Robots, Small Models, and RL with DeepSeek Alumnus Zihan Wang — #86
Zihan Wang: I definitely think robotics could be one of the biggest improvements for the next few years because like previously all of the robots can just not do any semantic understanding.
But for current larger language models with vision capabilities, I think this is gonna be more true at.
Steve Hsu: Welcome to Manifold. My guest today is Zihan Wang. He's a PhD student at Northwestern University. He was educated in China and is an expert in large language models and AI research. Zihan, welcome to the show.
Zihan Wang: Hi. Nice to meet you, Steven. Yeah, nice to meet you. I'm very happy to be here today.
Steve Hsu: Yeah, it's great to have you on the show. I discovered you on Twitter because you wrote some very interesting posts about AI research and the internship that you did at DeepSeek. And also I think you translated some interviews with the DeepSeek founder, for example.
Um, I, uh, wanna start by talking about your background.
So you're, you're quite young, right? You only graduated from college recently, is that right?
Zihan Wang: Yeah, yeah. Yeah. I actually just graduated like last year, 2024. Like from the university. Yeah. And now I'm a first year PhD student.
Steve Hsu: So in China, is there a name for someone? Like are you called the two thousand generation or something like this?
Zihan Wang: Yeah, definitely. And there is a name like, I'm not sure, like if I directly translate it'll be like zero, zero after zero, zero. I'm not sure. Yeah. It's like a Ling hole.
Steve Hsu: Yes. So yeah. Ling Ling Hall. So, so, um, you're not from Beijing, but you attended university in Beijing. Is that correct?
Zihan Wang: Yeah, yeah, yeah. I grew up in Wuhan and uh, like, I think I got most of my education in Wuhan. Like I got my high school like in, noir middle school affiliated to CCNU, which is like a Chinese, normal university. And also, I think all of my high school students are just so fantastic here.
And actually I just. I came to know that many of them are also working on computer intelligence, and they got very good places to study in the U.S., for example, like CMU, like Berkeley. And I, I, I'm also very fortunate to be studying in the U.S. For example, CHCS PhD. Yeah. And, in terms of my, uh, like college study, I study at a university and I actually, I think it's a little bit underrated school.
Yeah. Actually, I just talked to some, like AI models this morning and let it, like, see whether it's, if it understands or like to know about my undergrad study, undergrad school. And, I just find that their knowledge is pretty old. Like they evaluated my school as some like, top 1000 universities all around the world.
But actually, our university has been publishing a lot of influential papers recently, for example, I think some of them. Professors at, German University, just published a lada like large, diffusion model for a large language diffusion model, which is very popular around X. And also some of my alumni are also launched like Open Manus, which is an open source reproduction of like the very, trending, coding, agent.
I think it's as a general computer use agent, called Manus. And they just reproduce it within several hours after their release. Um, so I think it's fantastic and I think the school and also the university are growing. Yeah. Actually I grew up in, I, I, I got my undergrad study at like galling school of ai, which is very new and I think it just, was established five years ago.
Yeah.
So I. I think there has been a trend in China that a lot of people are trying to study artificial intelligence, not only for studying, using the web, but also for serious subjects, like undergrad study and graduate studies. So I think it's just so exciting to be studying or just studying living in this, in this age, in this era.
Yep.
Steve Hsu: Let me, so let me dig into that a little bit. So I've, I've been to Renin University, if I recall. It's right next to bea, is that right? Very
Zihan Wang: Yeah, yeah, yeah, yeah. We collaborate a lot with Beda.
Steve Hsu: Yeah. But I think in my case, for people my age, I think Renin is more famous maybe for humanities and social science and not as well known for technical subjects.
But, maybe that's changing now. It's interesting that in China there are many programs. It sounds like your, was your undergraduate major actually, specifically AI and not just computer science.
Zihan Wang: Yeah. Yeah. Like, the school is mainly targeted for AI and actually. I think, I'm not sure if, yeah. So I'll use that function. Yeah. Yeah, I think it's mainly because the school, like ING School of AI, was launched five years ago. We are like the CEO of Galling. I'm not sure if its name in English is, high, high field or like high Heel, something like that.
Yeah. Like the,
Steve Hsu: Is it high sense?
Zihan Wang: I'm not sure about his English name, but like the CEO is single. Yeah. He's a very great investor that has invested in a lot of great companies and I think he's one of the top VCs in China. And I'm not sure because I'm not an expert in investing, but I think he really invested a lot into the school, especially targeting things like artificial intelligence and all of that.
like professors at that school are just,I think they are all very like expertise, like they have so much expertise in artificial intelligence, for example, some of them are just,several very early researchers for information retrieval. And this is why like re universities have a very, very high information ratio ranking, around the world.
I think it's. like top five around the world and they just extend this to like general artificial intelligence, for example. They also have many professors that have strong expertise in machine learning and also like computer vision, something like that. Yeah. So I think it's, it's been growing and I think the main reason they have been growing is that there is so much money invested into that.
So, I think that's the reason. And for what you have said about the, like, humanity side of other university, I think this is, basically like, I think this is something unique about it, because it does not only do well in artificial intelligence for these years, but also like they have deep connection with, some other subjects, for example, like humanity or something.
I think the motto of the Golling school is like, to. Like to create some warm air? Actually, yeah, yeah. Something like that. I'm not sure how to translate it directly. Yeah. So I think the school is trying to build, like, fundamental AI research and also make it have some, like humanity, something like, like, like that.
To have like interdisciplinary research. Yeah.
Steve Hsu: Now for my audience, not everybody in my audience knows that much about China. Can you translate what Renin means in English,
Zihan Wang: Oh yeah. I think it just means, people, yeah.
Steve Hsu: the people's University?
Zihan Wang: Yeah, yeah, yeah, yeah. But I, I think originally it's named, like translated to like a people's university, but I'm not sure why they just changing name, maybe they just want some alignment between like, like their, how they are spoke, spoken in Chinese and how they're spoken in English, for example, if we, just speak, people's university and then like, when, when this like English audiences come back to, come to China for a visit, they will not know that re university is people's university.
So they just change it back to the original name. Yeah,
Steve Hsu: So again, for my listeners who have not been to Beijing, there's a part of Beijing where, there are, you know, very big, very famous universities, Beda ing HU and rein are all like, kind of in a triangle there. And, it's, it's one of the main concentrations of brain power
Zihan Wang: yeah, yeah, yeah, yeah. Yeah. I, I think like the center of the triangle is called a
Steve Hsu: And so
Zihan Wang: Yeah.
Steve Hsu: like a place where, for example, Google's office, before they closed it, Google, China was there and Microsoft research. all kinds of venture capital firms and startups and stuff like this. So it's a high tech area of Beijing. I think I was just there actually recently, but, so, Zhan, most people who are older, like professors in the US who at the time you were born, the Chinese universities were not as strong.
I think you even said like in the old rankings, Redmond was, you know, number 1000 in the world. But I think what's difficult for Americans to appreciate, because, you know, America has been a rich top place in the world basically since World War II or even before that. And the rate of change in China is one of the most shocking things for Americans. So when I talk to Americans, I would tell them something like, well, maybe the older professors even at Redmond are not necessarily that great, but the young ones are really sharp and the students are really sharp. So maybe you could comment a little bit on that. Like I, I, I would guess the students you went to college with are as good as any undergraduates in the United States. Do you think that's fair?
Zihan Wang: Yeah, I think actually this is mainly because like the previous scope it is not that much. I think there's a trend that people's education level has been rising a lot in China. Like previously, the GCA acceptance rate is very low, but now, like everyone can go to universities or colleges and there's even something like we call inflation in education level.
But I think actually it's a good thing because once people like to get more educated, they will know more about the world or they, they were just,like having more ability to do something fascinating. So, I think this is mainly because of the trend, for example, because previous professors at schools like LE University are great.
They are doing good research actually. re university is one of the first schools that started database research in China. And so their database ranking has always been very top among other Chinese schools. but at that scope, the, at that time, this is just a scope is so small.
So, like there is not that much voice, both like, in China or just globally. So people do not know that. But I think all of them. so-called necessary people. For example, those who are really in charge of the database systems in China, they all know about the schools and know about how they could contribute to the country, something like that.
So, I think this is just a scope change, you know, like expertise change. But maybe I'm wrong because I actually, I work mostly with those young professors, because I, like, I think my topic is mainly related to the young professor's research interests or something like that. but I do believe that there's still like, those expertise is not for, from today, but, just from, long ago.
But like the scope, the population, or just like the, how, how much money they're putting into the school is gonna be changing more and more today. Yep.
Steve Hsu: So I, I looked at your CV before the interview and it's very impressive because you've already been involved in very cutting edge research projects, both at the university and then probably in your internship at DeepSeek. and you're already, you only just finished your undergraduate degree, right? So, already as an undergraduate, maybe as a senior or junior, you're already involved in pretty cutting edge frontier research. you talk a little bit about your decision about coming to the United States in graduate school? Like for most talented CS majors or AI students in China, do they all wanna come to the US or would they rather stay in China and do their PhD at Chinois? How does that, how does that thinking go for a kid?
Zihan Wang: Oh yeah. So I think these are mainly two questions like my choice and like the other Chinese student choice. So for my choice, I think it is. It's just like case by case because I know about my current advisor. She's a really great advisor and I think she's one of the greatest advisors that I can make all over my career for, like research.
She's really supportive to students, and she has a very strong vision. She has great connections to a lot of pros like cutting edge professors, working in different directions. For example, computer vision, robotics, large language models, foundation models, agents. so, I think I mainly chose her, like not the United States or China, or not like Northwestern or other schools.
I mainly chose her. Yeah, so this is my case. And actually, there's also a lot of people asking why I'm not like them, staying in like my previous affiliation or like, for example, like, both, either REM University or Deep Sea. I like what I am doing. Different directions for today are a little bit different from what my previous affiliations are focusing on.
For example, we are focusing a lot on vision, language models and robotics. so Remy University is good at information retrieval and DeepSeek is good at foundation models. but I think it's a little bit hard for me to get some views or like get some research experiences in robotics for today if I continue studying in China.
That's not because of things like, like, different countries or something. It's just because of my background. But I know that my professor, like my advisor, is currently working on agent robotics, and I really, hope to learn more, just get more research done about it. Actually, I think I have an ongoing project about robotics, but it just kind of started.
Yeah. So, I think this is mainly a choice of direction. Yeah, that's it. And I.
Steve Hsu: Can you describe the situation though for a typical, let's say a kid who went to one of the better CS programs in China and is thinking about getting a PhD. What is their thinking about whether to stay in China or try to come to the United States or some other western country for their PhD?
Zihan Wang: Yeah, yeah, yeah. I think in China it is more like a half, half for now. Like some of the students, like, like for the students who have a real grade bracket, for example, they have several, like first author, peer review paper, like, during their undergrad study, and they have a lot of research experiences.
For example, at the top labs, like in Stanford, like Berkeley. I think it's really half and half. Like some of them go to the United States and some of them like, stay in China for their undergrad, for their PhD studies. I think. This is also a case by case, but I, I think I, I, I'm, I'm not confident to get, to give you like a statistical conclusion.
But I think, for example, one student of mine, like, left, I, I think one, one of my lab mates just, uh, left back, like in China for his graduate studies. He's from the university and he's now at, like Thu University for, PhD study. And this is because like Thu, is one of the best schools in information retreat when even better than re university.
So, he's already studying at the most techy, cutting edge institute. And the, the most tech, cutting edge institute is in China. One, one of one of them, right. So, he just chose that for, for study and also for his connections because he has been interning in that group for some time. So he just chose to, like do PhD studies like in, and some of the.
Hmm. Other of my friends, they come to, United States because, Hmm. I think this is also because of the professors, both for their, how, how they are leading their students or how they are advising their students and for their research directions. For example, one of my friends went to Berkeley and he just went to an efficiency group,because he believed that efficiency could be very important for current foundation model research, especially the efficiency for attention and for the MOE.
And, uh, that group is just doing so well in these directions. So he just, um, um, went there for PhD study. Yeah.
Steve Hsu: So, a few years ago, it seemed like you really had to be at a frontier lab because only they had the compute budget to do the pre-training, and only they had access to the models because there were no really good open source models. So I was a little worried, at that point, that the frontier in this whole field would shift into closed private labs. But now the situation maybe is a little bit different and maybe you can do really impactful research even though you don't have a huge computer budget and you're actually at a university. So I'm wondering if you can comment on that situation.
Zihan Wang: I have a lot of comments on that actually. I can share a little bit about my two recent public release, like the region, the COE, where region is like we trying to, enable the agents to learn from, like, self evolution with reasoning and COE's, chain of experts, where we change mixture of experts a little bit to email them, to communicate with each other.
Actually, both these projects just cost us less than 1000 USD.
Steve Hsu: And, and the,
Zihan Wang: So,
Steve Hsu: was the base model something like Queen 32 or what, what, what model were you using for?
Zihan Wang: We, we are basically, using a smaller version of QM models, for example, like 0.5 billion or just like. Yeah. for the COE project, we utilize the infrastructure of DeepSeek V two public releases on Hugging Face, but we change, since we're doing pre-training, like the checkpoint is not that important, we can initialize from scratch.
So we just change the hyper parameters, to like, make some models smaller, so as to fit our budget. Yeah, so I think that is the key, like, so for previous research you can definitely try to verify your idea with a small budget, but after that, like you can, if you want to enlarge it, you can find some money from other funding resources.
And I definitely believe that, like once this kind of idea shows some potential, I think there could be a lot of money like trying to. Finance or something like that. Uh, yeah, so I, I think this is one of the cases where,like current, cutting edge research, could be done like in some, like, like everywhere.
I think there's also some top 100 university like undergrad students who just find me and say like, hey, I have an idea. I'm not sure if you're interested, but maybe we can discuss it. And I just ask them to try on their own, like cloud resources, like their scale is even smaller, for example, like I just to try like q and 0.5 billion and they can even try a smaller model, but they're, they're just using some collaboration or some other cloud resources.
We just call them like less than 100dollars for each, each month, but they can also get it work. So I think, like current cloud architecture makes it better or makes it cheaper to verify the correctness of an idea and once we can. verify it very initially, we can just, try to, publish it a little bit or not.
I don't, I don't think we should stay published. Maybe like to open it or release it a little bit with our, like, blogs or just like code and like people will see this post and like be, like judge it and then like, USC, like whether your idea could be accepted by the public. Yeah. Mm. Yeah. I think I just want to talk a little bit more.
Yeah. so I think there's another factor, which is the open source infra. Yeah. So I think just the one year before when I was trying to implement something about online learning, which means that the model can generate some trajectories and they can get some feedback and based on the feedback they learn and then they just want to improve themselves.
At that time, this was just so hard to implement because, I think most of the training architecture supports like super fine tuning at that time, but not like online learning because the model,who generates the model must be fixed because,when you want to change the parameter of the model, you actually need very dedicated management of the memory.
So at that time, if you want to generate, make the model generating some trajectories and then use that to update the model, this would be effort. And we are not able to do that. But for now, I think different, infra for example, like the one we're using like the VRL and also like open RHF, and I think there are recently a lot of, lot of infra like, open reason zero, something like that, has been really making like a lot of people, being able to, have their like, like have those open source infra and build, build their own things.
on this infra, I think this just, like standing on the giant shoulder, something like that. Yeah. So, all of the things I think make it less of a barrier for current research if someone would just want to do some research.
Steve Hsu: The infra that you're talking about, were those projects built already based on Llama? Was it because Llama was available and then people started building that infra? Or did it actually require things like DeepSeek and Qwen to exist to drive that infra development?
Zihan Wang: Yeah. So infra basically means that you have a model where you want to train them, you want to correctly train them, for example, if you want to correctly train them. It's not just like data and just like, to run it and calculate the loss function, then do backward and then optimize.
It's basically like when you train a small model, that is okay, but when you train a large model, you need some experimentation. I think you need some to build something for the experiments. For example, you need a lot of metrics and you need to monitor the metrics. And like previous trainers, maybe they just do not, support this kind of function for you to easily monitor these important metrics.
But for now, all of the inference, they just submit the like experimental metrics to a platform called one db. I'm not sure if I'm spelling that correctly. It's,maybe it's one B. They could help you organize and also to see about the different metrics. So you can easily know whether the model is trained well or not.
It's just like, you train a model but you want to know more about it. You just do not, want to only know about the loss. But also like other things like the different factors of the loss, for example, the loss may be like some different things sum up. You also want to know whether your GPU is leveraged.
Well, for example, some of them. People, they just have strong GPUs, but the, like, the leverage rate is low. So they're just wasting their GPUs. With those many metrics, people would be able to learn whether they are really training well or not. But for previous, infra, I think, mostly they are just, make sure that you could make it run.
But, how well that, how well is it? I think there is, like people have been building a lot for today. Yeah.
Steve Hsu: And what's the split between academic labs and other entities in producing this open source infra that you're talking about?
Zihan Wang: So I'm not sure. Maybe, are you asking about their ability or like willingness?
Steve Hsu: No, who's actually, who's actually building it and releasing it? So is it academics at universities or is it, you know, DeepSeek that's releasing it? Who's actually building the tools that you're using the most?
Zihan Wang: I think it depends. Yeah. For example, VIR is developed by. yeah, like dance and like the open Reason zero is built by I, yeah. I, I need to check.
Yeah. It's built like a step fund. Yeah. And
Steve Hsu: That's another Chinese company. Right.
Zihan Wang: Yeah. Yeah, yeah, yeah, yeah, yeah. Yeah.
Steve Hsu: But so are you, are you mostly using tools produced by Chinese companies and open source?
Zihan Wang: I think this is mainly because my friends are using it, so whenever I have some questions I can ask them.
Steve Hsu: Got it. Got it. Okay, good. But, uh, so one of, I mean, one of the things that, this is now maybe totally irrelevant, but a year or two ago I was sort of, if you look at my tweets on XI, complaining that everything is dominated by. closed companies that don't release these tools to the academic community, then AI research is, the overall progress is gonna be slower because, you know, other people can't get involved. But it looks like the situation is much better now than it was a couple years ago.
Zihan Wang: Yeah. Yeah, yeah, definitely. I, I just think like open sourcing, is, like, I'm not sure, like, uh, there's a, some like game theory results, like when everyone tries to collaborate with each other, like the benefit of society is maximized, but like, when everyone tries to betray each other, like their own benefits are maximized, but the whole society's benefit is minimized.
I think there could just be like, maybe a little bit, a bunch of people, trying to open source. And, then like more and more people will open source because people will always give praise to those people who open source. And when they like just to hit several limits, the other people will just choose to open source because not open source.
It means that maybe they will make money, but they will not be praised and they, like scale, would be limited or something like that. So I, I think like, these days, like the machine learning community just, hits that threshold where like after that threshold more people will open source. Yeah.
Steve Hsu: I think you're right. I mean, I think, I think the trend is very strong, at least right now. I think Liang, I think even in the interview, one of the earlier interviews that you translated, you know, he makes a big point about this. Do you think they're very sincere, like a deep seq will keep open sourcing its models for many years to come?
Zihan Wang: I think so.
Steve Hsu: Yeah.
Zihan Wang: Yeah, I think so. Yeah, because, I think. Well, I'm not sure. Um, but like, based on my naive opinion, I think he doesn't want to make money. Yeah. Because he has made enough.
Steve Hsu: yeah, maybe he has enough money, but, uh, okay. But let me ask you, um, so what, you know, next week I'm gonna be in Silicon Valley, and I, I've known people in that industry for many, many years now. And the people that follow AI very closely, so investors, venture capitalists, even people who, you know, are CTOs or something, they're generally not very aware of what's happening in China.
So the DeepSeek thing was a little bit of a surprise to them. They don't know what Kimi is. They are what Gwen is. So, so they're, I think they're kind of sleeping on the general quality. The models coming out of China, which I think are actually quite high. I, I'm curious what you think about this.
Zihan Wang: Yeah. Yeah, I do believe that current Chinese,either companies or schools, they're building very fast. Yeah, I think fast is always some of the characteristics in China because, like there is a model that we have been taught since our programming school. Like, you must be very diligent.
Yeah. So this is just like some, some information that is like, like driven in our DNA. Yeah. So, so, so Chinese people always make things very fast and, I think like for the innovation, for example, for the optimizers, that drive like large training and for some important algorithms, I think this is just globally, for example, they can be produced or just, first discovered by us or any other, like, Europe, yeah, something like that.
And, I, I think, Chinese people always could. Detecting which algorithm is more promising and trying to scale it up. Something like that. So, so yeah. Yeah. Yeah. So this is one of the things that I discovered.
Steve Hsu: When it comes to more fundamental improvements, like say, actually going beyond the transformer architecture, or I think you already mentioned diffusion models. you see a point in the future, maybe the near future where actually the, the really unique or, or creative innovations are actually coming more from China than, uh, the US?
Zihan Wang: I think this is actually a good question. I have no confidence for any conclusions. I. Yeah, I, I, I, I don't have any confidence for any conclusions, because things are happening too fast and things are changing too fast, actually. Like I, I don't think the me like three months ago could ever predict like what kind of status I am right now.
I don't mean anything else. I just mean that like, maybe three years, like maybe three months ago, I'm working on my own project about structured reasoning about agents. That was a very small project and we have been building a very delicate algorithm on that. But after DeepSeek released his R-1 we just removed like 90% of the algorithm and found it worked for the region.
Yeah. So I don't think anything is predictable.
Steve Hsu: I
Zihan Wang: Yeah.
Steve Hsu: my, my priors from say three or six months ago are totally different from my priors now, so everything is, it's changing so fast, it's almost hard to keep track.
Zihan Wang: Yeah, yeah, yeah, yeah, yeah. And I think nobody can even predict what will happen in three months.
Steve Hsu: Yeah. So maybe I can get into, uh, some slight details about research. So you, you mentioned R-1 and R-L and so for the audience, I think one of the lessons that came from the R-1 paper from DeepSeek was that you could get very far by having a kind of reinforcement learning where you're giving the model very well-defined problems where there's definitely correct and incorrect answer. And in a kind of automated sense, the model is attempting to solve these problems. You're feeding back from what it does into adjustments of its internal parameters. And amazingly it's able to learn how to reason fast, very effectively from that kind of somewhat automated process. And I think that was a surprise to a lot of people I know from. Personal knowledge that a lot of the US labs were paying a lot of money to pay humans to solve problems and use that as fine tuning, training data, et cetera. But this RL method is more elegant and doesn't require as much human effort, uh, involved in the process. Um, so, um, I have a few questions since you're a real expert on this.
So, one of my hypotheses is that for a given model, a pre-trained model, it has a certain strength and then you put it through the RL process. It looks to me like all the curves I've seen show rapid improvement, but then there's some kind of asymptotic behavior where unless you make the original model stronger, you're gonna hit some max performance from rl. Is that a plausible interpretation of the data that you've seen?
Zihan Wang: So I'm not sure if I understand you correctly. You mean that like for it we have like an upper bond for the performance, but for.
Steve Hsu: As fun, yeah, as a function? No, as a function of the strength of the base model. There is some upper bound and, and you know, maybe asymptotically, you approach that upper bound. it, no matter how clever you are about the rl, you're, you're, you're probably still limited by some upper bound based on
Zihan Wang: This is definitely, this is definitely, I, I, I think this is very obvious.
For example, like if you have a, like I, I don't think this is like only constrained by data or model type catcher, we just talk about like model size for example. You just want to predict the weather and you have an accuracy threshold.
And it's definitely like the larger model, it can contain more information about every instance, for example, like the, I mean the weather conditioning every part of the region and then like they will calculate more efficiently. So I think definitely the performance of the model is constrained by a lot of things.
But I'm not sure whether it's constrained by RL or it's constrained by the model site itself. Maybe we can imagine that they have an infinite size model, or near infinite size model. I'm not sure whether RL will still, uh, make it a constraint here. Like, because, like in the, in the skating laws, like people always say that like, you would, you will always, you should always be clear of like, what is the constraint for now.
But I'm not sure like the current trend of RL training, his and upper bond is because of RL or any other factors.
Steve Hsu: Okay.
I mean, the reason why this is kind of a crucial question is because there is some feeling that for the pre-trained models, there might be a data bottleneck or, or something which prevents them from making the pre-trained model much better than GPT-4. So, for example, 4.5 is not really better than four, right?
Claude 3.7 is maybe only a little bit better than 3.5. So the question is, if you, there's some bottleneck for the pre-trained model. No matter how much RL you do, you're still gonna be limited. You can't get all the way to a GI or a SI without also passing that bottleneck on the pre-trained part of the model. Does that make sense?
Zihan Wang: Yeah, yeah, I understand your point. Um, but actually we need to know whether it is about. Model problem or it's about a data problem. because, I am definitely sure that GPT reads more than any of us throughout our lives, if that data account cannot make us understand the world. I'm not sure what kinds of data could be used to understand our world.
So.
Steve Hsu: Well, let me just be more precise.
So let's assume we're sticking to the transformer architecture, okay. Obviously there could be some innovation where we make it literally like our brain or something, but, let's suppose we stay within something fixed like a transformer architecture, then maybe those original scaling laws were true. And you do need a three x or 10 x in data to go to, a 10 x larger model, right? So under, in that scenario, there seems to be some. A bottleneck, like reasoning by itself, is not gonna get us all the way where we want to go. Right. I'm just curious what you think about that.
Zihan Wang: Yeah, yeah, yeah. So, I'm just talking about data and I think current data is definitely sufficient, but I'm not sure if current model size is sufficient enough. For example, we can train. On the same data, but with a different model. For example, if we just use a 10 times larger model and we, we could just find that, for example, after pre-training the 10 times larger models, like if, like, validation loss is larger than the smaller model.
Actually this always happens. For example, if I am training on a larger model, sometimes it is just, uh, uh, like a little bit smaller, uh, like a little bit slower to converge to the final loss trend. But, in that case, I'm not sure if RL will help even more because of the larger model. I think there's a theory that larger models tend to make the processing of the data more smoothly.
For example, like when you are trying to have a very small model, they're training on some strange data, then they definitely could, lead to some like overfeeding. But if you just increase the model size, still use the. Strain data and the model will, like, find some of the smooth transition between different data itself.
You can just increase the model size and the overfeeding issue will likely be lessened. So, yeah, I'm just curious. I'm also curious, but I think someone will help me answer this question, like maybe some of the researchers will help you answer this question. Like, for example, we know that data is just that much.
We do not have more data, but, if the model, if the base model could be larger, like could it, like just to raise the upper bond of RIL, than the current model? Yeah. I think this is definitely worth exploring, but this is definitely money burning. So, yeah.
I, I, I think like, so, so like current, there is definitely another trend of.
Uh, doing research is how to make your model more efficient. Like, for example, like in the same compute budget, like how to, or in the same money budget, how to make your model better. For example, like MOE for example, like MLA, those sort of kind of research are just, like moving toward this direction because we know that we are far from maximized efficiency and in order to like understand whether like current model, size or anything is a limit, we must, uh, um, in order to not pay that too much, we can, like scale up the model while doing a lot of research on efficiency.
And, yeah. So, I think these are just two different directions. For example, if we just do not train a larger model, but we experiment on efficiency for just a long time, for example, for five years and after five years, we find that efficiency has like a 1000 times of boost at that time.
The current large model is just so cheap to train. So at that time, we can just try to see if we can train a larger model to solve all of these problems. Yeah. I still remember very deeply that like I, the first time I saw Bert, I was so impressed by it, but also I'm so surprised that, okay, we, in order to train a model, we need to spend like millions of dollars.
But for now, I think that any lab can pre-train a birch based model. Like all of the improvements people have been doing on efficiency all over the years. I, I think it's just not too many years, I think seven years, something like that. But people, like, I think everyone can, maybe not everyone, but every major lab can pre-train a bird model without any too much cost.
Steve Hsu: Right. I mean, but you're, you're still talking about millions of dollars for the pre-training. Right.
Zihan Wang: So for now, pre-training a bird, I think it is 10 K to 100 K.
Steve Hsu: Okay. But, Bert's not maybe, for a, for a state of the art model, something that's as good as V three or GBT four, would at least take millions of dollars Right. To pre-train that
Zihan Wang: Yeah. Yeah. This is because I, I think this is because that people have been trying to scale up models and also trying to scale up the efficiency. So I think there is some balance, like people, a lot of people like, they feel the scaling up model is workable, so they're trying to make the model scale up.
And a lot other people are feeling like, um, efficiency could be much better to work on. And they work on efficiency. And finally, the budget all over the world achieves the balance between scaling up research and efficiency because like, yeah, go ahead.
Steve Hsu: at one extreme you have X, you have Elon's company, which you know, they have like whatever, a hundred thousand GPUs, H one hundreds, and they can just throw money at the problem they get a model, which is good, but it's not necessarily better than the model that DeepSeek train for 6 million of
Zihan Wang: Mm-hmm.
Steve Hsu: You have a pretty wide range of strategies here that people are executing
Zihan Wang: Yeah. Yeah, yeah. Yeah.
Steve Hsu: one of the arguments that I've had, both with people at the big labs and also with investors who invest in this space, is let's suppose we, we are not able to make, we. pre-trained model, which is significantly better than G PT four or V three. Can we still get to our goal of a GI or a SI, just by being better and better at RL and reasoning? And so that, this is a very important question because, because nobody knows how to push the pre-training one order of magnitude better, but, but people feel like, oh, we're still seeing these gains and reasoning, so maybe, maybe that's, maybe we don't have to worry about the pre-training bottleneck, reasoning is enough to get us to where we want to go, and I, I personally am skeptical about that, but I, I'm curious what you think. Yep.
Zihan Wang: Yeah. Yeah, yeah. I, I, I think this is just like. When you are playing a game, you have different features. For example, you can enhance your attack, you can enhance your defense, or, and you can also enhance your, like dodge or something. And it's just like when you have a bottleneck in a certain feature, you can try to focus on another feature.
So yeah, I, I think like, current IO is, is far from a bottleneck. So there it's definitely very, I think it's obvious when there's a lot of people trying to work on RL instead of scaling up these days because RL seems to be more workable than scaling up these days. Yeah, but I, I think when like RIL comes to a bottleneck, people will find a lot of other new things to work on, for example, like efficiency and also, and I think, I'm not sure about like what kind of, strength the model will be having at that time, but I believe like if at that time AI could help people do research.
Then people will definitely have a lot of new things to do. Yeah.
Steve Hsu: Yeah. So let me, uh, coming back to RL now, I don't know if you know this paper, uh, their acronym is LIMO. It's some researchers from Shanghai Ong University, and they claim they were able to develop very high level math capability, I think using Qwen 32 B, but only giving something like 900 or a thousand examples.
These were handcrafted examples, so, so they're made in collaboration between humans and big models. Only those thousand were used and they were able to bring this relatively small model to much state-of-the-art math capability. I
Zihan Wang: Mm-hmm.
Steve Hsu: Are you familiar with this paper?
Zihan Wang: I'm not familiar with the paper itself, but I think like it thoughts, could be similar with deep six R-1, where they use some CodeStar data, like it, it's also very small scale, but then after like making it like they can apply the model with RIL and then you can develop very good like math capabilities.
Steve Hsu: But in this case, the number of examples was so small. It was only like a thousand. And, the hypothesis, the bigger hypothesis these researchers had as a consequence of this result is that. These abilities for particular steps in the reasoning, the ability to do a particular step is already inherent, even in a fairly small model like Queen 32 B.
But it's just a matter of giving it the right example so that it knows how to proceed in the reasoning process.
Zihan Wang: Yeah. Yeah.
Steve Hsu: amount, a surprisingly small number of examples are enough to get it to fully utilize the capabilities that were already in the pre-trained model. And to me, this hypothesis is very plausible actually.
But it has a lot of implications, you know, because, because it means like even, even a very small group with very little budget can produce models that are really at the cutting edge of a narrow capability.
Zihan Wang: I think it's also very reasonable, and actually I'm not sure, maybe like, if your audiences know, more, like, know much about Chinese gca, where, like for the math problems, we just remember, try to remember about some basic knowledges, but like the, the final exam could be very difficult.
But it's just because we have been training a lot on some medium level or just difficult level tasks in our real life, for example, we try to learn something from how to organize this like different atoms of thoughts together to make some like connected thoughts. And we can try to summarize, from the connective thoughts and build, build a lot of conclusions about that.
Then like the first layer, second layer, third layer, and finally the got call problem could be very difficult, but we still have the chance to solve it. And I think recently there is another paper called Atom of Thoughts. Yeah, which is also very popular on, on acts. I, I haven't checked it in detail, but like, the core authors are just, also my like,university, like, schoolmates.
Yeah. So I, I, I think this just, they just claim that like using this sort of atom of thought, like any model can enhance its performance than like, previously people are using C-O-T-C-O-T, I think it's, it's more of like a natural flow, but not very structured thinking, but they're using structured thinking and they, somehow like develop some thought atoms and then try to connect them together to build a lot of like, fancy conclusions and they can try, finally try to solve these problems.
Yeah. I'm not sure if this could connect, but like, yeah, so I, I definitely, believe that like some of the, thinking patterns, some of the basic thinking patterns, for example, a very simple one, like to, to reflect could be like just to learn very simply, but, it depends on how you use it. You can use it for very delicate thinking patterns.
Like you can insert this as a function in your right. Delicate thinking patterns. But like the very, very, very basic assumption I think is just limited. And I think there's a chance that it can be contained in thousands of pieces of data.
Steve Hsu: You know, it's funny that usually people, when they talk about the Gau Cow, they just complain about how many years students have to prepare for it and they don't get to have a. much enjoyment when they're teenagers because the Gau cow is looming over them the whole time. But you're the first person now who's actually said like, Hey, there's a really good aspect of the Gau Cow because if, if you do manage to layer all these strategies together, you,
Zihan Wang: I, I think people complain because they do not get a chance to go to the university that they dream to be. Yeah. Once everyone could go to the university that they think, oh wow, it's good. I can choose the university. I'm happy about that. I think our cop is never under some pressure.
Steve Hsu: Okay. But Well, but there's also this stereotype that kids in, uh, South Korea and Japan and
Zihan Wang: Yeah. Yeah, yeah,
Steve Hsu: preparing for the gau, they miss out on parts of their childhood. Right. They don't have as much free time
Zihan Wang: yeah, yeah, yeah. I think this is not solely a problem with the GCO itself. I know GCO has some shortcomings, for example. It's like, um, you, you test it for once, but it could depend on your past life. I think this is definitely one of his shortcomings. but also I think this is more of like, um, social educational resource.
For example, only like in, in a province for example. Like there may be like 100 K people who try to go call each year, but only like top 100 or just like two top 200 of them will go to like picking and university. So people will be just so worried about that and they were just, uh, focusing too much on that.
So, I. I'm not sure how this could be solved because I, although I'm in Rein University, I'm not an expert in like, so like, so like society study or something like that. But I think, um, yeah, so like the girl called the test problem itself, I think it's funny. but maybe this is also because like I, I, I can enjoy it.
Maybe some people just cannot enjoy it. But yeah, I, I just, yeah. I have to say I learned a lot from it, although I know that like my high school has been a little bit frustrating because I have to do a lot of tasks every day. Yeah. So this is just a little bit critical about it.
Steve Hsu: So, uh, we've been talking a lot about reasoning and I think we, you and I both agree, there's still a lot of untapped potential. In reasoning and using RL to make the models better for reasoning. And obviously everybody in the world is working on this right now.
Um, I wanted to switch and talk a little bit about your paper on chain of experts.
Zihan Wang: Yeah.
Steve Hsu: So for the audience, one architecture of these models, which has turned out to be very efficient, is to have a mixture of experts. So within, instead of, instead of one giant dense model, you have different models that are slightly different in nature and there's a, a gating function or some kind of allocation function at the beginning where for a particular type of query, the query is routed to a particular expert or or subset of experts who try to answer it.
And, so not all of the connections are activated. Not all of the parameters are used in the quote thinking of the model. And so this is a more efficient way to do, uh, uh, large language model processing. And Han. His collaborators just recently wrote a paper where they did something interesting where I think the way a physicist might say it is you're making a kind of super position of experts, right?
So, there's a coefficient in your formulas, g sub i I think. And you're, you're mixing the experts, I guess, at every step of the, of the inference. So maybe just talk about what you guys did.
Zihan Wang: Yeah. Yeah, yeah, definitely. Um, so I think I can talk from the intuition of the project. So when I was thinking about a mixture of experts one day, I just thought that these are just kind of customer service, for example, like there is a token here and it's just like an issue and you would like it to be passed to some of the actors.
And then like, when they solve it, this can be closed. But I've been always thinking like, in the real world, like people do not just make separate experts handling this ticket and then close it. Instead, they will build up a chat group of the different experts and let them communicate with each other.
So at the beginning of my research, I was just believing that I could make it. Um, for example, if I can choose the experts first and then make the experts, to be having this token be processed for many times, and each time the token might be processed a little bit differently, but the experts, it's the same.
it's just like to, to handle the token, like for multiple times and each time, like different, experts are having, like, processing different parts of it, I, I was a little bit sure that it could work. and I do some experiments. Um, but finally I find that the code is a little bit hard to write.
Um, because like you would like to,to lock some experts and they just be used for, to process this token. And I find that the code is a bit hard to write and at that time I just found, I was just thinking if I could, not limit the expert that is used to process this token, but just to make the machine learn freely.
'cause we know that sometimes we humans just push too many constraints to the machine, but if we can open the constraints, maybe the machine could just be better. So I just moved, removed the constraints and finally found that the model is like learning very well and even better than not passing the experts.
So I'm, I, I think I can now formulate it, more of a, a union of like two different, information that I want to like, like, take as take aways for, for example, one of them is that the experts need to be processing that whole consequentially. So. Previously people have just been finding that, okay, the experts could be a person, token in parallel, and they can handle the token very well.
But for now, for example, a token could be passed to a group of experts, let's say group A, uh, at the first iteration and then to group B for the next iteration. And this could be effective because I think this could just enhance the effective layer of the MOE layer. For example, like for previous MOE research, the MOE layer is just one layer.
But we, we just find that, we, we just think that if we make it more of a sequential processing, we are actually making this MOE layer to be different layers. For example, the first iteration is expert group A to be processing the token. And then for the next iteration is expert group E.
And then just, just layer, stack. So, we believe that such communication could enhance the effective layer of the models. I think, previously some chain of thought research also pointed out that chain of thoughts is also trying to make this, language models trying to enhance its effective layer by making the tokens, trying to predict sequentially.
I think there are some relevant papers and a lot of relevant papers trying, theoretically, to prove that COT is effective. And I think this is something similar in, like COE, and we also use the chain of the name of chain of, yeah. And the other thing is like, I feel like in the COE paradigm, like we can enhance expert specialization.
Yeah. For example, if there's still an expert who is really good at handling several tokens, it has a chance to process the token many times in different iterations. For example, if there's always this expert trying to handle this token, but each time it processes the token, it actually processes the different status of the token.
For example, this is just like an issue. It is solved, a half solved first, and then okay, the expert sees okay, on how the expert itself and it is colleagues trying to solve this token and then like. Then the token is passed to this expert. And now it could seem like, okay, the token is half, uh, half processed and not, I, I can just process the second half of it.
but this all of, based on the assumption that this expert is really good at handling this token. so we haven't got too many, experimental evidence on that. But that, I think definitely this can be, somehow verified by just calculating, whether like the two different iterations, the experts are the same or not some, some of like the metrics and help us to interpret it, the experimental results.
So basically there are just the two assumptions, like I said, but I think we definitely need more. Experiments to verify them before like our next, more comprehensive, release or something. Yeah. I think there's also a side thing to talk about. I think people have been trying to get a small release first and then get a comprehensive release after that.
So, people have been building from journals to conferences and the archive and now this Twitter. Yeah. Um, yeah. Yeah. So I, yeah, I, I, I just believe that like we, I have been adopting the practice of having a very small, but a relatively comprehensive release at first, but, for all of these second releases, I'm still preparing so much for it.
So I, I just hope to like, resolve all of the questions people have been asking since my first release because they are really genuine feedback that can help me improve, like the paper or just like the project to learn, like what are people considering about it. For example, like some Twitter, like, uh, users that just, uh, have a lot of comments.
After, like, like in the comment section, I really learned a lot from it. So I think I will try to resolve every comment before my next release.
Steve Hsu: That's great. Well, that's a great way to do, I mean, it's like real time science where you, you're giving the seminar on X and you're getting a lot of good questions
Zihan Wang: Yeah, yeah, yeah. This is a good metaphor, I think.
Steve Hsu: So I, this is kind of a dumb question 'cause I'm sure you said this in your paper, but when you were just explaining it, I wasn't sure what the answer was. So you have these extra layers. Are you actually pre-training the whole model? Once you establish the chain of experts, architecture, then do you need to basically retrain the whole model because, uh, model you've got the layers, the layer connections probably depend right on, or probably should be changed in order that the experts do the right thing.
Zihan Wang: Yeah, we just train all models from scratch. Yeah. So this is why we are choosing like 0.5 billion models and even it's even an MOE model. So the activities parameters are even less so. I think this is the limitation of current, COE like method because it can,
Steve Hsu: With this style of research, you can show that there's a delta and improvement of the small model using this different architecture. But a skeptic would say, yeah, but what, what happens when it's a. billion parameter model. Like we, we wanna know what you know is, is it the same qualitative improvement or is it, is it better than what you saw, or is it smaller than what you saw?
So obviously there's no substitute for trying to do things at scale eventually.
Zihan Wang: Yeah. Yeah. So I believe another important topic that we will do next. Yeah. I know some of your audiences also like working on tech. Uh, so, so if any of them have the similar ideas, like I, I think they can feel free to let me know. I, I, I was happy to be taught in this way. Yeah. so I think one of them.
Definitely an important issue. Like the topic, we would do the next phase two transfer of knowledge for current MOE models into a COE partner. So we don't need to pre-train anymore. We can just, uh, leverage knowledge for currently pre-trained models. And that could definitely be a hard idea because I know that current, MOE models are trained, to maximize the efficiency pro for parallel so they can maximize like, so I, so for example, the experts can only handle this token for once, so they will maximize the information that it can do to like, to process this token.
But for COE, we definitely want the experts to process the toolkit for more times and each time they can communicate with each other. So, the objective is a little bit different. So I'm not sure how much knowledge we can transfer from the current MOE models to a COE partner, but I, I definitely think this could be something to do because, so first we don't have that much money to pre-chain a model from scratch.
And second is like, people would always want your method to be working on any kind of things like without, with, with as less assumption as possible. So the current assumption is that you need to initialize the model from scratch and try to train it. But when we make this assumption, like to be a little bit, better, uh, I mean, I mean like to be more widely applied, whether like we can leverage currently pre-trained model and trying to like transfer it to COE because we have been like proving that, training COE from scratch is useful.
But what about training COE from the ME model?
Steve Hsu: Yeah, I mean, so outside of the big labs, you know, like something that for them would not be such a big run, like maybe a few hundred thousand dollars or a million dollars. It's still a lot of money for an academic group to come up with that. Right. To, to, to actually do some fully pre-trained,
Zihan Wang: Yeah, yeah, yeah, yeah. It's harsh. I just calculate it. It is. It's like the annual salary of all PhD students. Our.
Steve Hsu: Yes. Yeah. I have a former colleague who was in theoretical physics, but now he does AI and he's at the Allen Institute, in Seattle, which is funded by the, the, the Money Foundation of Paul Allen, who was the co-founder of Microsoft a long time ago. And who's dead now, but, but, um, you know, they're, they're kind of in the middle where I think they, they have some resources that maybe a university group wouldn't have, and they're, they're actually trying to create like pretty much comp, almost competitive type models, but fully open source with even the d training data is open source.
So it is very, very admirable what they're trying to do.
Zihan Wang: Yeah, yeah, yeah. I learned a lot from them like O-L-M-O-E. Yeah, I, I, I actually like, we just, uh, got our price estimation because they open source the, like all of the things like GPU hours, so we can estimate it based on that. I think they're just so fantastic because they trying to open source anything, like anything they can open source, even like the one B logs, like experimental logs with a little bit, uh, with a lot of like metrics, which I believe like, like I'm not sure if they're the first to open source, but, actually I, I think they're the first to open source, a pre-trained models experimental logs.
Yeah. May I, I I, I, I know I can be wrong, but I, this is the first that I can see.
Steve Hsu: No, it's the, it's the only case I'm aware of. I don't think any of the others, even DeepSeek or, or meta, they don't, they don't give you that much. Right. So only, maybe, only the Allen Institute does.
Zihan Wang: Yeah, yeah, yeah, yeah. Yeah. I think this is just a. A huge benefit for the researchers because for researchers, they know what kind of parameters could work, but they just want to learn more from the detailed metrics that you use for each of your experiments. So, actually we are not currently open sourcing the one B, like it's in a mass for now, but we are trying to, um, like open source it later for like, all of our releases because we know that once we release it, it might be not that much helpful for, for like most of the audiences, but it could be helpful to those who really want to do research.
Steve Hsu: Yep. Good. Well, I told you we talked for about an hour and, uh, we're just right about an hour now. So let me start winding up a little bit. Um, let me ask you if you have any thoughts about how things are gonna play out over the next few years. Like, are there any non-obvious predictions that you wanna make or things that you think are gonna happen for sure.
And anything that, that maybe is surprising and anything you wanna say about the future?
Zihan Wang: Um, I'm not sure if AI could help accelerate people to go to the moon.
Steve Hsu: You're.
Zihan Wang: But I hope so. Yeah, I hope so. I hope so. So, I definitely think robotics could be one of the biggest improvements for the next few years because like previously all of the robots can just not do any semantic understanding.
But for current larger language models with vision capabilities, I think this is gonna be more true at. Yeah, I think this just raises a lot of the probability that we can see AI in the real world, for example, it's just like his humanoid AI and just, helps you to do a lot of things. For example, like household chores, something like that, because they really understand your language.
So previous ai, they pretend to understand your language, but it's like the fixed function, for example, you want it to do function A and function B, and these are just, predefined, when they're produced. But now if you can ask them to do a lot of things for you, they have the possibility. I know that like for current robotics AI, I think there is still a generalization problem where you, they're trained on task A, they can do a little bit well on task B, but not that much.
Well, so, if people can resolve this question, I think like, within several years, people will see robotics to be really like, like incorporated in your life. And after that is about. acceleration of research. So I've been posting privately on my WeChat like, I can't imagine that I can like, have two first authored, like a paper release.
I think it's released to be honest, um, in, in just one month. So I think research has been accelerated for today. so they are basically because that you can, get, your like inde, like the, get the necessary information for your project to, to like, so this process has been max, to be accelerated because like, so previously when people want to know about some knowledge, they can only, read the predefined documents.
But now people can really ask any of the ais and. Like say that, okay, I already know A and B, please interpret a C to me. And like everyone has different A and Bs, but AI can give the correct C for different audiences that have different A and Bs. So like the information, like, like obtaining the information is really getting fast.
But I think this is the acceleration for the current stage AI. And I think definitely like in 2025, I think it's just within this year, AI could help you to divide your code. I think it's very obvious because current AI can already help me debug my code, but at file level, so I can get some knowledge from AI in a single file.
But, for the repository level, debugging, I think it's not doing pretty well now, but for some cases it's doing well. But if. At the repository level, the AI can understand my research progress. For example, if I ask it a second time, I don't need to output anything, for, like the same as the first time.
I can just, uh, um, like, pretend that it's aware of anything, any progress that I'm working on my project. Then I can really have a great assistant that can help me do my project. And whenever I have a bug, I don't need to ask someone else to help me or, or just a like, have an afternoon just for this bug, but I can just ask AI to help me, like detect where the bug is and what kind of code I need to write.
And they just help me write 90% of the code and for the 10% of the code, they're not sure and they ask me. Um, so whether, uh, what kind of approach that I want to, uh, do with this and I can just, um, do the 10% of work. so I think this just, like the most, um, significant boost for now because at that time I, I, I think the.
I think someone has said that maybe it's Andrew Carpathy, that like, we only need to write code in natural language. We just need to tell them about our ideas. And we actually do not need to write code ourselves. We just need to be understanding the code that they write for us. And I think this is just another big part of the acceleration of research at that time.
And when, all of this, uh, like both of these parts are just, uh, merged together, I'm not really sure about what kind of research progress we'll have at that time. Yeah,
Steve Hsu: good. Yeah, I agree with everything
Zihan Wang: I.
Steve Hsu: said. I think we're right on the edge of being able to have AI understand our repositories quite well and then be able to say in natural language or, you know, very kind of natural pseudo code what we want and it builds it knowing what tools it has available in the repository.
I think we're very close to that in some settings. One thing I was just saying to my research group earlier today is like if you want a review article, there's some new area you're trying to understand and you want someone to write a review article of that new area, the AI will do it. And you can even, as you said, you say, I already know A and BC is what I'm trying to learn about.
Please use these articles as context and write me an introductory review article so I can understand it quickly. That is a thing which, you know, I would not have imagined that would've been possible a few years ago, but it's, it's totally possible now.
Zihan Wang: I would have never imagined that like one year ago.
Steve Hsu: Yeah. It's insane. Yeah. Great. Well, Zihan, I really appreciate this time. I'm sure my listeners will enjoy this conversation and, uh, thanks so much for joining me.
Zihan Wang: Yeah. Yeah. Yeah. Thank you so much. Actually, I really enjoyed chatting with you because like your questions just to like have me thinking a lot of things that I haven't been able to be thinking with in the past. Uh, like. Research life because like, you know, like research life is sometimes very inspiring, but most of the time it's just so boring.
I need to like to write a lot of things that, yeah, that just suck. Um, so I'm very happy to be chatting with you today and I really learned a lot of new perspectives.
Creators and Guests
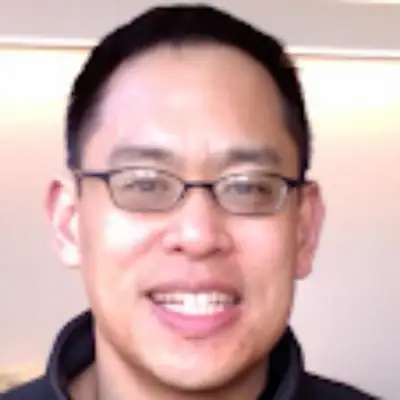