecasts, but pretty close to flat out predictions as to how the client would do if they adopted our particular approach. They’re rarely probabilities attached to them. They’re extremely confident.
Corey: I remember asking around whether we ever checked to see whether our predictions came true. The response I got was, “Well, that’s not possible because our predictions depend upon the client implementations, and we have no control of implementation. So, you can’t blame us if the prediction doesn’t come true.”
Corey: Be that as may, I think you can question that, but it was clear there’s very little incentive at the level the organization to assess accuracy because the people who are hiring the organization didn’t want to asses accuracy because they didn’t want to basically raise to their superiors that something they had paid a lot of money for didn’t work. We had very little interest in letting people know that our approach might not have worked.
Corey: So, I guess in the case to where there’s no feedback loop, that’s a case where you essentially don’t have any pressure on accuracy. So, it was a really striking experience to me when I compared that conversation to the conversation that, Warren, you and I have had over various periods of time about the power of forecasting because it’s a situation where it’s a very, very profitable company, but the people on top are just not held accountable on that particular line. They held accountable for having happy clients, but not particularly for whether the claims they make to those clients got to be true or false.
Warren: Yeah. I mean, in the case of McKinsey, it may be extremely damaging to them for clients to know what their actual accuracy is because they may be priced on brand and brand perception may be far in excess of what they can actually achieve in terms of accuracy, right? So, they may have a very strong incentive never to be marked and marketed in that way. Where are all the customers’ yachts?
Corey: Yeah. It’s interesting. I think McKinsey, at the time I was there, was charging 30% more than the nearest competitor. It’s hard to imagine they had 30% greater accuracy.
Warren: Yeah. Exactly, but better just to allow the illusion to persist that, “Hey, we in BCG are awesome.”
Corey: The comments we’re actually even funnier that that. BCG was described as commercial. Those people are just really commercial. We’re not like that at all.
Warren: Yeah. There’s also some division of labor that can usefully be part of how all of this fits together. Well, first, for the decision makers within larger organizations, they have different skills, right? That’s what helped them get to where they are. Being a skilled forecaster is not something necessarily you need to be a good leader, right? It means often a good leader is someone who can motivate people to get stuff done, whatever that thing is. That’s a different skillset, and one where the thinking probabilistically about, “Well, maybe this, maybe that, maybe the other thing, and I’ll wait. Let me think I through,” that gets you to an accurate forecast, but when it comes time to make a decision, things change and you need to get people to do things.
Warren: So, the idea of having a high quality probability estimate is that the decision you make is going to be the best possible one in the decision set in front of you. What you then do with it is a different thing. I think it’s the same thing with the management consultancies.
Warren: Now, they do other things. One of the things some of them are very good at is thinking about scenarios, right? What are the different ways the world might be from now? When we think about COVID, right? What might the world look like a year or two from now? What are the different worlds we might find ourselves in?
Warren: What they’re not so good at doing and don’t claim to do necessarily is which world are we actually headed into? Now, you might find an expert who will on that hunch go, “Well, I think it’s this world that we’re going in to,” boom, boom, boom, boom. What we’re doing here at Good Judgment is, “Okay. Let’s take those scenarios about what possible world we’re going to go into, and let’s break them down into testable propositions. Once we get those testable propositions, we can then go and get forecasts from the best in the business, and that will let us know which world we’re actually heading into.”
Warren: Now, if you’re a decision maker and you’re in a position to alter the world we might be going in to, then you can pose questions about the effectiveness of your interventions. So, if I’m, say, in the military and I want to know what the scenarios might be about confrontation with China and Asia, we’ll have a bunch of scenarios.
Warren: Then we can ask those same forecasters if I put this carrier group in the South China Sea, will that improve the probability of the objective I want to see or reduce the probability of that occurring, whatever that might be? So, separating decisions from scenarios from the forecasts can be a useful way to think about how the division of labor can be very effective because when you think about the decisions, right, the decisions can be very consequential in the tails, right?
Warren: So, a 2% probability of a coronavirus outbreak a year from now, if you’re decision maker, that has a very different meaning than if you’re a forecaster. If you’re a forecaster, you’re just going to wait and see what happens and get your feedback. If you’re a decision maker, that 2% might be too high or not. So, the consequences of what the probabilities are telling you can usefully be kept separate from the panel, from the group of forecasters who are providing the probability estimates, and have a division of labor.
Corey: So, I think this is one of the really interesting findings of the Good Judgment Project is that this kind of different perspective, this cognitive diversity leads to better group forecast. That was an interesting finding because it was actually contrary to what was being published before primarily about bias, which is when you have people who think the same way and putting them in groups has negative consequences. So, I think that was well-established, but it wasn’t clear what happened when you had people of different points of view.
Corey: Do we know whether this works in general or just when people have the mental outlook to be a good forecaster? Is there something special about the people going to the teams you think aside from cognitive diversity? Can we take any group of people who’s cognitively diverse? Do you think you’re fine and suggest that they will make better group decisions when put together or is there some special sauce that happens when you put people who are high performing forecasters together?
Warren: Well, setting aside decisions, they will, I think, reliably come up with more accurate forecasts by having cognitive diversity. If I don’t know anything other about a group, but that one is cognitively diverse and the other is not, no question which one I’m going to pay more attention to. That’s at the group level.
Warren: At the individual level, there’s some characteristics that are consistent with good forecasters. Being good at pattern recognition is a very important one. Another is being what they call actively open-minded, and this is the idea that your beliefs about the world or the things that you’re always testing not protecting, right? So, we often see people on TV, they’re protecting their beliefs, “Oh, that doesn’t matter. Oh, this doesn’t matter.”
Warren: They’re no very good forecasters, and you can screen for that, too, and see people who are going to be better. Then put them on a platform, start seeing how they forecast, and start scoring them. When you do that and you can observe that there’s cognitive diversity at work, the results of the forecast that they generate will be superior over time.
Steve: Could we be a little bit precise about what we mean by cognitive diversity? So, for example, is it beneficial to have high IQ and low IQ people on the team? Is that a positive form of cognitive diversity or do you mean diversity in ways of thinking or knowledge backgrounds, perspectives? So, what exactly is meant by the diversity?
Warren: Oh, much more of the latter, Steve, very much so. Different mental models about how the world works has a lot of value. That can show up with different backgrounds, different education, different life experiences, different ways that we engage and think about the world is what you really want to see. So, we’re not clones of each other. So, if we all went to the same schools, have the same jobs, have the same experiences, have the same mental models, and you pose a question to us as a team of forecasters, you’re not getting a diversity of the crowd. You’re just getting one person cloned multiple times.
Warren: What you want to have is a lot of different people with those different perspectives, able to contribute their views on a level playing field to really contribute to filling out what the group is understanding.
Warren: Now, there’s a great new research that, there’s always more research coming, and one of the really interesting ideas that’s coming out of this is the concept of noise and how it relates to bias as well. It all fits with what we’re talking about here. So, in the original research, they were focusing on, “Well, what’s really going on here?”
Warren: Well, so, in accurate forecast, you can think of as one that improves the quality of information about the event that we’re trying to forecast. We want to properly identify and wait that information. We also want to be aware of information that is not useful, that doesn’t contribute to the accuracy of the forecast.
Warren: A lot of the attention on that side, the error is the kind of error you get from bias. For instance, we tend to be overconfident. That’s a bias. That’s predictable systematic error. Over time, so it’s very difficult to do things about bias, but over time, because it’s predictable, you can identify it, “Oh, you’re overconfident. I’m going to correct for that in the algorithms.”
Warren: Now, the other kind of error that I think is really interesting is the nonsystematic error. It’s information that does not correlate with the outcome at all. It’s noise. This is research that Daniel Kahneman is doing. Phil Tetlock has done some, too. The whole idea is that noise reduction can sometimes be difficult to identify, but once you do, they’re very good techniques to reduce it.
Warren: So, at a group level, how does wisdom of the crowd works? What’s really going on here? Well, one thing that’s going on is that all the errors that we all have in a big group of people like Who Wants to be a Millionaire, they’re canceling each other out because the error is normally distributed. You go to the median. There’s the wisdom of the crowd. That’s great. So, it’s a very crude but effective noise reduction tool with the crowd is just to take the median.
Warren: Now, what was going on with Good Judgment is now, let’s provide individuals and teams of individuals tool to reduce the noise at that level, to squeeze out more of the noise, boost the quality of information that they are sharing together. So, that’s something that really works when you have cognitive diversity to identify the pieces of information that matter and zero in on them, and the same time built throughout the information that is not so useful, the noise.
Corey: Warren, can I stop you? Because it’s getting a little abstract perhaps for our listeners. My guess is you actually have concrete experience with a couple of teams, of actual individual people working to try to reduce noise. So, could you possibly just pick in your head a team that you’ve worked with? Describe to us who is on that team. What kind of backgrounds they have? Try to give for our listeners an illustration of what might be biases, what might be noise, just to give people a concrete sense of how the concepts of noise and biases will apply to group judgment. Is that possible to do? Just think of a team you’ve worked with. Give a sense of who’s on there, what sense they’re diverse, and try to give a concrete sense of what these terms mean on the ground.
Warren: Well, how about a specific example of what I think to be a good example of noise? Because we see it all the time. That is, without picking on anyone in particular, there are people who have a view about the world. So, I’ll give an example of Nouriel Roubini, right? His nickname is
Steve: Dr. Doom.
Warren: Dr. Doom, right.
Warren: He is always saying the world is on the edge of an abyss or sliding into the abyss. He’s telling us the same forecast over and over and over, and I’ll go a little farther is that back in February, he was saying that the world is headed for a global downturn because of what he saw to be a spike in oil prices that was on the way. Okay.
Warren: Now, a month later, oil prices collapsed, which he then offered as support for why the world is heading into a global recession. So, oil price spiked, oil price collapsed. Either way, it’s the same forecast.
Warren: Now, when you go back through time, how often do we have a global collapse? Oh, they happen, but not every year. Maybe it’s one every 20 years or thereabouts. So, empirically, to the degree that those kinds of forecasts are get made and they do not correlate with what subsequently happens, that’s noise, and not to pick on just one economist. There are many who do that.
Warren: So, I as a forecaster and the other forecasters on my team, when they see that kind of information, those kinds of headlines will very rapidly discount it and move it to the side. There may be something useful in there that’s buried that we want to pay attention to, but that’s the stuff we want to filter out. We want to filter in the subtlety significant information, and make more use of that, whether the early detection signals we can use.
Corey: How do you identify those?
Warren: Well, one of the superforecasters put it very nicely recently, and I’m not going to get it exactly right, but we’re talking earlier about the importance of being skilled at pattern recognition as being an indicator of a good forecaster, but it’s not so much just identifying the pattern. It’s also detecting when the pattern itself is changing.
Warren: A wonderful example of that was during the last US election when some of the superforecasters based in the DC area during the summer went on a car trip to the Midwest, to Upstate New York. Usually, in an election year, they’d see a lot different sign in people’s yards. That year, there were signs far and away for just one of the parties and not the other. So, that was as subtle change that some of those superforecasters recognized as being significant and they adjusted their forecast as a result.
Warren: You think it through, right? What they’re seeing in that observation is that the usual pattern of different parties having representation out in rural areas have changed, and they shifted their forecasts as a result. That sort of thing I find is a great example of filtering out the noisy stuff and identifying and appropriately waiting the more subtle significant information that’s out there.
Corey: Now, Warren, one of the, I think, new focuses of Good Judgment is on trying to merge since the machine techniques and computation with human judgment. Many people are saying that, “Well, look. Eventually, computers are going to be just unbelievably good at making forecasts of all sorts, and large heap of people out of business.”
Corey: Now, I think that’s a extremely unlikely. My view is that they’re probably going to be working together for the foreseeable future and probably forever, but I do recall a conversation that we had a couple of years ago where you said you were beginning to investigate performance of essentially machine prediction combined with human prediction. Do I have that recollection right? If so, where is that research now?
Warren: You’re right, Corey. The billion has end. It’s humans and machines is really the way to go. In a certain sense, that’s always been true. Even the Good Judgment research relies a lot on technology, and the superforecasters themselves use a lot of different models to assist their own forecasting. The research that you are talking about is a little different.
Warren: What that was trying to do is just like the Good Judgment Project was a part of research to see what sorts of tools and techniques can improve on the wisdom of the crowd, this asks the same question about what combinations of humans and machines on different kinds of topic areas can lead to better results than you would get from the wisdom of the crowd.
Warren: The results of that research to my awareness have been inconclusive and the upshot I think is the future for humans in forecasting is not in doubt for these sorts of subjective events.
Warren: Now, machines absolutely have an edge where you have a lot of data and you need to do rapid computations. That is the division of labor that seems to be taking shape. That’s not in the division of labor either. It’s just moving, right?
Warren: So, computer themselves are machines that replaced humans. The original computers were people with pencil and paper and an adding machine. Machines came along and we’re able to do that work more efficiently. What that means is for the humans is that they have more time to do other things. They don’t have to do the wrong number crunching. They can instead think about the consequences of making decisions, the element of judgment, the combination of the subject and the quantitative we were talking about earlier. That’s the zone of judgment.
Warren: By having machine learning and big data and artificial intelligence really assist and do a lot of the heavy lifting, that leaves more time for us to focus in on what really matters to make better decisions. That’s a great thing. I think that’s a great trade.
Warren: As time goes on, no doubt, machines will be doing more. There may even be more and more areas of decisions themselves where we go, “You know what? The machines have got it. Let’s rely on that.” Radiology might be one where they’re not to do this in future machines will be doing a great job at detecting those sorts of issues that can outperform humans. We’ll be seeing other areas, too. For the moment, when it comes to the more subjective sorts of decisions that we all need to make, the machines are not there yet.
Corey: So, in your work, where do you use computer models, and what do you use them for?
Warren: So, it’s three levels, really, I think. Level one is at the level of an individual forecaster, right? So, one thing I do myself when I see a forecasting question is I like to make a spreadsheet and get all the data I can find and drop it in, and look for base rates, right? I’ll do very good models that way. Other forecasters will get far more sophisticated and create base nets even, and take other sophisticated approaches like that.
Warren: So, at an individual level, we’ll be using machines and computers. Then when we aggregate the data, the individual forecasts that come through the teams, we have a group forecast. This is the next level, which the machines can really be helpful, and that’s to have an algorithm that further squeezes out the noise and gets much more higher quality forecast. In a Good Judgment research project phase, that step alone could contribute materially, 10-20 percentage points to the accuracy of a forecast, and you’re putting it all together.
Warren: So, individual and then the group forecast, and then what do you do with that forecast is the next level. So, the forecast from the humans and the machines through this process can then be fed into a larger system that can include other sources of information from big data, from AI and machine learning, and have a much more robust ongoing system that doesn’t rely just on big data or just on subjectivity, but is a blend of everything to have a much more fully formed model about how things will work for consequential decisions.
Steve: I have a general and meta level question. So, the firm you run, is it basically the business model is like a consulting service, so you’re selling forecasting capabilities to clients and they pay you on a consulting basis? How does that actually work?
Warren: There’s a little bit of that, but we’re not really in the consulting business. The main thing we do is we have the superforecasters. We have the best. Clients will pose their questions to the superforecasters and we’ll provide probability estimates along with comments about those forecasts. We’ll do that for individual questions. We also do it on a subscription basis.
Warren: So, we’ll have a set of questions, say, on global risks as we do, and people are able to subscribe to that. So, that’s our main central business line is that service, which is quite scalable, and we work with organizations and government, defense intelligence, finance, energy, and others.
Steve: Can you say how many superforecasters you actually employ to make these forecasts?
Warren: They are independent contractors and will work with us on task orders. At the moment, there are about 150. Each year, we go to Good Judgment Open and invite the best of the best there to come and join the professionals. So, that’s one thing we do.
Warren: Sometimes organizations want to build these capabilities internally, too. So, we will provide training to show what works and what doesn’t, what the best practices are to come up with forecasts, as well as to pose the questions that will matter inside organizations, where they can get the wisdom of their own crowd.
Steve: Is there any evidence that your customers are in a sophisticated way evaluating your performance?
Warren: I’m not quite sure I understood the question.
Steve: Well, so I’m buying a product from you. I’m buying some forecaster probability distributions from you. If I’m disciplined, I would, after a few years of having a subscription to your service, have my own view of just how good you are, how well do you outperform some other entity that’s selling me predictions or my internal capabilities. What’s the level of sophistication on the client side evaluation of the product that you detect? In other words, they read a book called Superforecasters and they decided, “Hey, these guys are awesome. Let’s just pay them to do some stuff for us,” or are they themselves sophisticated consumers of what you’re providing?
Warren: Boy, Steve, I wish it were that easy that they just read the book and say, “Sign me up.” It isn’t. They’re very sophisticated. They want to see value, the value of proposition in what this can do. The specific way that you’re asking about is, “Well, how do I know that this is accurate information?” You’re right. The best way to find that out is to be able to compare it to something else, some other external source of forecasting, for instance. That’s been done, and it’s been done by clients. It’s also been done by us. As far as we’re aware, the superforecasters on any reasonably rich mix of questions, so the data is meaningful, have come out ahead.
Warren: There’s been more informal experiments, too, where some client have posed questions to the superforecasters and done their own internal forecasting on their own. Didn’t tell us about it until later, and to the degree that they have been sharing those results. It’s showing the superforecasters to be well ahead.
Warren: It’s not terribly surprising in a certain sense either because a lot of the way that forecasting gets done is without the benefit of these best practices using crowds, doing updates, pulling information. These sorts of basic things have not yet diffused as widely as they could, and I hope that that changes in the months and quarters ahead.
Steve: Yeah. I’m not surprise that you guys outperform other options. What is interesting to me is the degree of sophistication of the consumers, the clients in terms of how well they try to measure that or benchmark that.
Warren: For them to keep coming back, they’re going to want to have evidence to support it, and that’s what we certainly have been seeing.
Steve: To give you another classic example, which I’ve been interested in for a really long time is estimating Alpha of traders. So, obviously, huge compensation numbers are tied to this question. You can talk to experts either academic researchers or people in the business who have diametrically opposed views. One set will say, “Oh, yeah. We can measure Alpha.” There’s enough record in somebody’s trades over some five, 10-year period as a portfolio manager to really get a sense of whether they have Alpha or not.
Steve: Then there are other people who are very pessimistic and say, “No, we never know because this guy could have just been lucky because his personal biases that arise for idiosyncratic reasons from his life just happen to align well with market conditions for his five-year run.”
Steve: So, I think there’s still some dispute as to whether that very well-defined quantity can be reliably estimated or even if it’s a stable thing to estimate for individuals. That’s a very, very clear problem that everybody has. Everybody that runs money has this problem. Yet, I don’t think there’s a universal agreement on what the actual situation is.
Warren: I’m glad you brought that up. It goes to how do you evaluate performance. Some of the other interesting research that’s been going on and what Phil Tetlock has called Alpha Brier. So, what is that about? So, Alpha, when you boil it down, it’s your P&L, right? So, it’s an outcome-based way of evaluating performance.
Steve: Slight correction. So, it’s your P&L, but normalized to the amount of risk you took on. So, if your portfolio is very stable and you didn’t take on a lot of risk, even a smaller level of rate of return would be acceptable, right? So, it’s return normalized to the amount of volatility or risk that you took on.
Warren: However you define it, though, right? It is an outcome-based evaluation of performance. A Brier score is a process-based evaluation, right? So, it tells you, “Were you right for the right reasons?” You can combine the two. You can think of a grid where you have Alpha on the Y axis and the Brier score on the X axis, and what you want to see is high Alpha and low Brier score.
Warren: This is information that exists. Listen, portfolio managers are making forecasts all the time that could be tracked and converted into a Brier’s score. We also see what the performance is and that can be measured as Alpha, and populate a grid to see where analysts and portfolio managers collectively land.
Warren: Ideally, you’d like to see them in that upper left quadrant, but perhaps, they’re in the upper right quadrant. That’s the zone of better lucky than smart, right? That’s a time-tested adage. “Oh, well, I’m right even though my reasons were completely misplaced.”
Warren: There’s a great example on the last election on that, too. I know a lot of people, I’m sure you did, too, who had a view about the election outcome that the Democrat would win, and that that would be good for markets. So, they went long. Sure enough, in the New Year, stock market soar, and they looked brilliant, but they were better lucky than smart. They were right for the wrong reasons.
Warren: If you take an Alpha-Brier approach, you will get the feedback that you were just lucky and not smart in that instance, and be able to act to improve in the future. So, that’s a really interesting area. Some firms are actively trying to combine ways to evaluate performance in that way. I think it’s a very promising area to be.
Steve: Yeah. It’s very interesting because some people could have … If you see high Alpha and low Brier, then I think, first hypothesis will be this guy’s lucky, but it could also be that the articulated events that are in the Brier score that people think are driving the market actually aren’t, and so you could be wrong on all the Brier stuff, but you have some gut feel for just what the market is going to do, and you’re able to trade on that and generate the Alpha even though your Brier score is low. So, I don’t know if that really could be how things are, but I wouldn’t completely discount it.
Steve: On this issue of hunches, I don’t know if you’re familiar with the work of this guy Gigerenzer. So, he’s a, I think, German psychologist, who is a little bit of a foil for Kahneman and Tversky. I don’t know if you’re familiar with his work, but he’s also fairly prominent in some of these decision theoretic places. He actually claims to have data that suggested in a lot of settings. We have to define this very carefully, and I won’t do a good job of it, but expert intuition, where people make up their minds very quickly but they have a lot of experience in the area does actually outperform this rational Bayesian thinking, which incorporates lots of details and things like this.
Steve: Just recently, I think he’s been making claims that he has lots of data that suggest that informed hunches. Actually, of course, it’s subsuming lots of information processing and deep intuition that your brain is doing, you just don’t have conscious access to it. He claims there’s a lot of evidence that that’s actually better than the more rational algorithm-like things that some of us might prefer.
Warren: Well, and I’m not as familiar with his work, but they’re maybe more overlap. I am more familiar with Gary Klein and his work. It’s the idea of acting on intuition. I’m going to be saying it right. I’m sure I’m saying it in a different way, but the idea is that you accumulate a lot of experience, and through experience, you begin to recognize situations almost intuitively and act on those recognitions that you may not even be able to articulate to yourself. It’s just something that’s become built in that you have learned, that you have acquired through experience.
Steve: Yes. I think we’re about talking about the same thing.
Warren: In that sense, superforecasting is the same kind of thing, being able to translate hunches into numerical probability estimates. It’s a skill of the same sort. For the people that do it a lot, it becomes second nature. Oftentimes, they won’t even be able to articulate why it’s going to be a hunch that they quantify with a number because that’s now second nature. So, in that sense, maybe there’s a fair amount of overlap that these research results would be showing. Love data. Love to see that data, too.
Corey: Yeah. This has been a debate. Gigerenzer’s been having this with Kahneman since the ’90s. I think some people do think that it may … This is very hard to separate them empirically, right? Any scientist or any expert in an area has internalized the phenomenal amount of information and computational principles. The question is, is this self-explicit or not? That just may be the difference. I think the extreme version of Gigerenzer is that the computation is not happening, that at some level something else is happening aside from using base or a physicist using very sophisticated intuition that effectively internalizes a number of physical laws.
Corey: The extreme version is there’s something else going on. I think the natural version is that over time, these things have simply become part of your basal computation. So, you make judgments about explicitly pulling out a pencil and paper. I think that’s right, right? Obviously, it’s data that you’re talking about, Steve, but if that’s right, it’s very hard to imagine whether there’s an exception, there’s an empirical difference except whether it’s conscious or not.
Steve: I think without getting too far into his stuff, I think some of the recent stuff I think I listened to a couple of talks, recent talks of his, maybe the distinction was judgments that someone can make very quickly, and so quickly that they clearly didn’t go through any articulatable analysis or algorithm.
Steve: Well, it could be an algorithm, but it wasn’t something where they chained together a bunch of reasoning. They just said, “Okay. That’s not going to happen.” Yet, in some circumstances, he claims to have data where that just outperforms, allowing a guy to have 20 minutes and access to Google and do a bunch of other things before they come to, and they use a pencil and paper, and a spreadsheet, and he claims to have … I think, I could be getting this wrong, but I think he claims to have examples where the former beats the latter for certain tasks. So, I found that quite interesting.
Corey: So, they get it wrong if you give them time to think.
Steve: Yeah. Exactly it. That’s what he’s claiming. He has examples like that.
Corey: Interesting. So, Warren, we’re about out of time, and we, in fact, didn’t get to the one topic we thought we’d talk about. It’s been a great conversation, anyway. Any last few comments about your new project on COVID because I think this is something our listeners might be very interested in. So, tell us a little bit about your current crowdsourcing project, where you’re really focusing on experts to answer specific questions about COVID.
Warren: Yeah. So, we’ve got our superforecaster dashboard with some high level questions, but we’ve also set up a platform that … Good Judgment has been open, too, where the public at large can engage on a lot of questions related to COVID. We thought it would be helpful to provide a platform for the experts themselves that’s closed. You need to be a professional and qualify as such. Then you can go and contribute your wisdom to that crowd on the more technical questions.
Warren: I’m hopeful that it will be helpful for the experts because many of them, and we’re talking about this earlier, have been forecasting with one hand behind their back not doing best practices. So, often we see out in the media, too, a collection of forecasts from experts that just don’t look good because they are made at a moment in time. It’s a simple aggregation, a median of the experts involved, and they don’t have an opportunity to exchange their own information and make an update based on that other information, those very simple basic best practices that can be really helpful.
Warren: So, that’s what we’ve set up a platform for experts to go and forecast on some of these questions, make their initial forecast, benefit from the other perspectives they’ll see there, and then make an update because, really, when we’re making forecasts, most of the work, the mental work goes on into that first initial forecast, but the payoff and accuracy is comes next. So, it’s maybe 80% of the labor coming up with that initial forecast, and then 20% more laborer are reading other comments and just rethinking your own assumptions is a big payoff in accuracy. So, 80% of the accuracy can come from 20% of the work.
Corey: So, who are you inviting onto this new platform?
Warren: Anyone who qualifies as an expert broadly defined. They’re self-qualifying. So, epidemiologists, practitioners, medical professionals, policymakers who are deeply involved in these issues, they’re all invited to come and benefit from that platform and, hopefully, it will help them in their own forecasting, as well as provide them with the wisdom of that crowd when they need to make the decisions in front of them.
Corey: Well, Steve, I think we’re about at the end of our time with Warren. Do you have any last questions?
Steve: No. Warren, it’s been really great talking to you. It’s been a privilege, actually. I have been, as you can tell, deeply curious about the area that you work in. I raised some cynical observations, but I fundamentally think it’s a useful technology for human decision making and making better predictions. So, I wish you guys all the best.
Warren: Well, thank you. It’s been a great conversation. I love tough questions, and I hope I gave you some reasonable answers.
Corey: It was great catching up, Warren. Thanks for your time.
Steve: Take care.
Warren: Thanks, Corey. Thanks, Steve. Bye-bye.
Corey: Okay.
Steve: Bye, everyone.
Creators and Guests
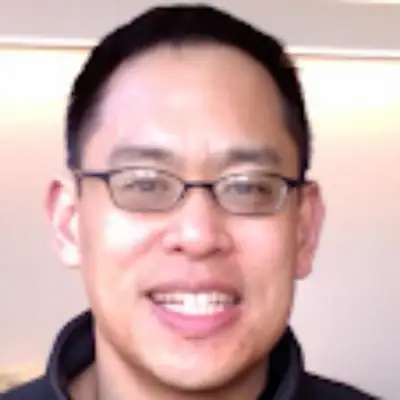