Shai Carmi: Polygenic risk scores & embryo screening — #5
Steve Hsu: Welcome to Manifold. I'm very excited today to have Professor Shai Carmi of Hebrew University in Jerusalem. Shai is a distinguished and very well-known professor of statistical genetics and computational genetics.
He also is a Twitter explainer of other people's scientific papers. It’s not the way I first became aware of Shai, but one of the ways in which he impinged heavily on my consciousness. Because there's some set of people who are following the field really carefully. When they see an interesting paper, they actually put out a Twitter thread on it or tweet on it. My lab has benefited very much from Shai because actually, all the postdocs in my lab follow Shai's Twitter feed, and well, it's sad, but true. But science is now kind of being centered on Twitter these days.
Shai, welcome to Manifold.
Shai Carmi: Hello, welcome. Thank you.
Steve Hsu: So, I would like to start with your early life and education. I discovered, embarrassingly for me, just recently, that you also began your career as a physicist. And so you have undergraduate degrees in physics and computer science, and then your Ph.D. was in physics. And I think it looks like you started in some kind of statistical physics, or network theory, but then by the time you finished your Ph.D., you are already kind of getting into things like gene editing and things like this. Maybe you could just tell us a little bit about your intellectual journey.
Shai Carmi: Yeah, sure. So I studied at Bar-Ilan University. That's in central Israel. And as an undergrad, I studied computer science and physics and a little bit of math. By the time I was in third year as an undergrad, I started research in a computational group in the physics department there.
And I worked in statistical physics towards my master's degree and a Ph.D. And the subjects that I worked on were network theory, as you mentioned. We studied transport processes over networks. And in other words random walks or search or navigation over random networks. And sometimes, sometimes over real networks, like the internet.
And I did that for a few years. And then also studied diffusion equations. So-called anomalous diffusion theory.
And then by about 2007, 2008, I decided that I would like to try the life sciences. I would like to learn more biology and see if I can do research in that area. It really interested me. So I took some classes and I started talking to some people. I also worked in the wet lab for about a year. Doing experiments with RNA … genetics experiments. And then a year later I even joined a lab still at Bar-Ilan University in the life sciences in a lab that worked on RNA editing.
So that's a few years before you know, CRISPR and all that. We studied the changes that happened through the sequence of RNA after the transcription. So I did that for about another year. And then I moved to the United States for my postdoc. I did my postdoc at Columbia University in New York with Itsik Pe’er. The things that I studied there were population genetics and later medical genetics. And these are still the areas where I do research in my group at the Hebrew University. So I've been at Hebrew University since 2015. It's been about seven years now. And I'm at the faculty of medicine at the school of public health.
And I'm still working on population genetics. I spend about half my time in that field, developing a theory, methods, learning how to use genetic variation, to learn about the demographic history of populations, about mutations and recommendations, and about these processes. And specifically we're interested in the demographic history of Jewish and other Israeli populations. And also recently, in the context of ancient DNA.
And in the second half of the activities in my lab, we work in medical genetics, and we have a few collaborations studying specific diseases.
But we also have some projects on screening preconception carrier screening, and also pre-implantation genetic testing. And, I mean, maybe I can share the story of how I got to this you know, to work on, on subjects so diverse, you know, from Ashkenazi Jewish genetics to pre-implantation genetic testing. In other words, testing of embryos.
So in my postdoc, just about 10 years ago, we started a large sequencing project, in the Jewish population. So it was a consortium of about a dozen researchers from the New York area. And we sequenced nearly 130 whole genomes, which at the time was, was quite a lot. Still quite nice.
And I led the analysis of this data as a postdoc. We used this data to study the history of the population. For example, we studied a founding event in the history of Ashkenazi Jews. The population was very small until about 700 years ago, which led to a lot of disease mutations, for example.
And we also did some work in medical genetics with this data. And for example, I mean one of the applications of this data, one of the ways this data is used is for improving imputation. So if we have genetic data coming from microarrays, which is as far as, and only has about a half-a-million or so genetic markers, we can use whole-genome sequencing data to impute, to fill in all the missing variants.
But by finding similarities between the genome that we are trying to impute, that for which we have missing data similarity between that genome and one of the genomes that we sequenced. By the time I was about to return to Israel, someone approached me from the Shaare Zedek hospital in Jerusalem from the pre-implantation genetic testing lab there. And they were trying to improve the accuracy of methods for sequencing the genomes of embryos for specific disease mutations.
And because many of their patients have Ashkenazi Jewish ancestry, they thought that by using these polygenic sequences, they could improve the accuracy of genotyping of the embryos, which is difficult because it's basically a single-cell experiment. There is very little genetic material to work with.
I started working with this person, his name is David Zeevi. And then we published a little paper about these Ashkenazi reference journals, but we also started developing methods more generally for sequencing the genomes of embryos, using very low coverage sequencing of the embryos.
And we published a couple of papers on these methods, which have both experimental and computational innovations. And we're still working. We're still working together. And by the time I became more knowledgeable in how PGT works and what are the challenges in sequencing embryos and, you know,just the whole procedure of how PGT works, I also learned about all the developments in the genetics of complex traits and diseases. I thought that, you know, now that we have whole genomes available for the embryos, which actually surprised me, it's, you know, we could achieve very accurate coverage of those embryos for basically just a hundred dollars per embryo.
And then I thought if we have all this information from the embryo, what else can we do with this information? And then all those studies started to come out with polygenic scores for predicting traits and disease risk, and so on, which led me to start thinking about screening and IVF embryos for polygenic traits and diseases.
And since then, this is when I started this series of research projects on this subject.
Steve Hsu: Great. That's a great segue. I think we are going to discuss several of your papers if we have time. But it reminds me, we need to make a couple of disclaimers. So I'm a co-founder of two companies, Othram, which does forensic DNA analysis, and another called Genomic Prediction, which does preimplantation genetic screening of embryos.
And you are in no way endorsing those companies. You are only stating your scientific opinions based on your own research. And we should not ascribe any endorsement to you for the work of those companies. Similarly, you are a consultant for MyHeritageDNA, which is a, I guess you could call it a consumer DNA company, which I guess has focused both on ancestry, maybe, and also on health risks. And I guess we should just point that out for any conflict of interest reasons. Is that fair?
Shai Carmi: Yes. Thank you very much for pointing this out.
Steve Hsu: Great. Now I'm really interested in your paper. So you wrote a paper analyzing the gain or the utility gain from embryo selection — risk, I guess I should say, risk reduction — from embryo selection. And one of the phenotypes that you looked at was schizophrenia. I have a personal connection to this because in high school, one of my close friends, an Ashkenazi Jewish American, but we were growing up in the middle of Iowa. His father was a history professor. He was a brilliant kid and he went to Princeton to study, he was a double major in biology and English. But then he had an attack of schizophrenia in his early twenties. And he's never been honestly normal since. He's been an actual, you know, I would honestly say a kind of a burden to his family for his entire life because he never really recovered. And that was a very big shock to me because intellectually this guy was very strong, very ambitious, and he was just struck down by this disease. And so I've always had a little bit of an interest in schizophrenia. I don't know that much about it, but for this personal reason, I have an interest in it.
My understanding is it affects something like half a percent roughly. I mean it varies from population to population, but...
Shai Carmi: Even up to 1%.
Steve Hsu: Even 1% of the general population. So it's not a super rare condition, but it can be devastating, not just for the individual, but for their whole family, as I understand. And if you have a family history, conditional on having a family history of schizophrenia, the individual risk for just conditional risk might be as high as 10%.
So in your analysis, you looked at what would happen if you could use a genetic predictor, polygenic predictor of schizophrenia risk, and you had some number of embryos, let's say, I think was the number four, one of the cases that you looked at was ...
Shai Carmi: We mostly looked at five embryos.
Steve Hsu: I'm sorry, five. So say you pick the lowest schizophrenia risk embryo out of five. You could have a relative risk reduction. I'll define in a minute what that is. Relative risk production of something like 50%. Was that your result?
Shai Carmi: Yes.
Steve Hsu: Okay. Now relative risk reduction means if the embryo, you know, if there was some pre-existing risk for that family, then the reduction was 50%. So by going through this procedure, they would have half the amount of risk. However, the absolute reduction in risk maybe is only 1% down to 0.5%. If the family doesn't have any history of the condition, the absolute risk reduction. Whereas if they had a family history, the absolute risk reduction might be much larger, like maybe from 10% to 5% or something like that. Is that a fair summary of your results?
Shai Carmi: Pretty much. Yeah. Would you like me to expand?
Steve Hsu: Yeah, absolutely. Please do.
Shai Carmi: Yeah. So, fortunately, I mean, I didn't have any personal encounter with this disease. The interest in this, specifically this disease, comes mostly from my colleague Todd Lencz with working specifically in this field of psychiatric genetics. But also I tend to believe that those psychiatric diseases are probably going to be popular targets for polygenic embryo screening, either in companies like yours or elsewhere because they can be so devastating.
So to find the expected relative risk reduction. So yes, we assume that there are five embryos available, and then we use two approaches to compute the relative risk reduction. And in both approaches, we assume that the parents would sequence the genomes of all five embryos and compute the polygenic score for schizophrenia, for each of those embryos.
One important point is that we are assuming that the parents are considering only this single polygenic score. They are only considering this one disease, not testing for multiple diseases. There's something we can talk about later. And then the parents are going to be transferring the embryo that has the lowest risk score for schizophrenia.
And then we use two approaches. The first is mathematical. A statistical model, which is called the liability threshold model. And which are states basically that each disease has, um... So disease is a binary trait. Or a binary variable individual can be healthy or sick, but it is assumed that each such disease has nevertheless a continuous so-called liability that is unobserved but this is something that we, you know, we cannot measure it. But when the liability is very high, we see the disease, the disease the person becomes infected with. The disease appears.
This happens to be a good model for fitting various types of genetic data. And the assumption is that this liability has a genetic component and a non-genetic or environmental component. And the relative sizes of the genetic and the non-genetic components are dictated by the readability.
But we don't even know the entire genetic component. We don't know every single gene, everything, every single genetic risk factor for the disease. We only know the polygenic risk score, which is basically just a number of risk leads that are carried by an individual based on results of genome-wide association studies.
So these studies, they look at cases and controls. They try to find associations, they find typically hundreds of layers that are associated with the disease. And then for a new individual, we just count how many risk areas we have. It's a good idea to do a weighted average, to give a little weight to each person by the risk of carrying that life. But these are technical details. Eventually, we are still under the liability is, has a genetic component represented by the polygenic risk score and then a residual component, which comprises all the other genetic risk factors plus environmental factors.
And then under the very reasonable assumption that these components are normally distributed, then we can compute the distribution of the polygenic score for the embryo that will be selected. And from that, we can compute the risk of the embryo to be affected by the disease as an adult. And then we can compare it to the average risk in the population. And from that, we can compute the relative risk reduction.
That was the first approach. The second approach was to use real genomes from cases and controls. And so we can not do an experiment. There's no data we can do. There's no data we can get from an experiment where we would actively select an embryo based on one strategy or another. So we still have to rely on some, to some, some degree of simulation.
So we take genomic data from cases and controls, in this case of schizophrenia, cases of schizophrenia and the individuals affected with the disease. And then what we do is we generate, we call it virtual couples. So we made individuals at random to create pairs of father and mother. Although they don't even have to be male and female, we just create those pairs. And then we use, I would say standard methods from genetics to generate, to simulate genomes of children.
And then once we have the genomes of children for each such virtual couple, we can compute the polygenic scores of the children. And then we can compute the risk of the children to be affected based on models that were learned for the parents.
So we use the parents, we have those case-control genomes to learn what should be the risk of an individual based on the polygenic score. And then we use these models to compute the risk of each embryo, and we select each simulated embryo. And then we do this experiment, a computational experiment, where we say, okay, what is going to be the risk of the embryo that has the lowest polygenic increased score for schizophrenia.
And then you compare it to selecting a random embryo, you know, equivalent to the prevalence of the disease in the population. And again, from there we can compute the relative risk reduction.
And what we found is that for schizophrenia, the polygenic risk scores that we have right now, in technical terms, they explained about 7% of the liability of the variants in liability of the disease.
It's a way to quantify, to measure the predictive power of the polygenic score, which is not, not very high, but it's also not very low. It's typical for many diseases. Most diseases today for the gene scores explaining the variance of about five to 10% of the liability.
And given this parameter and given that we have five embryos for each couple, then our estimates were that the relative risk reduction is going to be between 40 and 50%. As you mentioned, this is the relative risk reduction. It's not the absolute risk reduction. So if the disease has a prevalence of 1%, this means that the absolute risk reduction is going to be only 0.5% or, or more correctly 0.5 percentage points.
And this is something that we need to be aware of that risk reduction that may seem very large as a relative risk reduction is actually quite small on the absolute scale. In both forms of both ways of presenting the risk and correcting it. But I think it's, it's usually better to present both to make sure patients know what, to, what to expect.
The last point that I mentioned again, as you said, like what happens when one of the parents is one or both parents are affected and, of course, it increases substantially the risk of the child. So there's, you said, I think these are about the right order of magnitude. The children of an individual with schizophrenia, have a probability about 10 times higher to become affected compared to random individuals. So it's about 10% prevalence.
And what we showed is that the relative risk reduction still remains. I think if I remember correctly from the numbers reported, I think it's a little bit less, maybe not 50%, maybe closer to 40%, but it's still quite high.
And the important thing here is that it's also relatively high on the absolute scale. So a risk reduction can be four percentage points which is perhaps more meaningful.
I thought that these, their visions or, or this part of the modeling that we did is quite important because this might be, these might be the first use cases for polygenic embryo screening, perhaps not in the U.S., but in other countries, these might be cases where parents will ask for, for their embryos to be in screened or prioritized based on polygenic scores in case one of them is affected by schizophrenia or some of these other diseases.
Steve Hsu: Yeah. On that last point at Genomic Prediction, a lot of the parents coming in who request our embryo reports, they have a family history of some pretty impactful condition like breast cancer. I don't know if we've had schizophrenia yet, but breast cancer and heart attack are two of the big ones where, if there's a family history, then the family is aware of that. And if they're not carriers of BRCA or some other Mundelein variance that they know it's polygenetic in nature and then they're interested in selecting an embryo, which is lower risk.
I wanted to ask you a technical question. So we did some complementary calculations where it turns out in UK Biobank and in other data sets, there are siblings. And so we were able to look at siblings who, you know, their average age is in the sixties or something. So they've already lived, you know, a big chunk of their lives. And then you can ask how well did the predictors allow you to predict which of the two siblings has the condition in which one doesn't. And for more common conditions, you can easily assemble enough data to figure out, say how well the heart attack, the CID predictor works, or hypertension or whatever it is.
And it's interesting because our, those are based on real people, but we get very similar results to what I think you and other groups got from more, a little more simulated kind of analysis. So that was quite interesting at a scientific level.
I had a very technical question I wanted to ask because we've thought about making synthetic babies, not, I mean, on the computer, in silico. So the question I always have, and this is, you know, exposing my lack of biology knowledge as a dumb physicist, is when you have meiosis and you make gametes, so you make an egg from the mother or you make a sperm from the father, we sort of know that chunk sizes that, you know, the gamut only gets half the half DNA of the, say, the father. Do we know much about the distribution of how those chunks are determined in meiosis? Like I would think this is something that you could actually answer this question through data now.
Shai Carmi: Yeah. Yeah, we know. We have a pretty good idea. And we have something called a genetic map, which basically for every position in the genome or for every small window of the genome, it provides the probability of having recombination crossover in that window, in each meiosis, in each generation.
So with these gigantic maps we can easily model crossover. That's exactly how we implemented our simulations. And these ideas are also very commonly used when people are trying to study, for example, methods for detecting relatedness. So, this is like, if I have a new method to detect the relatives, then I will need to simulate these relatives and this is how it's done.
I would have the two chromosomes from each parent. And then now I need to draw the positions of crossovers. And the standard assumption is that the position is uniform along the genome. It's not exactly accurate. Also, separately, I wrote a different paper about when this assumption does not exactly hold. But broadly, it's fine to make this assumption and simply then it's a personal process. You know, if you need to go even more technical. And the rate of the process is simply determined by the total so-called genetic map of the chromosome.
So this is how we drew the chromosomes. The one problem with this method is that we don't really have the genomes of, we don't really have the chromosome sequence that the haplotypes of an individual. We can only infer them computationally using something called phasing, which is imperfect.
In a sense like every, like each of the chromosomes that we think we are for an individual is actually a mix, a mixture of the two chromosomes of the individual. But at least over a relatively short range, something like a hundred KB or one megabase, we have a phasing that is quite accurate. So I think what's important is that we get the local LD structure. We get it right into that in the children. But otherwise the precise positions of the crossovers, it doesn't matter that much.
Steve Hsu: I see. Wait, so as a first approximation, you can take it to be a unit, just a uniform probability at each position for….
Shai Carmi: Yeah. There's something called crossover interference, which means that not only in humans, in many organisms, there are two crossovers that cannot be too close together. There's like a repulsion between crossovers. And it can be modeled, but overall it's not very important, I think, in this for the purposes of studying risk reduction.
Steve Hsu: Yeah, I mean, if, if the predictor is super polygenic, this will kind of come out in the wash, I guess. The sort of basic biology question that I was ignorant of is I thought I had heard that there are quote, recombination hotspots where the probability just shoots up. And the question is whether you have to model that in your….
Shai Carmi: Right. So we did, as I explained, like, like we have this genetic map, which is you know, in, in windows, that our hotspot there is higher probability. So maybe just to correct something I said before, we select the position of the crossover uniformly, but in genetic map units. Yeah, and that takes hotspots into account.
Steve Hsu: Got it.
Yeah, so that's a very beautiful work and extremely useful, right? Because if you can generate synthetic siblings or offspring, right, there are many uses of that. And you actually hinted at another one, which is genetic genealogy and DNA forensics, which maybe will, hopefully we'll get to that later in our discussion.
Shai Carmi: Yeah. I can also say that. In terms of working with siblings, I think it's a great approach because it avoids some of the difficulties that we have with simulation. So I think the genomes of the simulated embryos that we generate on the computer, I think they are pretty realistic, but the problem is we still have to rely on a model for the risk, given the polygenic score. And by working with actual siblings from the UK Biobank or other datasets, this problem is avoided. So it's a lot more realistic.
The problem with working with siblings, it's more difficult to find out what happens with more than two siblings. So maybe I'll just take the opportunity to tell you about our previous study, where we looked at screening embryos for height, where we did a similar experiment, but we had a really unique and interesting data set.
We had a data set of about 400 Ashkenazi Jews from families from very large nuclear families, families were both parents. And the number of children was anywhere between three and 20. The average number of children per family was 10 and they were all adults. So we knew the height.
Steve Hsu: Are these orthodox families?
Shai Carmi: Yes, exactly. There were Jewish Orthodox indeed. So in this community, typically there are very large families and that was quite nice because we could study empirically what happens if you select, you know, the embryo. I mean, it's not really an embryo. It's like, it's [unclear]. It was a thought experiment. What if these children were embryos? I mean, it's the same thought experiment as you did with the UK Biobank. But we could go into very large numbers of children. But we only had height. It was only the study of height, unfortunately.
Steve Hsu: I wasn't aware of that. So thanks for bringing that up. So I'm curious, first of all, the height predictor. If it's trained on sort of a general European population, what do you know what the falloff in a variance account for or correlation is when you use it on Ashkenazi jews?
Shai Carmi: So it's not too bad. I mean, from all of our experiments in working with polygenic risk scores or putting scores in general in the Ashkenazi population, performance is quite similar to the European population. Actually, recently, just a month ago, we published a paper. I was a minor author on that paper. It was led by Florian Privé and Bjarni Vilhjálmsson from Aarhus. They looked at the UK Biobank and studied the transferability of the relative performance of polygenic scores across populations from within the UK Biobank. So there isn't a problem of using different arrays. Exactly. Genotyping platforms and so on.
And we found that so relative to Europeans, the Ashkenazi population, the performance of the polygenic score is 85%. So there is like a 15 percentage point decline in the performance of the scores, which is not too large.
So these scores work very well, even the schizophrenia study in the newer paper on diseases is also from the Ashkenazi population and performance was quite good.
Steve Hsu: Yes. That's great. So this large family idea is excellent because I often find people have the wrong intuition about how much variance is present amongst their children. So some people think there's no variance among children, which is very strange, right? Because I remember in high school, you know, there were families where one brother was, you know, six inches taller.
That's a little bit rare, but you know, four or five inches taller than the other brother. And so it immediately tells you, or one was a really good student and one had a lot of trouble in school. So intuitively I think if you're a careful observer, you know, that within a family, the kids can really differ. Or if you're a parent, even the kids can differ a lot. But I think obviously you can quantify this, right? So it's sort of half the variance in the sibs as in the general population.
Shai Carmi: Yes. I mean, I agree that it's not, it's not obvious intuitively. I mean, maybe even, you know, if you had asked me like five years ago or 10 years ago, what should be the variance within the population then I wouldn't have guessed half. But it's a classic result in quantitative genetics that variance within siblings is half the variance in the population.
And I think this is, you know, relating to the statement by the European Society of Human Genetics. I mean, that was, I think, one of the main issues where I had a disagreement there. But it's kind of typical of a lot of the criticism that I've heard about polygenic embryo screening. So I mean, a lot of criticism is, you know, maybe justified and worth, or at least worth discussing. But that point, I think is just I mean, you just have to be familiar with these mathematical results. And the claim that there is not enough, a variance I think is simply the, it does not hold.
The fact that there is a half the variance as we showed in a previous paper on traits doesn't mean the gains are going to be particularly high. For example, in that paper, we showed that for IQ in that paper, we talked about selecting out of 10 embryos. The gain, the average gain in IQ was only around two points or three IQ points, which is, I think almost everyone would agree, is not very little. But for diseases it turns out that the larger, the effect can be quite large.
Steve Hsu: Yeah, I should point out that our company only does health-related risks. We don't do IQ or anything like that. Or height.
So you mentioned this ESHG, so that's European Society of Human Genetics, and they wrote a viewpoint, I guess, which was kind of negative about embryo screening.
And I guess my attitude is like yours. If somebody has a valid, scientific point, then I want to understand it as much as I can. I have a responsibility to understand it as much as I can and discuss it with them. But then if they have some argument like, oh, there's no variance among sibs, that's why this is not even worthwhile doing.
It seems kind of wrong-headed and you wrote a very nice, I think it was kind of like a one-pager that you put on Twitter, which really dissected their statement. And I think I agreed with you on every single point.
Now, to me, the really valid issues that they raise obviously are things like, well, we don't have the predictors as we were just discussing. They work best in the populations where we have the most training data. And the more distant you are from those say European populations, the less, well the predictors work, that's the current situation. And that's a problem the whole field has to fix, not just anybody involved in IVF or embryo testing.
And the other issue I think is that there has to be, I think you said this as well, there has to be a big society-wide discussion of how this should be regulated, what should be allowed, what should not be allowed. Of course, it should be based on the best science. Right? So it shouldn't be people who aren't familiar with all the recent research, giving their opinion. It should be an informed opinion.
Shai Carmi: Correct. So I mean, there was some criticism that I agreed with. And we also raised some of the points in a comment that I wrote with colleagues bioethicists colleagues. But yes, I mean, some data is out there on the expected outcome. So no reason, no reason not to use it.
And of course, I mean, I think everyone is aware about that. Everyone is aware of the issue with what was the lower performance in non-European populations or, you know, individuals with non-European ancestry.
And I think there is another issue with mixed individuals that I think was not mentioned, or we don't discuss enough. I think I hope to do that in our group or study it in the near future. And maybe it's a good opportunity to mention this, is that what happens if we have, and that makes the individuals like, like if the couple, if one or both individuals of the couple are unmixed and for example, African-Americans or Hispanic in the context of the United States.
Then the problem is that what happens is that the embryos will vary in the proportion of European ancestry that they have, because individuals themselves, they will have ancestry, partly European and partly other. And, for example, African. And then because of the randomness of meiosis, so-called Medallia and segregation, some embryos would have more European ancestry than others.
And then the problem with polygenic scores. Actually, there are two problems associated with non-European populations. One is lower, predictive accuracy. And the other is bias. So the polygenic risk scores at least tend to be lower, reflecting low-risk in European populations. And if we are going to be selecting an embryo based on having the lowest polygenic risk scores, what may happen is that we may simply be selecting for more European ancestry. And this has the obvious social problem, but also it means the risk reduction that may be achieved may not be as high as we expect in Europeans.
That's a bit more subtle I would say a problem with, implementing polygenic embryo screening in the context of mixed individuals. But I think it's worth thinking about as well.
Steve Hsu: Yeah, I think it's a very serious problem. And, I think one of the interesting ideas that we've discussed is if you can do block by block. Haploblock by haploblock identification of which ancestry group that chunk of DNA came from, then you might apply a specific predictor. So, you know, one trait in Africans on certain sub-blocks and then one trait on Europeans on certain other sub-blocks, and get some kind of aggregate score.
But, of course, this requires a lot of research to really be able to do it.
I think another thing that I guess is a broader issue, but one of the things that I think the ESHG letter or viewpoint said was that there's no way to know whether any of this. Maybe I hope I'm not straw-manning what they said. But it seemed like they said, you can't know whether any of this ever works until lots of these babies grow up. And, you know, you decide. Did the polygenic risk really correspond to the actual risk for that set of kids? That seems like a very unrealistically high standard. And I'm curious what you think about that. I think you commented on that.
Shai Carmi: Right. Yeah, so I think they are aware that it's infeasible to do these experiments. So let me just reiterate what they were saying. They were saying that if we have this, possible intervention of prioritizing the embryos based on polygenic scores and then transferring based on these scores. If you want to do this intervention, and show that it has health benefits, we need to do, let's say randomized clinical trial, where we would, you know, randomized some couples to select, select the embryos by polygenic scores and others to select embryos at random or based on morphology and so on. And then wait, you know, a few decades until there are health outcomes. And then see if this method works to reduce the disease burden. So what they said is that, it would be impossible or irresponsible to use polygenic embryo screening before such trials are conducted. So I think they are well aware that it is impossible to conduct such trials.
I don't think they're seriously suggested to do them. But I think their point was that it would be because it would be impossible to run a trial as it should be. Yeah, we should be careful… We should be very careful with using polygenic scores with embryos.
But I think that we have the tool of statistics and we can develop a model or, or, you know, even simulations, like based on the UK Biobank as you did. And I think these tools can give us a reasonable estimate regarding what we expect. And I think what is important is not the result of one specific model or another model or one or another paper, but the triangulation of evidence. If we have evidence coming from multiple methods, multiple approaches, distinct approaches, or in distinct groups that show similar results, then I think we can have trust [in] these results [to] some extent at least, you know. Get started with implementing this, you know, this screening by polygenic scores.
Steve Hsu: Now, the analogy that pops into my head. Well, I guess there are two. One is that we already do, or it is fairly common to do mendelian screening against rare Mendelian variants. And sometimes in those cases also it's not fully understood any kind of causal biological mechanism by how the mutation is actually causing the disease. But it's just a statistical association between the presence of that variant and later development of the disease.
Shai Carmi: But I would say that for most. I think the difference is that for most Mendelian mutations, I think most of them were we understand that the cause, or at least the evidence is overwhelming.
I mean, as I find it difficult to find, I mean, how many diseases we have for which we don't understand the biology of how the mutation causes the disease.
Steve Hsu: Okay. I'm not an expert on this. But to me it sounds like, I guess being kind of skeptical, always about biomedicine a little bit is that sometimes I go to a talk and they're explaining to me what the mechanism is, and it looks like they just draw a cartoon. And then really they're secretly, they're appealing to the statistics to justify their belief in this. But the mechanism sometimes, I mean, obviously if they have some intervention or something, very definitive biochemical tests that can support the hypothesis. Sometimes it seems like they're confident, but maybe the main source of the confidence to me seems like statistical data.
Shai Carmi: Yeah. I mean, in some cases, yeah. I don't know enough to say how widespread this is. But I think it's not at least in my opinion, I don't think the fact that we only have statistical evaluations of the expected outcomes. I don't think it should. I'm not saying we should approve the technology because of one statistical evaluation or another, but I think we should definitely consider, I mean, simply because we can not wait for trials.
I mean, this is clear. I think for everyone that it is impractical to do these trials and to wait for the outcomes. So we would have to decide whether it should be approved or not based on what we have right now, based on the tools that we have right now.
Steve Hsu: Right. And again, I think it's not that different from, you know, when the FDA has to approve a particular drug. When I looked at the analysis some years ago, my doctor, I had a high cholesterol readout, which was anomalous, but he immediately said I should start taking a statin. And so I started looking into statins and at the time that the data supporting the efficacy of statins seemed pretty weak to me, but it had been approved. It was already starting to be a billion-dollar business or something. And this Alzheimer's drug, I think it's called Aduhelm. That also seems to have been approved on fairly weak statistical data. So I feel like the evidence in our papers is much stronger than that. It seems like it to me, but I like to hear your opinion.
Shai Carmi: I don't think I know enough to give a confident assessment of these other drugs. But I think, you know, particularly not on Alzheimer's drug, the one difference is that for these drugs, or, you know, in general, let's talk generally. Not about one drug, or another. In general, for drugs, there have to be a clinical, like randomized clinical trials, so you measure the efficacy, you know, you eventually you have to run a statistical test and you know, to get a P-value, but you have outcomes from people who are treated compared to people who are not treated.
And this is something that we will not have for polygenic embryo screening in the, you know, nothing in the near future and also not in the distant future. And I think that, in my opinion, that's a big difference. But whether that's a difference big enough to justify banning polygenic embryo screening, just based on that, probably not in my opinion.
Steve Hsu: Yeah. I agree. I mean, that is a fundamental distinction. I guess when I was comparing to these other sort of tests of statistical tests for drugs, I was thinking about the delicacy of the statistical analysis and how, you know, you, you have to make some assumptions in the analysis and for the, again, not to get into a specific one, but I looked into this because I was interested, the Alzheimer's one, Aduhelm, you know, there's negative risk too. If you take the drug, there's some bad things that can happen to you. And so you have to trust their analysis to decide if the net utility is positive for people taking this drug.
Shai Carmi: That's right. You have to. Yeah. I mean, I agree sometimes the statistics can become tricky but you know, sometimes you have cases like the COVID vaccines, for example, where you don't read any statistics.
Steve Hsu: Right. Now another criticism often against this kind of thing, against embryo selection, comes from something called pleiotropy. And I don't recall where the ESHG viewpoint mentioned pleiotropy, but, you know, we looked at this pretty carefully and it, as far as we can tell for the major polygenic predictors for the major diseases, there's actually fairly modest pleiotropy.
And in fact, if you select on an index. So this is something that the doctors immediately demanded that there be some kind of general health index that they could use because it's just simpler to think about for them and simpler for them to explain to patients. That if you select on an index, generally, there doesn't seem to be a zero-sum thing going on, that you can actually improve. You can lower risks across a bunch of different risks. And this seems to be supported by other people who independently are studying things like longevity and such that you can build a kind of polygenic longevity predictor. I'm curious what you think about this, or if you've studied this at all.
Shai Carmi: So we studied this question in both papers. In the 2019 paper on traits and a recent paper on diseases. In the 2019 paper on traits, we found that if that action is done for so it's a bit tricky that the gain per trait, it decreases with the number of traits that are, that are considered.
So what we showed in the paper is that the gain that you have in the traits of the future children decreases, with the number of traits that you're selecting for, and it's inversely proportional to the square root of the number of traits. So if you're selecting for four traits, the gain per trait will be only half what you get had you selected for only one trait.
And so, you know, and if you are selecting for 16 traits, you will get on a quarter of the game. But, on the other end, you're going to be getting gains for more traits. So the net benefit is positive. So you will gain more by selecting for more traits in an index from all diseases.
But again, for each individual trait or individual disease is going to be, it's going to be smaller. Which I think once you think about it, once they complete the thing, it's also intuitively clear that this is what should happen.
And so the thing is with diseases, I never studied it myself, but from what I've seen, I mean, there is a correlation between diseases, genetic correlation between diseases. But the thing is that, usually, this correlation is positive. Usually, it's not very strong. I mean, of course, except for diseases in the same, very similar diseases like schizophrenia and bipolar disorders. Of course, there is a very hygienic correlation. But in general, if you, you know, across all domains of medicine the correlation is really not large and it's a positive.
So if you're reducing the risk of one disease, you will be reducing the risk of another disease. So I tend to agree that once there is an index of, like a health index of some sort, either based on longevity or based on a combination of diseases, it should probably reduce the risk of most diseases simultaneously.
Although, as I said before, the risk reduction is going to be much smaller than like the 50, 40, 50% that I mentioned earlier when you're selecting for a single disease. But I think what, you know we have to be careful about is specific pairs of diseases that have very strong other correlations. Because this can happen sometimes it's not very common. But if a couple is about to select for one specific disease that has high anticorrelation, a strong negative correlation with another disease, then this should be taken into account because our results from the 2021 paper showed the disconnect can increase quite substantially the risk of the correlated disease.
So to summarize broadly, I don't think it's, I don't think it should be a major concern. Again, because of the mostly positive correlation, positive genetic correlation between diseases. But I think this is something that everyone should have in mind, you know, for, for some specialized cases.
Steve Hsu: That was a very nice summary. So if you just assumed uncorrelated risks across the different diseases, then this one over square root N scaling that I think that you gave is, is very plausible, right? It's what you would guess. And if you look at net consequence, you have sort of N gains and one over root N reduction. So you get root N gains.
And then, as a kind of caricature, we find the same thing. Generally what you find is weakly positive correlations. And so weekly, positive actually helps you. So you get, I think roughly — very roughly — like the way a physicist would say it is, you're going to do a little bit better than root N gains.
Shai Carmi: Yes.
Shai Carmi: Right, right. But again, you have to be careful about specific cases, like specific types of diseases that can have very strongly negatively correlated other diseases.
Steve Hsu: Yes. The way I think I can describe the way that often the clinics and the parents are using these reports that we generate is that the doctor, honestly, this is maybe a flaw in the American system because the doctors are always, you know, unfortunately, they have kind of a motive where they're just trying to do things as simply and quickly as they can. Generally, they like having an overall health score. But then the parents, especially if they have a family history or something, they're looking specifically at a line item risk. Like, okay, I understand that overall ranking using an index. But then I want to see what is going on specifically with heart attacks because a lot of people in my family had heart attacks or something. So that seems like a reasonably rational way for people to use this information. It doesn't seem like people are having trouble making use of it.
Shai Carmi: Yeah. Although I would be, I mean, I would be careful in that case about, I mean, in that setting about the expected relative risk reductions, if the identity of the embryo of the selected embryo is eventually not the one with the lowest or highest health index, maybe it would change the calculations to some degree.
Steve Hsu: Yeah, it's very subtle. I mean, the genetic counselors that we train, it takes a while for them to explain, you know, RRR and ARR to the patients. But it's, it's the real focus of trying to give them a clear picture of what, what the information says.
Shai Carmi: Yeah. I mean, your experience would be very interesting. I mean, I assume you will be, you will publish at some point all the data that you have on the patient experience. But, but, but these are indeed the kinds of concerns that that we raised that, you know, maybe negative sides with with polygenic embryo screening, like the we referred in some places to choice overload, just having too many, too much information to, to comprehend and, just having to select out of a few embryos may, may make it difficult for parents and also difficulties in explaining the probabilities, because the risk is probabilistic. It's not deterministic as, as the case of, you know, systems proposes or seminal diseases. And also explaining the distinction between relative risk reduction and absolute risk reduction.
I think all these points are, they are causes of concern, you know, in the greater reproductive medicine community about this technology and, you know, any data that you have on this I think it would be very welcome, you know, by everyone studying this.
Steve Hsu: Yeah, I think I agree completely on the importance of these issues and I'm pretty sure the company will publish some results on all this stuff. I'm more involved in the computational stuff. Not that sort of thing. I probably wouldn't even be a co-author on that paper, but I'm pretty sure they will.
[music]
Steve Hsu: This is Steve, adding some information in after-editing. I checked with my colleagues on Shai's question about followup studies of our patients. Genomic Prediction is preparing more than one paper on this kind of data from patients consenting to participate in research, which is most of them. The studies explore patient experiences and attitudes toward polygenic screening, and gather not only longitudinal health outcome data, but also the psychological outcomes of screening - whether the patient found PGT-P useful, whether it induced anxiety, relief, or regret. Whether reducing risk of mental health disorders in their family gave them courage to have children. Whether their family and friends approved, or whether the patient elected to keep the testing a secret from their peers, or even from their own child. Or whether they decided not to receive PGT-P results at all, and if so, why not - so far, more than half have said yes, when PGT-P results were offered. All very interesting stuff, which the company looks forward to publish. Some of it has already been presented at an IVF science conference called ASRM last year. You can find more about the study designs at lifeview.com, and also on their public pre-registration on clinicaltrials.gov. They have been IRB approved and submitted before initiation, in accordance with best scientific practices.
[music]
Steve Hsu: … Okay. Well, we're at an hour now. Sorry. And there are two more things I want to discuss. If you can just give me another, maybe another 10 minutes. That would be great. Okay. So one is genetic genealogy and solving crimes. Cause you wrote one of the early papers on this, which I think I, at the time I read and was influential in my thinking, because I had been making kind of back of the envelope estimates which were similar to, with conclusions, similar to what you got.
And then the other thing I wanted to discuss was the Israeli health system. And whether you think there's a good chance that polygenic scores for adults might make an appearance in, I think it's a single-payer system. Is that right?
Shai Carmi: I’ll explain once we get to that.
Steve Hsu: Okay. Okay. So let's, let's do five minutes or five or 10 minutes on genetic genealogy, and then however much time you can spare on health care.
So this other company I'm involved in is called Othram. The CEO tells me it is solving several really prominent cold cases a week. These are like cases that have been outstanding for a decade or maybe sometimes multiple decades. And, you know, they're able to, I think they've solved cases with as little as 15 cells, equivalent of DNA. And so they're getting really good at dealing with contaminated, messy DNA and crime scenes and getting a fair amount of case volume from police departments, FBI, stuff like that. So it's a real thing. I think it will change law enforcement quite a bit. And again, I want to give you credit and I forgot your collaborative's name.
He's a computer scientist at Columbia.
Shai Carmi: Yeah, the person who led this, that paper in 2018, is Yaniv Erlich.
Steve Hsu: Yes, Yaniv. So I heard him give a talk at ASHG, I think it might've been 2018 or 2019.
Shai Carmi: 2018 just after we published the paper. Yeah, he was at Columbia and the same department where I was in computer science. And then, in 2017 I moved back to Israel and it was the CSO of MyHeritage. And I'm a consultant at MyHeritage, as I mentioned. So we worked together on this paper.
It was mostly led by Yaniv and we also use data by MyHeritage to generate those projections for the ability to identify at least Americans based on their DNA and this genetic genealogy approach.
Steve Hsu: Right. So I, as I was saying, I saw this as an opportunity to solve crimes and do things and I was making much simpler estimates. And so when your paper came out, I was like, wow, this is great. And, so I think one result you had was
Shai Carmi: Yeah. I mean, that's, I mentioned the paper by [...] and Graham Coop. They also generated some estimates of the expected number of people that we will be able to identify using this method. It comes in parallel to us.
Steve Hsu: But I think roughly speaking, you were saying, if you have roughly a million people in your data set, and let's say, we're just talking about Europeans. I think you said 60% chance of finding a third cousin match. Is that...
Shai Carmi: Yeah, that was, yeah. Those were about numbers.
Yeah. It's all very sensitive to like, you know, modeling assumptions, but, but that's the order of magnitude. Once the database has become large enough to have, you know, 1%, 2% of the population, then it becomes highly probable that everyone would have a relative, at least a third cousin or closer in the database.
Steve Hsu: Yep. Now there's been a whole evolution where a lot of the initial data used for this came from kind of enthusiasts. Genealogy enthusiast websites, where people had uploaded their genomes. And then there was some controversy about whether some people were willing to allow their data to be used to catch criminals, et cetera. And so the whole thing has evolved in a kind of [pretty fast].
Shai Carmi: Very rapidly. Yeah. Just quite amazing. How fast things developed here.
Steve Hsu: Incredible. And actually at the science level, the results, like Othram knows a lot now about sort of the corrections to the first order kind of analysis that you and Graham and these other guys did because they see much more population structure, right.
And population structure, even among people that are more likely to commit crimes or, you know, there's a bunch of science that they could do if they were not so busy building the company, they could publish a bunch of papers on this kind of thing. But, I'm curious what you think will happen. So one of the hypotheses I had at the very beginning when I was talking to venture capitalists and looking for a CEO to run the company and saying, we should really start a company to do this.
I thought that there would eventually be legal challenges that would just force Ancestry and 23andMe and MyHeritage to, you know, allow their data to be searchable by law enforcement because a very similar thing happened at the beginning of the internet where the police would always want to go to an ISP or some email free email provider and say, hey, there's a crime being committed and we need to look at all these emails. And initially, those companies resisted, but then the police got court orders and things, and now we've flipped completely to where all of those internet companies have compliance departments whose only job is to basically comply with law enforcement to, you know, dig up the data that the FBI wants or something.
So I'm wondering what you think will happen in the consumer DNA business, in the long run regarding, you know, this kind of thing.
Shai Carmi: Yeah. I mean, let me first, just a comment about , you know, development, like scientific developments in the field very quickly. I think that, I think that you commented correctly that there are not too many developments, at least on the, on the question of how to find the relative or how many relatives where we expect to find.
I think most of the challenges come from extracting DNA from sometimes highly degraded samples. Sometimes even almost enshrined samples. It can be, you know, decades-old samples. Sometimes there are mixtures. And so all of those complications and then sequence these DNA samples to high quality.
I think these are mental challenges that are still not fully resolved. And I think there can be a lot of progress there that will make applying this method easier. And I think that there are also challenges in the genealogy part. So once you have your second cousin of the, of the criminal or the or the victim. Sometimes these are unidentified victims. Once you have them, once you have to say, once you have that second cousin, it's not always easy to find the person you're looking for. And I don't think there are like a, I don't think it's like, there's been major development in methodology that would help a genealogist to do this work.
I think it's still kind of a lot of the cumbersome manual work that has to be done. And, the question is how many relatives we will detect. I don't think this would change too much. I think now that databases keep growing at some point it would be just, everyone is going to be nearly everyone is going to be, is going to have a relative. So this question is going to have less importance.
And your question is about legal aspects and I'm less familiar with this, but I'm also equally impressed as you that there were no court orders. I think there was only one case if I remember correctly, but by and large, the companies that did not want to cooperate with the law enforcement on this, they didn't do it.
I mean, MyHeritage again, where I'm a consultant and ultimately 23andMe and Ancestry that have the largest largest databases, they were very successful, very aggressive in protecting the privacy of their consumers. But also not, not, not helping law enforcement. It's also a question of what's the right thing to do, and it's not obvious, but, but the decision of this company was to protect, protect as fiercely as they can, the privacy of their users. And yeah, it is quite interesting that it's successful.
I mean it is not practiced in Israel, this method, for various reasons. It's a bit complex. I won't get into it. But in the U.S. forensic genetic genealogy is now very widespread. But I think, I think the, I think the law enforcement agency is they, they now simply, they just live with, with what they, what they are allowed to, you know, to use. I mean, with GEDmatch and FamilyTreeDNA, and they probably gave up. Maybe there are some things happening behind the scenes that I'm not aware of. But, yeah.
Steve Hsu: Yeah.
Shai Carmi: Yeah, but just realize that it's just what the, just want to have that court order. So they just should not waste their time on it.
Steve Hsu: My description of what's happening in the U.S., I guess I have some special information, but I don't know how much of it I should share. But there is a lot going on in the background with proprietary data building proprietary data sets. And the police are just happy because this is already, even in the current situation, a big quantum leap over what they could do three years ago.
The local incentive structure for the detectives and prosecutors is they're just trying to clear as many cases as they can. And for them to engage in some protracted legal struggle with say 23andMe, they don't have a good incentive for investing that much of their time and energy to do it. But it could happen easily if like some kind of politically ambitious district attorney or somebody wanted to really push it in, you know, maybe in some red state in the United States. That's, that's, that's the fluctuation that I'm waiting to see. I think that fluctuation will eventually happen.
But right now the police departments are just happy that they can clear a bunch of old cold cases, you know, that they had never had any hope to solve.
Shai Carmi: I think they also have I mean, I assume they don't solve every case using genetic genealogy. They don't always find relatives, but even if they find relatives in half the cases they are studying, they probably have enough work to, you know, for for a few years forward, you know, to keep them busy working on
Steve Hsu: Exactly. Exactly.
Shai Carmi: Yeah. And there out so many rape kits, for example, that, you know, from what I read, that there were not even a genotype. You know, there's so much potential to use it, you know, to use this technology even before, you know, going to court with 23andMe.
Steve Hsu: Yeah. The rape kit issue is a terrible one because, you know, there are many, many rape kits that have not been processed. And it's also true that a very small number of offenders are responsible for a lot of the rapes. So there's kind of some, I don't know if it's a power law, but you'll look, you'll like this from your earlier work, but, but that, there's some kind of distribution where a relatively small number of people are responsible for a hugely disproportionate number of crimes.
And so when, when you lock them up, it has very large network effects or not network effects, but, but disproportionately good effects.
Let me switch gears. Cause I, again, I'm conscious of the time and I don't want to keep you too long. So I'm curious about the Israeli healthcare system. And I just have the feeling that well, I guess maybe that the crudest level people are smart in Israel.
So I'm wondering if, if you'll, if it's possible, there'll be the first to start using PRS constructively for adult healthcare.
Shai Carmi: Okay. So briefly the Israeli system is, so there is universal healthcare, but it's not, there's no single provider or insurer. There are four providers for HMOs. The largest is called the Clalit. It ensures about half the population and they recently became very famous after they published several papers on the COVID vaccine because they have really, really good, high quality longitudinal electronic health card data spanning almost 30 years later, I think.
They also have a really good research institution. And then there is another provider called Maccabi, which covers about a quarter of the population. Then there are two smaller providers. But everyone must be every person in Israel, every citizen must be a member of one of these HMOs.
So regarding polygenic risk scores. They are not being implemented clinically right now, anywhere in Israel. As I mentioned earlier in this conversation, only very recently, we have good data on the predictive power of polygenic scores in the Ashkenazi population. And we know that their polygenic scores work pretty well. Not, not exactly the same accuracy as in Europeans, but almost there. So we know that they should work well in Israel. Regarding the other populations of Israel. We know it is very lethal if at all. I mean, I did it like a very, very small study of 500 people from, in our village, studying LDL cholesterol. And that's it pretty much I think. So there's a lot of w we, we don't know enough about how well polygenic scores are going to work. And we also don't know enough about the attitudes of people towards using polygenic scores clinically. And I'm trying to run a few, at the moment, a few small studies in a context of cardiology and oncology to just take a sample of cases and controls from clinics in Israel and compare polygenic risk scores and, you know, so we know it should work in Ashkenazi Jews. You know, we should validate that first and then see how it works on the other end, you know, in the other populations, particularly the non-Jewish population.
So we have this missing data. What we do have, which I hope will take off, you know, in the near future is a large national project, to set up a biobank of or, you know, a study of a hundred thousand Israelis along with their genomes and their clinical records. And this project has all funding secured from the government, but it ran into some barriers and technical and legal problems throughout the past few years.
Now, there is new management and I would say more excitement about it and things are starting to move on. And I hope that this momentum will continue and then we will have a really large resource of genomic data from Israel. And then of course, we [have] these amazing longitudinal medical records that we have in Israel associated with these genomes, I think it will be very easy to study the utility of polygenic scores. And then I think there would be openness to try polygenic risk scores in the clinic. I think it will be important to have some preliminary cost-effectiveness analysis or preliminary trials.
But from conversations that I've had with clinicians, maybe it's biased by some of the clinicians, but I think they have an excitement about the ability of polygenic scores to identify individuals of very high risk. But I mean, at the same time we have to realize that the sensitivity is very low. I think it's, you know, we know that from many studies that were published, including studies you know, on embryos. We know that just identifying individuals at very high risk is going to find only a small proportion of the cases.
And even the positive, predictive value is going to be, you know, it's not going to be very large. So we have to have that in mind. And still it could be another tool. Another way to stratify individuals by risk and, you know, maybe give them better treatment.
So it's, it's early to say whether, you know, we would have it implemented at scale in Israel, but I think there is a definite willingness to try it out and see you know how it works.
Steve Hsu: It sounds like Israel could be … the other two that I think are promising are Estonia, weirdly, and Finland, because they're relatively small countries and they have good medical records and, you know, already some biobanking going on.
Shai Carmi: Yeah. But, they are a few years ahead of Israel and in terms of setting up their biobank. We are just, I mean, again, there is funding for, you know, the funding was secured already a few years ago. But there were several problems that slowed down, if not, stopped altogether this project.
And only now we're studying more seriously. I mean, this is a project of the ministry of health. I met a consultant there, you know, trying to help with experience on, you know, population genetics, if he's running so on.
Steve Hsu: You know, the example that stood out for me was breast cancer because people are already very familiar with BRCA and the polygenic risk can be as large for a fairly large chunk of women in the population compared to BRCA carriers. And so when you do very crude estimates of how much money you save by identifying it's, it's about an order of magnitude larger, the set of women who are as high risk as BRCA carriers, but only for polygenic reasons.
And it looks like you can pay for, if you just take the cost savings that other people have published in other studies from early detection or early diagnosis, it looks like you can pay for genotyping all the women in a population just by breast cancer.
Shai Carmi: Also genotyping is so cheap now that it's almost, I mean, I don't think it's a major consideration anymore. Like the cost of genotyping. I mean, it can be under $40 now and you know, if you do it at scale, it's even less and also if you genotype, you just need the genotype once for all diseases.
So I don't think this is going to be the major consideration. It's going to take a lot of effort and additional infrastructure and clinician time and so on to actually do the testing and interpret the results and provide you with the counseling and all that. And these, I think these court costs, I think, are going to be just much, much higher and the cost of genotyping.
And this is where, you know, it may end up in the north cost-effective. But I think we can also learn, you know, to do it efficiently. You know, if we are convinced that the scores are sufficiently accurate.
Steve Hsu: Yeah. If you could convince theHMO that they'll actually save money, then that’ll do it.
Shai Carmi: Yeah. That always helps.
Steve Hsu: Yeah. Well, I appreciate your time. It's now a minute, an hour and 20 minutes, so I apologize for taking up so much of your time, but I really like the conversation. Yeah.
I would love to come back and talk to you about Ashkenazi, the kind of history, genetic history. You know, the one thing I wanted to ask you before we go is for Sephardic Jews, do you have similar results for the effectiveness of European trained predictors and things like this.
Shai Carmi: So first of all, I would emphasize that what some people think aren't called Sephardic Jews. I mean, they're really, we have North African Jews and as far as you mean Spanish Jews, and then we have Middle Eastern Jews, mostly from Iran and Iraq. So we know from genetic studies from population genetic studies, we know that North African Jews are genetically relatively similar to Ashkenazi Jews.
They are distinct, but, you know, quite close. So if I had to guess, I would guess that the accuracy of polygenic scores will be somewhat lower, but not too much lower. But regarding middle eastern Jews, I think, I mean, it's really that there's no data and they are more genetically distinct from the other Jewish communities.
You know, we really, we don't have a good clue. I mean, like there is the only middle eastern data at scale that we have is coming from Qatar. Actually, just like a couple of days ago they had a paper out on polygenic scores for cancer, but they didn't have enough cases to actually determine the accuracy of the score.
So really there's no data from the Middle East on the accuracy of polygenic scores. That's a pretty big gap in the literature in this area.
Steve Hsu: Great. Well, I couldn't resist slipping in that last question. Shai, thank you very much for being on the podcast. I'm really happy that we did this and I would love to have you back again if you have some more time.
Shai Carmi: Yeah. Maybe whenever, you know, a new paper's out. That way we have more to discuss.
Steve Hsu: Yeah, that would be great.
Shai Carmi: Yeah It was fun. Thank you for having me.
Creators and Guests
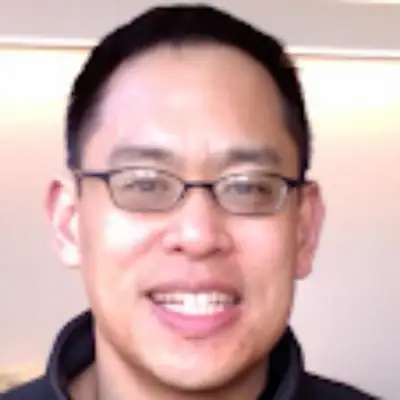