Raghuveer Parthasarathy: The Four Physical Principles — #11
Steve Hsu: Welcome to Manifold. My guest today is Raghu Parthasarathy. He is the Alec and Kay Keith Professor of Physics at the University of Oregon.
Professor Parthasarathy, who I will call Raghu I think because we are old friends, is a professor of biophysics and he is the author of a new popular book published by Princeton University Press. The title of that book is “So Simple a Beginning: How Four Physical Principles Shape Our Living World.” Welcome to the podcast, Raghu.
Raghu Parthasarathy: Thanks. Thanks a lot for having me.
Steve Hsu: It's great to have you on. People who are readers of my blog may know you from the comment section because you occasionally comment on my blog. You have your own blog, which I will put a link to in the show notes. I enjoyed having you as a colleague for many years before I moved from Oregon. And, I really enjoyed our physics department in Oregon. And I enjoyed almost essentially all of my colleagues there, but there were a few colleagues I always felt were super insightful and always missed having you around for discussions. And you're certainly one of those people.
Raghu Parthasarathy: Oh, thanks. Well, we miss you too.
Steve Hsu: Thanks. So what I thought I would do is go through a little bit of your intellectual history. So what were you like as a kid, when you first decided to study physics, did you first start to specialize in biophysics?
Raghu Parthasarathy: Yeah, yeah. This, it's an interesting question. I mean, I'm of course biased since I'd probably find my own history interesting. So I'm a biophysicist. So I look at things at the intersection of biology and physics, and I think like a lot of biophysicists came to this by a very sort of meandering path. And in fact, like, you know, despite the fact that I probably talk more to biologists these days than to physicists and really cut it out this edge, I actually never took biology in college or even high school. I think eighth grade was the last actual biology class I took. Even though now, you know, very much, much immersed in this.
And, and that's not, you know, hugely atypical for focuses. But anyway, to be more precise, when I was a kid, you know, I was always quite interested in science and math, but especially physical sciences. I was really into astronomy for a while and was very fond of math and like physics. And I think like a good fraction of us who end up being physicists, I had an extremely good high school physics class.
And one of the things that was, that was really great about it. It was very kind of rigorous, but we also did a lot of experiments, just actual hands-on things. And I remember distinctly one day being just blown away by something that seems really simple.
We did an experiment, you have a ball rolling down a ramp, then it rolls along a table. It goes off the edge of the table. And you have to predict where it is going to land. And you put a cup there and see if just the ball lands in the cup.
So, you know, very, in some sense, a very elementary first-year physics kinematics exercise. But the fact that you can actually predict this and you put the cup there and the ball landed in the cup, I found just kind of mind-boggling, that there's this actual power of quantitative prediction in the world.
So, you know, I didn't exactly know what I wanted to do, but I was interested in math and physics and that kind of stuff. And, I went to UC Berkeley as an undergrad. And actually for the majority of my time, there was a double major between physics and astrophysics.
So you know, it's very different from what I'm currently doing. And, I had the good fortune of being involved in an undergraduate project in the astronomy department to build a radio telescope. So a bunch of us, we put a huge amount of work into this, met, you know, weekends and designed and built this, this big metal bucket that ended up on the roof of the astronomy building.
And the thing actually worked. It detected the so-called 21 centimeter line. So these radio waves are put out by an atomic hydrogen. You could point this up at the sky, detect this, this hydrogen, you even determine this velocity and figure out, you know, the speed at which the galaxy is rotating. It was a lot of fun.
It was extremely educational. Very hard and really stimulating. But I began to get more and more interested in the fact that, okay, you could build this thing, it's just, you know, a meter wide or so, you can put it on your roof and the total amount of this radio wave energy at 21 centimeters, that's hitting the entire surface of the earth is about a hundred blocks. Well you can build something that actually detects that and turns it into a signal. So I got more interested in the backend of the telescope than the things we were pointing at. You know, even though I do think the things pointed out were interesting too.
So, you know, there still isn't biology. I got more interested in kind of the, the tangible in a way or materials, the end of physics and thinking of what can we build them and sense and stuff like that.
Steve Hsu: Sorry to interrupt. But you say the backend, do you mean you got interested in the electronics of signal processing or the...
Raghu Parthasarathy: Right. So, yeah, don't mean kind of in, in detail, the back end, like how exactly did the circuits work and so on, more just the fact that. Kind of the broad sense of like materials. What are the kinds of things that make this possible? How do we understand, you know, metals and semiconductors and this kind of stuff, tangible stuff that you kind of work with?
Does that help?
Steve Hsu: It does. Actually just another, just a technical question before we get into the more interesting stuff. So you, you said a hundred watts of energy in the 21 centimeter line is spread out over the whole surface of the earth?
Raghu Parthasarathy: That's what I recall. I guess I have to admit it's been a while since I looked up that statistic probably when I was an undergrad. But that's what I recall, a hundred watts for the whole, you know, hitting, hitting the surface of view of the earth.
Steve Hsu: If that's true, then your sensitivity is off the charts, right? Because you're
Raghu Parthasarathy: Yeah. Okay. I should actually, I should be a little careful with this story to see if that's really, really correct.
Steve Hsu: I don't, I don't recall enough to check you on whether that's true.
Raghu Parthasarathy: Let’s leave that as an exercise for your readers. They can put it in the comments if I'm wrong.
Steve Hsu: But I’m a little embarrassed because when I was, when I was doing astrophysics, like stuff, I probably could have estimated that on the back of an envelope pretty quickly. But right now, I'm a little bit at a loss.
But, okay, so this led you to be interested in properties of materials?
Raghu Parthasarathy: Yeah. I'd actually, even at the same time, kind of overlapping this. When I was an undergrad, I got involved in a research lab. This physics professor, Paul McEwen, is a wonderful person. Who's now at Cornell, doing things like nanostructures and nano electronics with various sorts. So I got a bit of a foot into the door of this, this end of physics as well.
And so, then I decided, you know, for grad school, I started, I wanted to go to grad school and continue in this sort of vein of, you know, nanostructures materials. As you know, the technical term like condensed matter physics stuff, that's stuff, how stuff works.
And so I ended up going to graduate school at the University of Chicago, which was a wonderful experience. And the bulk of what I did was again, actually not related to biology or, or about physics. I actually worked on a bunch of different projects, but the thing that ended up being like my dissertation was the rise of nanocrystals. So these little, couple of nanometers wide, a few [unclear] of a meter wide particles that are so small, it takes an appreciable amount of energy to put just one more electron onto them.
And so like electricity going through these things, isn't like a sort of continuous sea of stuff, but almost like a, a granular flow of the electrons blinking one to the other and knocking off the next one and making the next one go and so on.
So I worked on these sorts of systems and here again, there's sort of two parts to it. I began to get more interested in one aspect rather than the other. And the two parts in a way, one was these electronic properties and what could you do with them and why are they what they are and so on. But the other is something that actually comes up in my book a lot, a notion of self-assembly.
So the way we would make the arrays of these little nanocrystals, these six, six millimeter wide little particles, is that we would make these in a liquid solutions here. These particles are suspended, technically these little particles jiggling around in liquid, coated with some nice molecules that coat them on the surface. We'd put a drop of that liquid onto a surface that we, for example, powder and electrodes or something onto it. Now you just go get some coffee, come back half an hour later. And it's just assembled itself into this beautiful order array of nanocrystals in like a regular geometric lattice. So the thing puts itself together and there's this notion of self assembly going on that if you kind of understand the physical forces that drive interactions between stuff, you can have them build themselves into things you might want.
Steve Hsu: And you don't, you don't need to plant the kind of seed structure that the additional molecules or atoms that create onto it? Does it just naturally form this lattice?
Raghu Parthasarathy: Right, right. I mean, there are situations in which, yeah, you can plant a seed and that'll influence, you know, the further growth and so on. But there are plenty of situations. Like in fact, the one we were dealing with where you don't have to be just this spontaneous, you know, so called lowest energy state is the ordered one that you actually want.
So I got really kind of mesmerized by this and because of this and just other, just general reading and talking to people and going to seminars and stuff like that. actually a one side project, I held off on getting protein fibers to organize themselves into structure as I'm putting metals on them.
I began to think, okay, the self-assembly thing is really, really pretty amazing. So that might be a good thing to look into as a postdoc. And where are the really amazing examples of self assembled things? Well, it's in living stuff. So let me move, let me just make a big leap towards biophysics.
So I took a leap, ended up in the chemistry department at UC Berkeley. So back at Berkeley, in a group looking at the biophysics of membranes where basically the materials that make up cell membranes and asking you how proteins organized themselves in these membranes, how did the constituents, you know, form themselves to structures and stuff like that.
Raghu Parthasarathy: So that was, yeah, go ahead. Yeah.
Steve Hsu: Sorry to interrupt. It's a bit of a jump, right? Because doing solid state physics. I mean, you're looking at
Raghu Parthasarathy: Yeah.
Steve Hsu: irregular crystal structure and suddenly it sounded like for your postdoc you not only jumped to the chemistry department, but you're doing soft, condensed matter IE membrane.
So I'm just curious, was it because you apply, I mean, I guess you had to apply to that group after, in your last year of graduate school. And so there must've been something in your head that just said, well, I'm, I'm going to cast my net widely. And there are lots of different systems that I'm interested in studying. Is that how it happened? Or...
Raghu Parthasarathy: Yeah, that's definitely part of it. So yeah, I did decide to cast my net widely, add some personal constraints or of wanting to get to the Bay Area actually, but, but at least scientifically I did cast my net somewhat widely.
But also, you know, even though my own background wasn't exactly in this area like I was handing out at the beginning, this isn't that uncommon in biophysics. You know, there aren't a lot of people who have exactly, you know, a tailor-made background to exactly the thing they ended up studying. And in fact, even if they do it may not be a benefit to really have that being often, you know, very wide perspectives are good.
So I was really saying, okay, I've, I've developed these skills, these sort of general skills of doing experiments, analyzing stuff, coming up with experiments, stuff like that. Are you guys willing to take a chance on me and uh, and go for it?
I think it also helped that the group I joined was a quite new one. And so they were interested in people and willing to take risks. And this was Jay Groves who's in, who continues to be in the chemistry department at UC Berkeley.
Steve Hsu: I see.
Now, in my experience when physicists, who, well, people who are not originally in biophysics somehow migrate over to biophysics. There's some kind of background learning that either they already did and it motivated them to make the switch or they then have to quickly do it and
Raghu Parthasarathy: Yes, definitely.
Steve Hsu: They go back and they read Schrodinger's book, What is Life? And, start thinking about all kinds. Maybe, maybe if they didn't know statistical mechanics very well, they start with statistical mechanics or diffusion things like certain, certain aspects of physics that are ubiquitous in the biological world, but maybe they didn't study it before. And did something like that happen for you?
Raghu Parthasarathy: Oh, definitely. So, you know, I had been, you know, somewhat paying attention to just, you know, what's published in science and nature and stuff and going to a lot of random seminars and things like that, even when I was a grad student. But you know, especially once I made the [unclear], I decided, you know, I just really need to make sure I have the equivalent of a good undergraduate at least education in, in, in biology.
So lunchtime reading for the first six or nine months or whatever it was gigantic, I don't know if you knew the Albert's Molecular Biology of the Cell textbook. It's one of these. I think it's about 1100 pages or so, one of these doorstops. So I read it cover to cover and took notes, actually. I'm like, what, what seems, you know, what seems interesting, but also what seems kind of physically hokey that might be interesting to, to like, think about from a more you know, physicsy point of view. So I went through that entire thing. I also, like, sat in on an undergraduate immunology course.
So yes, I definitely felt that I should brush up on the things I wasn't aware of. And this is also a pretty common thing, like you were saying as well. For people going to biophysics, you know, whatever their background, there's a, there's something lacking. And it definitely takes some, some real concerted effort to, to address that.
Steve Hsu: One thing that I often find is that, you know, as a physicist, if you try to read through that book, like Molecular Biology of the Cell, you know, you, you immediately like, even on page one or page 10, you, you start asking questions like, wait a minute, how did they know this? Can you deduce these first principles?
And sometimes it's true. They don't really, they can't really deduce it from first principles, but sometimes there is some expert in the world of molecular biology who does know and has thought really super deeply about it from physical principles. But it may not be generally known to all the biologists who are making use of the knowledge.
And I don't know if you've encountered that kind of phenomenon.
Raghu Parthasarathy: Oh, definitely. So, yeah. Those two categories and things may not be known or they may be known, but not generally known. And then there's the third category, which is a little bit frustrating where they're known and not even that difficult to, to point out, but it's felt that that doesn't belong in an undergraduate biology textbook. requires, you know, an understanding of statistical mechanics or of you know, various sorts of things.
And it's, it's, you know, instead one takes throughout. Okay. We're just going to listen to these phenomena or facts or things that are there.
Steve Hsu: So at what point did you feel that you really were a card-carrying biophysicist?
I think even in the years that I was a postdoc, I mean, if you asked, I would have said I was biophysicist, to really kind of internalize that, I think it took even longer than that. Like, so I started here at the, on the faculty at the University of Oregon in 2006. And I continued to do things related to membranes and materials that make up membranes. But then especially after a few years, and this is actually becoming interesting story in itself, but I branched out into, can we think about the gut microbiome from a biophysical perspective, which is now like what my group completely does and it's, it's a lot of fun and really interesting.
Raghu Parthasarathy: And I think it's really, even though that's actually an extremely biological topic, that's not often thought about through the lens of biophysics. I feel like kind of really sketching out, okay, here's something where I'm embarking on something biophysical, but really, really new. Like not what ghosts talk loud, dead or anything like that.
To me, that is where I can really call myself a biophysicist.
Steve Hsu: Yeah. The gut microbiome is super trendy. I didn't realize that you could make progress on it from a physics point of view. That's really great.
Raghu Parthasarathy: Yeah. Yeah. It's, it's, it's a fascinating topic. It's a really messy topic, by the way. It's one in which there's a ton of stuff out there. There's a lot of stuff. That's almost, self-contradictory, there's a lot of stuff based on, you know, very indirect premises and very small, small and studies and it's, it's in some sense, a giant mess. But it's also really fascinating.
But it's interesting, you know, the, the reason that I at least feel, and this has actually been a really fruitful direction from our group, that there is that you can get from a physics perspective is in some sense, not, not hard to see, cause we have this thing inside us, this, this collection of trillions of microbes, this vast community of stuff. And we know just from correlational things that it does a lot of things related to things like health and disease. But almost everything that the field in general knows is from these beautiful techniques like DNA sequencing or RNA sequencing that have no information about things like the physical organization of the stuff.
I mean, to paint a picture that people might not want painted in their minds. A lot of what we know comes from taking fecal samples and then running that through, you know, DNA sequencing machine and figuring out like, what are the bacterial species there? What other genes are present? Stuff like that, which is very, very powerful.
But you know, it just is a pure sampling of stuff. It doesn't tell you what species are near each other, what things might be moving around, what things might be stationary, all the kind of physical information that, in any kind of macroscopic ecosystem we would say is really important to understanding, you know, how it works.
So our entry into this is sort of making that realization and then saying, okay, well, can we say something about spatial organization of gut microbes? So what my group has been doing is building microscopes that let us look inside the guts of zebrafish, which are a neat model of organisms that are pretty transparent at young ages. And actually looking at what is the physical structure of these systems. So it's been pretty fun.
Steve Hsu: Well, they already just sampled from different regions of the gut and just, yeah.
Raghu Parthasarathy: Yes. So people have done that. Not as much as you might think. Because it is actually quite challenging to do. But even when you do that, you have pretty coarse spatial resolution. You're not really getting that much of a sense of who's neighboring who, and things like getting a sense of like aggregation versus, you know, who's swimming around and stuff like that are, are hard even with, even with those approaches.
Steve Hsu: Right. I had read articles claiming that a pretty significant fraction of the calories that are extracted from food by your body, are actually used by the microbiome. So, if some qualitative state change happens in your microbiome, you could potentially either lose or gain weight. You know, in some microscopic way. Does that sound familiar to you?
Raghu Parthasarathy: I've, I've definitely heard that. I don't know enough to say anything kind of quantitative about that, but there's definitely a lot of, kind of processing of nutrients and generation of various metabolic molecules that these gut microbes do. And that seems to be important, not just to like overall levels of, you know, calories available and things like that, but also, you know, secreting things that work like neuro-transmitters and all kinds of things like that.
So this, this processing of food, I mean, people have known that gut microbes are involved in processing food for a long time, but like we're getting a better sense of like, there's a lot to that. And a lot of consequences of that, of that processing and this idea that the micros might be changing our steady state. You know, sort of set points. So things like metabolism. Yeah. That, I don't know enough to say anything intelligent about it. But it's a big deal and legitimately so.
Steve Hsu: Yes. You know, I think we could spend the whole podcast talking about that particular aspect of your research, but I want to spend time on your book, because it, it is, it was just recently published, right? So this is
Raghu Parthasarathy: Yeah. Just this part.
Steve Hsu: In a way you're on a virtual book tour now.
Raghu Parthasarathy: Yes,
Steve Hsu: So you got to, you have to, you have to pimp your books, so to speak.
Raghu Parthasarathy: Exactly.
Steve Hsu: So the book is called "So Simple a Beginning: How Four Physical Principles Shape Our Living World." The version that I have is a hardcover from Princeton, which is beautiful. I believe these are watercolor illustrations that you yourself made.
Raghu Parthasarathy: Yeah. Yeah. Some of them are markers and colored pencils, but yeah, they're all things that I made.
Steve Hsu: Yeah. So if, if you have a, if you're a visual person or you have a kid who is very visual, I highly recommend this book because I think it's fascinating. Once you start reading it and looking at the pictures, it's hard to put down.
So what I wanted to do is on the cover, it says four physical principles. So I imagine that the listeners would like to hear a little bit about it. We don't have to give away all the best parts of the book, but I'm sure they would love to hear what those four physical principles are. And then maybe at least a few examples.
Raghu Parthasarathy: Well, I should maybe first note that there isn't a sort of a canonical set of biophysical principles, you know, different biophysicists would probably pick different things. There's probably some overlap.
But anyway, like, you know, thinking about, and also teaching about a really wide range of biophysical topics, I felt that there were some motifs, especially the, these four that I've picked that are both really powerful, but also really elegant in organizing our thoughts.
And so the first is actually one that I mentioned already, this notion of self assembly. And this is basically the idea that the instructions for putting components together are encoded in the components themselves. And especially their physical properties. So this is one that isn't unique to biology. There's even giving you that example of nanocrystals earlier, but living things make use of this all the time and, you know, across all scales from molecules to cells to even tissues and organs.
So to give you an example, and this is one of the, that, actually having the introduction of the book itself and you can actually get the introduction from the publisher website. So check it out. You know, we, we take something like a soap bubble that everybody has, has blown a soap bubble, and, you know, you know, you blow a soap bubble and it's this beautiful sphere of soap foam floating around.
And, you know, that's physical shape. Nobody has to sit there and fashion it into a sphere. It just spontaneously puts itself into that shape. And it turns out that's because of these kinds of physical interactions and principles that it wants to minimize the surface area of that, of that bubble and the shape that does that, that has the smallest surface area for a given volume is [unclear].
Okay. You can say, okay, well, yeah, we all know that that's, that's a pretty simple one, but then you can keep going with your soap bubbles, and you can actually, you know, try this in your kitchen and blow soap bubbles so they're all coming together on like a wet cookie sheet or something like that. And if you have junctions between soap bubbles, you'll find that there's some kind of junctions that you can have. And some that you will never find.
Like you'll never find four felt soap bubbles that come together like an X. So we're like the boundaries are like an X kind of like, you know, corners in the Southwest United States. You'll just never see them. And it turns out that that's again, of a physical media to think that that arrangement, but having an X is not one that'll minimize the surface area of this thing.
Rather you'll get something that looks more like an H or like an H with bent arms, where you have like, you know, this is to illustrate not just audio, but kind of bent arms and like a line connecting them.
Steve Hsu: It is, for the reader. It is illustrated in the book. So.
Raghu Parthasarathy: Yes. Yes, it is.
Steve Hsu: If you can visualize what we're saying, you can look in the book.
Raghu Parthasarathy: Yes, yes, please do. Anyway, but the point being that there are cell arrangements that form and some arrangements that don't form. And so that's what you got from soap bubbles.
One thing that's really amazing though, is that people have looked at arrangements of cells and various contexts. For example, there are these [unclear] cells in the compound eye of flies, and you have this arrangement of four cells and you look at it and it looks exactly like the arrangement that four soap bubbles would have.
In other words, it's not an X it's like this bent H I was trying to. So you might think to yourself, hey, maybe the cells are just organizing themselves based on these physical interactions into this form. Well, and for research this has been kind of noted for a while. And then a couple of researchers went kind of beyond this and looked at Newton flies that have, you know, 1, 2, 3, 4, the normal five, six or seven photoreceptor cells.
And it turns out those have exactly the same arrangements that 1, 2, 3, 4, 5, 6 or seven soap bubbles would have. So it's like it's telling us that, nature doesn't have to specify the exact position of each of these cells through some complicated chemical encoding of a position of angle and so on, rather put these there and rely on physical interactions for these cells to assemble themselves at the property.
So this notion that, you know, nature can kind of place the ingredients there and they'll assemble themselves based on various sorts of physical interactions just comes up all the time and it's this kind of beautifully all pervasive and, and, kind of elegant motif that, that nature makes you soft.
So that's theme number one, self assembly.
Steve Hsu: Yeah. You know, you, you spend a fair amount of time later in the book on, I guess the general, the name of this journal subject is embryology, right?
Raghu Parthasarathy: Yeah.
Steve Hsu: You know, we all start with as one cell and yet, and, and the need for, you know, the DNA in each of our cells is the same. So how do individual cells in our body decide that they're going to become ice cells or bone cells or hair cells?
It's really a fascinating question. You know, you start with one primitive machine, but at which it keeps copying itself, but somehow there's differentiation in terms of purpose and rupture.
So I first learned about this, I believe in, from a paper that Alan Turing wrote where he studied the differential equations, I think for the diffusion of densities of, you know, certain molecules of chemicals that might create a kind of stable pattern. And from that pattern, the individual cells in the embryo could figure out whether they're, you know, in the ha in the part that's supposed to become the head or the spine.
And I'm curious whether that has advanced to the point where we really know, how, how the whole thing works?
Raghu Parthasarathy: I'll give you the shorter and longer answer. The shorter answer is yes, we've made a huge amount of progress on this and not so much in humans, but in things like the fruit fly, we can really make a quantitative, you know, predictive model of how these kind of patterns of chemical cues lead to, you know, different things being in different locations, the segments of the, of the fly forming, where they do, where they do, for example. And actually that it's great that you bring that up because that actually gets at another theme of biophysics and another theme in the book, which is this notion of, as I call it predictable randomness that, you know, fundamentally there is, kind of a randomness underlying everything.
And the most important manifestation of that is something many, but unfortunately not all physicists are aware of, Brownian motion. The idea that every little molecule is jiggling around and that's a manifestation of just temperature and thermal energy all around us. So everything is sort of jiggling around and has this randomness of its, of its physicians.
But superimposed on that randomness is kind of a statistical predictability. We can say on average where things are, or on average how a cloud of molecules, for example a cloud of signaling proteins that tell cells what to do. How that diffuses through a landscape and how an organism like an embryo can make use of that.
So what Turing did, which was just really prescient and just, know, well ahead of its time is, is realizing, if you have things that can signal to cells or whatever, and those are undergoing these random, but predictable processes of, of diffusing through, you can ask, okay, how did these what kind of patterns can you.
And he did this in a very kind of abstract way of just, you know, what are the general mathematical kinds of structure of these, these things. But now we actually know the identity is of a lot of these molecules that are diffusing around and dictating decisions by cells.
And in a lot of cases, we can actually use some interesting things, like fluorescent probes and stuff like that, we can actually watch them and really measure what their kind of fields are and so on. And so, especially for things like the fruit fly, which is this, you know, model organisms that we've learned a lot from.
You can actually write down, okay, what are the so-called reactions to fusion equations? How can I get things like, not just the front of the embryo being different from the back, but can A interacting with B and B interacting with C and C interacting with D giving me like stripes, for example.
And then I can actually measure those stripes and see, okay, these stripes are corresponding to this segmentation and so on. So really just within the last 10 or maybe 15 years, we have these actually quantitative measurable, predictable pictures of how things like early embryonic development work that are just extremely beautiful and just mind blowing as well.
Given that the underlying thing is, although it's somewhat predictable, it's still random. Are there understood methods of error correction that nature uses? Yes.
Raghu Parthasarathy: Yeah. I would say that's actually a topic of a lot of present day work, especially as Bill Bialek at Princeton has just done gorgeous stuff on this. So you can really ask, okay, given the numbers of these molecules and this randomness, how precise could nature be?
And you can actually phrase it as, you know, how many bits of information can be encoded by like the early embryo? And how does that define, you know, how sharp your boundaries of one segment or another can be?
And we're starting to actually be able to answer those questions and say, okay, given these molecules diffusing around and how many there are and stuff like that, this is the best you could do. This is the best thing that nature could possibly do. And here's how well, you know, an actual embryo does usually that's actually pretty close. So you could really get into the information, the information processing of things like embryogenesis in ways that I'm sure would have just blown Alan Turing's mind.
Steve Hsu: Yeah, but yeah, he, of course he had an incredible vision of foresight.
Raghu Parthasarathy: Of course. Yeah.
Steve Hsu: is how it might work.
I seem to recall a long time ago Bialek was studying to be the fly's eye. I actually, or at least the operation of some kind of eye. And I think he also showed from an information theoretical perspective, or even a purely physical perspective, it was close to optimal. Like it can detect I don't know if it's RI or flies, I can detect almost single photons.
Raghu Parthasarathy: Yeah. Yeah. There's actually a beautiful description of that. So Bialek has a graduate level biophysics textbook, called biophysics searching for principles. It's it's, you'd really like it. And it starts off with the question of the eye. What are the fundamental limits on its sensitivity, both from the physics of photons, but these information processing motifs as well. And yeah, it's true. We are close to optimal.
Steve Hsu: Yeah. the, the thing, I'm not sure this is your area, but something I've been kind of interested in is to what extent quantum coherence is actually used in biological systems. And to the extent that you think evolution is this super powerful optimizer that's been working for billions of years, you know, it may even start to make use, you know, it starts to make use of anything it can make use of right? To make the organism more fit. And so I guess there are some claims now that quantum coherence actually plays a role in certain functions of animals.
Raghu Parthasarathy: Yeah. So that's definitely not my area and I haven't paid too much attention. I mean, definitely the place where this clearly comes in is things like photosynthesis, where there is really, one of these quantum mechanics to think about sort of expectations propagating through these systems.
You know, I almost have the opposite view. the more I look into biophysics and all these different systems, it's really amazing what you can do with classical mechanics. How much, you know, just order and pattern and, and dynamics and stuff that, that uh, yeah, just sort of classical interactions can give you.
Steve Hsu: Well, I'm sure that
Raghu Parthasarathy: it.
We'll see.
Steve Hsu: I'm sure quantum coherence affects our, our, you know, special cases. I
Raghu Parthasarathy: Right? Yeah.
Steve Hsu: everything in all biological mechanisms are primarily classical, but I think there are at least a few cases. I think maybe navigation is kind of like a carrier pigeon or something.
Raghu Parthasarathy: Oh, actually sensing magnetic fields. That's it, that's a fascinating and a very difficult area to explore, but there've been some really nice things on like how, how animals including humans actually might be sensing magnetic fields.
Steve Hsu: Yeah, So I think that's a rapidly developing field.
Raghu Parthasarathy: Definitely. Yeah, no, no.
Steve Hsu: So coming back to the big principles that in your book one of them is regulatory circuits. Maybe you could just say a little bit about that.
Raghu Parthasarathy: Yeah. Yeah. So what I mean by this is within every cell, you know, you have these wet, squishy building blocks of DNA and proteins and all these sorts of things. And, you know, they assemble themselves into basically a computer. So circuits that can respond to their environment and make decisions.
For example, I'm a bacterium. I like digesting sugars, but I don't want to make my sugar digesting protein if there aren't any sugars there to digest. So how can I make these decisions? You know, it turns out you can make them just at the level of molecules of DNA and molecules that interact with DNA. So you can, it's kind of self assembly again, but self assembly of circuitry and decision-making and things like that.
So there's lots of beautiful examples of this. In fact, I was just continuing what I was talking about. You can have things in which, you know, there's, there's a, a gene. So one of the things I spent quite a while on the book is, you know, what is a gene and how can we like to have a good physical picture of them and stuff like that. For the moment I'll just say, you know, some stretch of DNA that's encoding Uh, let's just say some protein that will do something like the sugar digesting gene.
So you could have something where that stretch of DNA. It's not going to be read out because something is just physically sitting on it and blocking that readout. But you could have things where that thing that's blocking the DNA, perhaps binds to the sugar you're trying to digest. So if that sugar is present, it will bind to that blocking molecule that won't block the DNA, and the DNA will make the enzymes to digest the sugar. So you can have this kind of feedback or sensing of the environment to at the level of like molecules that these things can put themselves together into these like regulatory motifs.
Steve Hsu: I'm always amazed at you know, these molecular machines that nature has developed in biology. I mean, there's so many of them and I always think, ah, gee, could there be any human in the future that understands all of these things? It seems like you have to specialize and we are one particular set of mechanisms.
Yeah, go.
Raghu Parthasarathy: Well, so that's a really, that's a really interesting, that's a really interesting point or a really interesting question. So yes and no, because you could almost take the opposite approach. So this idea of regulatory circuits also comes back to this really fascinating puzzle that especially, you know, with the sequencing of the human genome, like really started to weigh on people. How many genes do you have? And, you know, we've sequenced the genome even before fully doing that. We've got the sense that it's actually not that many?
You know, as, as you well know, we have something like 20,000 protein coding genes. In other words, 20,000 distinct stretches of DNA among the, all the DNA that we have, that encode particular protein machines that do something.
So 20,000, you know, it's kind of a big number. But when you think about, you know, how complex we are and all the wonderful things we do, 20,000 doesn't seem like that big of an instruction set.
And what's more, it's the same 20,000 genes that are in like your skin cells or your neurons or your highs or whatever.
So.
Steve Hsu: Or in a worm or a fly.
Raghu Parthasarathy: Yeah. Yeah. Like many of them are very similar to, and many of those have, yeah, sort of similar, similar counts of genes. So how do you get this amazing and beautiful complexity from a relatively sparse instruction set and how do you make those instructions turn on in one cell and not in another and so on?
Well, one of the ideas is this principle of regulatory circuits that you don't need, like a gene for everything. You need ways of combining these genes into circuits that then do something. So you get this kind of combinatorial effect from just having a few pieces that then can interact with each other in different ways. Kind of like, you know, transistors in a computer chip, they're all more or less the same, but you are wired together in different ways to do different tasks.
Steve Hsu: Yeah. You know, when I was a kid and I was first learning about molecular biology and they said something like, oh, there are only these 20,000 genes. And I guess they didn't even really know the number at that time, but that they had some estimates and I think when the human genome project completed, they had a better estimate of maybe 20,000 or something. And they were surprised at how small. Maybe they thought it was a hundred thousand before.
ButI always thought this is crazy because surely the stuff that, you know, at the time they would get characterized as junk DNA had to be playing some kind of regulatory role, as to, you know, what was actually happening with the expression of the genes or how they interact or something.
And so, you know, when I actually got into the field of doing some stuff with DNA, I was sure that we would find that, you know, in our group we build these machine learning predictors of complex traits and, you know, it turns out that pretty good chunks of the DNA that are involved in being able to predict something like the height of an individual or something like that. They're not in coding regions, they're outside of coding regions. So, and so that idea that there is actually much more complexity than just the specific proteins that are coded for it turns out that we realized, I think most businesses would have guessed that actually just, just from this a sense of the numbers that
Raghu Parthasarathy: I completely agree. Yeah. I've never understood why this notion of like large swaths of junk DNA ever made it anywhere.
Steve Hsu: Yeah, but it was, it was really the predominant view for a long time.
Raghu Parthasarathy: Oh, I agree. Yeah. Yeah. I don't know why, but yeah. You're right. It definitely was.
Steve Hsu: At one point I met James Watson and I asked him about this and, and, and there was
the central dogma. So I, so you know, this question of whether the central dogma really could be the whole story just seemed to me, even as a kid, very implausible.
Steve Hsu: And I think, and I think now it's understood that there's much more going on than just that.
Raghu Parthasarathy: Oh, definitely. But you know, it's interesting though, that I still feel that people really, both in the general public and even to some extent among scientists, really cling to a notion that, okay, there's, there's one gene and it has one effect that we can really kind of make a one-to-one mapping between like genes and phenotypes. And, you know, every biologist or metaphysics knows that that's not true, but it still, I think, influences the kind of intuition people have about a lot of things.
I mean, going all the way to, you know, the notion of thinking about, you know, genes and these, these regulatory circuits and stuff, you know, these are dynamical systems, but we don't introduce like undergraduates to this, for example. Even though it's really central to how these things work, how these things work.
Steve Hsu: Yeah, I would say I've encountered firsthand being one of the people that work on polygenic prediction. Or I should even say not even polygenic, but poly low-sci prediction of complex traits that, yeah, it was very counterintuitive to the vast majority of biologists that the story turns out to be what I think most physicists would have guessed, which is that, you know, they're large numbers of switches controlling all of these complex phenotype.
Raghu Parthasarathy: Yeah. Yeah. So it's actually, for that reason, I, even though this is, these are things that you work on that are actually quite far from what I, what my actual research is, but, but I thought it was really important to actually have a chapter in the book on like polygenic traits and what we can, and can't tell about them and things like that.
And I think this theme that I mentioned before is like predictable randomness. It's an important one to try to get, you know, the public in general to have an appreciation for. Because you know, it really comes to things like what can and can't we predict, how does this scale with the number of measurements we might be able to make of something, you know, whether it's a population we're sampling or embryos we're sampling or things like that.
And I think it actually is. Yeah, it's not just elegant and beautiful. It's actually practically important as well.
Steve Hsu: Yeah, absolutely. And, and it'll become even more, you know, to take one very newsworthy kind of case of gene editing. You know, if, if you think that. We have good gene editing tools And there's just one gene that controls something, I care a lot about, that seems pretty straightforward to make that edit and get what I want.
But on the other hand, if you realize, no, I might have to edit a hundred or a thousand differences. I have a very different intuition about what's going to happen if this technology becomes widely used.
Raghu Parthasarathy: Exactly and yeah, I think I totally agree. And you know, there's really not a good sense for many people up the fact that these are a very kind of profound, even qualitative difference between something like cystic fibrosis, where it really is one gene and one phenotype, you know, you have a bad cystic fibrosis transporter gene versus a really profound difference between that and some trait like height, where there are, you know, thousands or even thousands of genes that can influence touch rate.
Steve Hsu: Yes. So I think, you know, in my own work, it's getting to the point where it, and this is actually a common story that's repeating in all sorts of different areas of science, where it's becoming a story of machine learning, where gather the data, but then just make sense of it you have to point a machine at it. You have to point a computer at it, and the computer makes sense of it. But then the computer doesn't generate a story, a narrative that the human brain can really latch onto. The machine learning results are something like, oh, these 6,000 different places scattered all over your chromosomes are the ones that are, in some really complicated way, controlling what your diabetes risk is.
Raghu Parthasarathy: Yeah. Yeah. This brings up a really interesting, almost philosophical question of like, to what extent should we care about having the mechanistic picture or the narrative? Or should we just say, okay, well we can do prediction. That's fine. And sufficient.
That's actually a tough question to answer.
Steve Hsu: It is a tough question. But the question is replicated all over science. So like, you know, you hear about them coming up with better ways to control plasma during fusion. And you find that, oh my God, that, but what they're doing is using some machine learning algorithm that they train to control the plasma or something.
Or even the AI that's going to drive your car for you. Right? They don't really fully know how it works, but it's been trained to work, right? So they can make some statistical statements about how well it works. But it's, it's different from having a kind of causal narrative story that the human brain can understand.
Raghu Parthasarathy: Right, right. You know, this leads to other kinds of interesting questions. You know, having the causal narrative story, is it just something that we like, because it makes us feel better as humans? Or is it that, you know, having that causal story is what enables the deeper advances that are beyond kind of predicting from the immediate data set?
You know, one thing I wonder, for example, coming back, actually to the example I was giving at the very beginning from my high school class of like predicting, you know, where a ball rolling down a ramp predicting where I would land at putting a cup there. You know, you could do that by just making, doing the experiment a whole bunch of times and making a table of where on the ramp I start and where on the floor the ball ends. And just having some purely predictive phenomenological mapping between my starting point and ending. And, you know, that could work really well.
But then you could say, okay, actually knowing, you know, Newton's laws and having a deeper sense that gets us beyond the classroom demonstration to making, you know, a spaceship that goes to the moon.
So, you know, you can argue.
Steve Hsu: I totally agree with you. I think it's worth a lot of investment in human time and energy to get to that simpler causal story, as opposed to just accepting some very competent predictor whose guts you don't really understand.
And when you, when you can get to that simple, kind of traditional physics model of what's happening, then it is super powerful when you can get. Now it may be that for say understanding the human brain, there is just no such thing, right? That's such a complex thing going on that there's no simple description. And even the simplest cartoon description involves 10,000 variables or something. So...
Raghu Parthasarathy: Yeah.
Steve Hsu: You know, I think we're just going to confront some aspects of science, where the latter is true. And in that case, we're going to rely on computers basically to, you know, to give us predictive power.
Raghu Parthasarathy: It's an interesting time.
Steve Hsu: Yeah, no, It's a great time. I think there's just an incredible amount of stuff happening in science, which I want to discuss with you more broadly. But let's talk about the fourth physical principle, which plays a role in your book, which is scaling. And then we can broaden our horizons and talk about other things.
Raghu Parthasarathy: Sure. Yeah. So, scaling is just this idea that physical forces depend on size and shape and they do so in ways that determine, you know, what forums living things can have. And, you know, this, this is perhaps the most intuitively familiar of all of these, that these themes. We know for example, that, you know, little water Striders can walk on water, but you can't. Or the big animals have disproportionately thick bones compared to little animals.
So there's this connection between the physical forces of size and shape. And this is in some sense, a really old observation, like actually Galileo wrote some beautiful things about the necessity of big animals having big bones, but really kind of appreciating that. It makes a lot of things in the living world make a lot of sense and exposes kinds of these hidden unities between stuff.
So, you know, for like walking on water, for example, ignoring any, you know, theological implications. You know, forces like gravity pulling us down and the force of surface tension. This is the property of all liquids that can keep things from deforming or breaking their surface. Both of those depend on the size of the object. That's like being out of water in different ways. And it turns out that the bigger you get, the force of gravity, you know, is disproportionately pulling you down more than the force of tension for surface tension is pulling you up. So there'll be some size above which you just can't support yourself from surface tension anymore. And so, you know, if you try it, you just sink in the pool, but the little water Strider happily stays on. So the scaling thing just comes up in all kinds of stuff.
Steve Hsu: On the subject of scaling, is it true that all life on earth has cells of approximately the same size?
Raghu Parthasarathy: Oh, no, definitely not. So there's a pretty big well, the extremes have a lot of variation. In fact, it actually just very recently highlighted that there's still a lot of cool stuff in the world too, to uncover these bacterial cells were discovered. Bacteria were discovered that have cells that are like millimeters in size breaking the previous record, which was already, I think, around a millimeter or so.
So there is a huge variety. It's true that the majority, you know, most bacteria are archaea around the micrometer, a millionth of a meter around most cells in you are about 10 times wider than that. But there's not that much of a uniformity.
But this notion of scaling does get to a lot of things that are kind of mysterious and actually quite controversial. So there are these claims that things like metabolism should scale with size in kind of predictable ways. In other words, little creatures like mice have a much faster metabolism. They consume much more energy per day than big creatures like elephants.
There's this next quote from, I think [unclear] that an elephant-sized pile of mice consumes 20 times the power of an elephant. So observation for a long time and people have tried to come up with just like I was stating for the walking on thing, what are the physical forces that would lead to that kind of scaling? And I haven't come up with anything that has managed to convince everyone of like, what is the origin of this?
Steve Hsu: I seem to recall maybe these results, maybe they're not original to Geoffrey West, but so there's a particle physicist, particle theorist named Geoff West who became a biophysicist kind of as a network scaling kind of physicist. And he proposed, I think, something related to what you're describing. This would have been in the late eighties, early nineties, and I thought his model mechanistic model, for the reason for these particular scaling laws, had to do with the circulatory system.
Raghu Parthasarathy: It did. So he's proposed that, or he and coworkers have proposed that this particular scaling of metabolism is a consequence of basically the fractal geometry of like circulatory and other networks, the way pipes basically branch to other branches and other branches and so on. Which actually is the cover illustration of my book, that explains this [unclear] scaling law.
So that made a huge splash when it came out. But then a lot of people were very critical of it and pointed out some flaws both in the analysis and potential flaws in the assumptions. And then other people put forth competing theories that similarly got poked out by others. So if you look at any one of these, it does look like an explanation. If you look at the ensemble, it's actually kind of a mess.
Steve Hsu: Yeah, I had heard that the whole thing was not, it was still somewhat controversial and not settled.
Raghu Parthasarathy: Yes. That's a good way to put it.
Steve Hsu: At least in the early versions of this, like, okay, you could say like there's some kind of self-similar structure of the circulatory system, which is in, in, in a way the same in a mouse as in me, and maybe the terminal sort of minimal size of the smallest capillary diameter of the circulatory system is limited by the cell. And then maybe if there isn't that much variation in the cell size, then you can get some scaling relations from that. And that's, that's my big recollection of the whole thing related to the cell size I asked you about.
Raghu Parthasarathy: Oh, yeah. Yeah. So it's really fascinating to think about, but he tells me one of these things where I mean, to, to their credit or even to the credit of the whole field, it's a tricky thing to try to prove in any case. Cause it's, it's hard to really test these models and really say, okay, how valid are the assumptions that go into them?
You know, when I finished writing about this in the book, it did make me realize, I am an experimentalist and at the end of the day, I can go into the lab and see, you know, what, what, can we actually build and test and do. So it's hard to test these notions of ER things set by the size of the smallest node or its metabolism related to flow through pipes. And that kind of like.
I should say that. You know, even though it's hard to test these, that may not always be true in the future. So one of the things that's been a really amazing development in kind of biotechnology and biophysics class engineering in general has been what are known as organoids. Or also like, you know, organs in a vat or, or artificial organs, basically.
So things in which you can kind of seed in like stem cells [unclear], you know, a pancreas or a, an eye or those sorts of things. And they really do start to put themselves together to things that are really beginning to mimic, you know, proper organs, and so on.
And, you know, that's a fantastic and fascinating field in itself. But I also speculate, I wonder if these things are also going to be a way of addressing some of these hard to answer scaling questions. Because you might actually have in a lab system organs with different size and shape with different self assembled vasculatures, and so on that might actually let you measure relationships between metabolism and structure in ways that would be just impossible in purely natural systems.
Steve Hsu: Yeah. I think if you have these things growing, you know, on your bench, you can probably make some measurements that a pin down, you know, what limiting factors are probably better than you could just by observing an adult mouse or something like that.
Raghu Parthasarathy: Yeah. Yeah. I don't think anybody has done that. But I think that would be a real development probably in the next decade or so.
Steve Hsu: Yeah. So I think we've given the listener a kind of rough idea of what they're going to encounter when they buy your book. Which I hope they do.
Raghu Parthasarathy: It's fine with me if they check it out from the library too.
Steve Hsu: Yeah, that's good enough. I'm curious, what, when you, what was your decision like to write the book and who were you really envisioning reading the book? Is it a bright high school kid? Is it a scientist from another discipline? What, what were you thinking?
Raghu Parthasarathy: Yeah, that's a great question. So I really do want to reach a wide audience, like as much of the general public as I can. So I intentionally didn't write this. I mean, I think scientists will enjoy it, but I didn't write it with scientists as the target audience. I also didn't write it as a textbook, so there aren't exercises or equations or things like that.
I think it would actually be something that I hope high school kids would enjoy, especially to get a sense of what's actually out there in contemporary science, which is often very different from what you hear, you know, you know, you know, in a class.
But really I'm, I'm hoping for just sort of the educated lay public that might be interested in only the beauty of the biophysical perspective provides, and you know, how you look at the world, but also, you know, the intersections between this and things that are really quite practically important, like gene editing and growing organs in a vat and stuff like that.
So, , some of this, and especially some of my thinking about how to present things like this came out of actually a class that I designed a biophysics for non-science majors class, that I designed and taught, I think back in 2010 or 11. And I have taught a few times, and that's one of these courses that are for non-science majors here at the University of Oregon. It just satisfies the general, general science requirement.
And, you know, we have a lot of these courses and I've actually been involved with various programs. Like we have a so-called science literacy program that's really trying to, it has been trying to like, re envision how we do this sort of education. And I thought that was my kind of first dive into how can I convey this kind of thing to a non-science audience?
And it was a really good one because it also really got at the question, what do people find interesting and what do, what are the things that I find interesting that really most people don't, which is, you know, sometimes a little bit depressing, but it's a very kind of eye-opening exercise.
Steve Hsu: Yeah, I can imagine, like, you know, a kid who's been told, you know, starting in fourth grade that this is a DNA molecule. You know, by the end of it, they're just like, okay, so that's a DNA molecule. What do I care about? Right. Like if it looked neat, if it looked differently, how would it, would it affect my life? Right. So you can imagine kids losing interest in the kinds of things that would fascinate you and me.
Raghu Parthasarathy: Yeah, exactly.
Steve Hsu: No, I think you're well, when I left Eugene, you had one kid, I think who's about the same age as my kids. And so it must be nearing the end of his high school career. Is that, is that right.
Raghu Parthasarathy: That's correct. Yeah. So I have two, in fact, yeah, you're exactly right. That the older one is, yeah. I believe you are the same age as yours. So he's in 11th grade at the moment. And the younger one is in seventh.
Steve Hsu: Yes. So I'm just amazed at how much I don't want to call it busy work. Some of it's actually good learning work, but there's just so much stuff that they have to always be doing for their classes. And don't remember having to do that much stuff in high school. And, you know, so if I had found your book in the library, I would have just loved to like, know, go home and lie down. We'll read your book. But it seems like my kids don't have time for anything like that these days.
Raghu Parthasarathy: So, this is interesting because a lot of people tell me that I don't know if it's a Eugene thing or what, but I feel like there's less, less work in high school here than a lot of people in other places are telling me, which I'm not, which I think is a good thing for kids who do have, you know, self motivation general curiosity to do stuff. I do. Yeah, it does seem like a general trend that if there is sort of more busy work and kind of, less thoughtful work in a way, at least there's some reasonable regional variation to it.
One of the things that I've been a bit struck by, with his education or like, you know, high school is how, you know, he's taken, he, unlike me, he took biology in high school. It's interesting how non contemporary it is. And you know, how it really does seem like a lot of the biology that would have been taught, you know, decades ago without kind of merging it with advances in technology or physics or anything like that. So that's been a little bit sad to see actually. Not terribly shocking, but sad to see.
Steve Hsu: Yeah. You know, my son is sticking with AP biology right now, and there's just a tremendous amount of junk that they have to memorize. It reminds me of this story of Feinman going to the library and asking for a map of the cat. You know, he had to memorize the anatomy of a cat. So he goes to ask the librarian at Princeton, do you have a map of the cat? And you know, it just seems strange that they have to memorize all this stuff. When you know, now we're in an age where you could look it up on your phone or on your laptop, you know, if you needed to know the exact, you know, detail for some reason.
Raghu Parthasarathy: Exactly. I completely agree. And it's, it's, it's really unfortunate that. I mean, there's, there's flaws with physics education as well, but it's really unfortunate that biology education still is, largely about memorizing things and not introducing these things. Like, you know, let's think about these as, you know, dynamical systems and let's actually show that, you know, math is actually useful in these, in these contexts.
Steve Hsu: Yeah, I think it's just because maybe the, the, the teachers that are teaching AP biology, aren't, they're not used to thinking of it this way. They're not biophysicists right away. So, yeah.
Raghu Parthasarathy: Yeah, yeah, yeah. There are efforts here and there, like, I mean, I'm obviously more familiar with university-level things, but there are efforts here or there too, to change that. There was a paper actually like an education paper that just came out by a group at UCLA that has been making a freshmen level, you know, biology has dynamical systems course, which looks, you know, quite nice actually.
But you know, it'll be while if ever, if this really permeates, education as a whole.
Steve Hsu: Yeah. You know, I also think it's true that, you know, for us, after having gone through an entire physics education then we can appreciate oh yes, yes, let's think of the capillary system as some self-similar branching network. Or yes, let's think about this dynamical system. Like the word dynamical system doesn't mean much to most people, even to some engineers, they wouldn't know what you meant by a dynamical system.
So, yeah. So of course we have the luxury of already being exquisitely well-trained in something else then like, thinking about juicy things from biology, but these other people don't have that luxury.
Raghu Parthasarathy: Yeah. Yeah. And how you, how you bridge that gap is a challenging question.
Steve Hsu: if the kid hasn't studied the Newtonian trajectory of the ball falling off the table, analogy to that doesn't mean anything to him. Right. So it's, it's almost like you're gonna make him learn all the other stuff first and then, and then learn some biology, right?
Raghu Parthasarathy: Well, but you can imagine a world in which, you know, the teaching of biology itself includes. Okay. Let's think about how to describe something like a feedback loop, for example. and you know, if you're, if you are learning calculus in high school, which hopefully people do you know, let's have that as, as examples of how we can think of the reactions that govern a cell working.
And even if they're simplified examples, it introduces the idea that yes, these things are actually amenable to quantitative descriptions, which I think is really alien to a lot of education.
Steve Hsu: It is, I, you know, one of the analogies I've used with my kids since they were little, just to teach them about, you know, geometric progressions. And then later on exponentials are just like the story of an amoeba, the amoeba getting there and it splits. And then each of them splits. And then, well, how many members do you have? Six hours later, like that was always like a very fun thing to discuss with my kids. Uh, maybe we didn't find it that fun, but I found it fun.
Raghu Parthasarathy: So I'm curious actually, with your kids in their biology class, you know, they learn about DNA. Did they learn about how DNA sequencing works?
Steve Hsu: I have to ask. I don't know the answer to that. You know the other problem is when they become, maybe this is not the case for you and your son, but you know, when they get to a certain age, they don't really want to talk to dad that much.
Raghu Parthasarathy: Oh, totally. Yeah. I agree with that. Yeah. It's getting information. Volunteering is definitely hard. I asked this as a specific question. So I got an answer. I'd be curious what the answer is for you, because from Anya, the answer was no. You know, one of the, you know, biotechnologically just stunning things of the past couple of decades. The fact that not only can we sequence the human genome, but the cost has gone from $3 billion a genome to like a few hundred dollars. Thanks to, you know, our ability to think of this as a physical object that we can do things to, you know, if that doesn't come up at all in high school biology class, which is really smart.
Steve Hsu: Well, you put your finger on the exact thing that finally got me into biology. You know, a physicist, the fact that all that data was becoming available, you know, one thing I will say is that I've looked at my kids' textbooks and the textbooks are much better than when we were kids. And so I wouldn't be surprised if tucked into some corner of one of the chapters is a very beautiful explanation of how gene sequencing or at least some genes, a particular gene sequencing technology works. But you know, unless the teacher really makes them, you know, absorb that and test them on it. I can easily imagine the kid not reading it. So...
Raghu Parthasarathy: Right, right. Yeah.
I should. I should add just in fairness cause you know, people might get the impression we are dumping on biologists, but I often find that physicists are also completely ignorant of, you know, the amazing development of gene sequencing and what's happened in the last couple of decades.
Steve Hsu: Absolutely. Yeah. I mean, a lot of my physics colleagues think it's bizarre that I got interested in this, but I keep explaining to them , now, we have to pay $10 billion in a decade to get some new data at the LHC. um, whereas, you know, this is something that the DNA stuff we learned about already in high school, but apart from trying to crack the code of how do you relate the actual sequence of DNA to the properties of the organism.
But now we're actually positioning all that.
Raghu Parthasarathy: Right, right. Well that, but also just the fact that, you know, we, we made this enormous advance and this, you know, factor of, you know, 10 million in the decline and sequencing costs, not by somebody figuring out some, you know, complex biochemical reaction, but by making really interesting physical tools that push strands of DNA through gels or detect the charge from adding one nucleotide to the end of the growing straps, they're very actually physical things made all this possible. I mean, it's really kind of a triumph of sort of physics broadly defined that we can do all this.
Steve Hsu: is, is a triumph of physics and some aspects of chemistry
Raghu Parthasarathy: Yeah. Yeah. And it's not well-known at all. We don't teach our undergrads about it.
Steve Hsu: Yeah. In some gene sequencing technologies lasers play an important role as well, so.
Raghu Parthasarathy: Oh, yeah, definitely. Definitely. And I mean, I'm sure you were about to very new Nanopore techniques and you're putting a single DNA molecule through a pore and measuring just the changes in conductance from this thing just blocking ions from going through the most just extraordinary that anybody could get this to work and yet a test.
Steve Hsu: It's probably arguably the most impactful nanoscience right. That
Raghu Parthasarathy: Oh yes. Yeah. I would agree. Yeah.
Steve Hsu: Maybe you could argue Photoville photovoltaics or some other things are also super impactful now science, but, but definitely gene sequencing is as up there.
Raghu Parthasarathy: It's up there.
Steve Hsu: Yeah. Hey, let me, let me broaden out a little bit, to science in general. and one thing I want to ask you, you know, I'm a theorist so I know nothing about running an experimental lab. I'm just curious what it's like for you. How much of your time is spent, you know, on grants and managing your lab and you know, how does, how do you divide your time?
I mean, it's definitely the case that the fraction of time I spend doing what I would call actual sciences is definitely the minority of my time. You know, a lot of course it goes to teaching, but then even with other things, just administrative things, working on grant proposals, just talking to students which I actually really enjoy it, but on, just sort of logistics of how do we get the stuff ordered and then this piece of equipment breaking down and things like that, is definitely the majority of stuff.
Raghu Parthasarathy: So, you know, I don't have a great sense of how that differs from, you know, what it was, yeah, 20 or 50 years ago and stuff, but it is sometimes discouraging though. The relatively small fraction of time that gets spent on actually doing science. I try to occasionally do some stuff in the lab, which is just much less than I would, because I do actually really enjoy it.
On the one hand, so in my own career, I started out as a theorist, who would, you know, a lot of my papers are single author. So I would be sitting there working on something in my head, you know, in my office or something for long periods of time. But over time, and as I've gotten into genomics related stuff, I tend to have a bigger and bigger team. And I find that managing the team, getting them organized to be productive and efficient. That's very similar to my experience with tech startups. it is its own thing. I don't mind that so much. What I do mind is for the first time I'm submitting a grant to the NIH and that is like one of the most painful things I've ever done.
Raghu Parthasarathy: Oh, yes. Oh, I totally agree.
Steve Hsu: Yeah. And I'm just curious, like, are the people that are good scientists now in laboratory sciences? Just all mainly the people who are just really good at, you know, grantsmanship being able to write up these grants and get them funded and just have the stamina to just keep doing it. It exhausts me just working on one grant proposal.
Raghu Parthasarathy: Oh, I mean overwhelmingly yes. And I mean, I saw this, especially at Berkeley. I mean, to be a PI was being, you know, coming with almost a full-time grant writing machine. And you rely on being able to attract, you know, postdocs or others and kind of having a hierarchy of stuff. You know, here in my lab is small, you know, it's not hierarchical and I'm more connected to the actual data and stuff, but, but if you really want to be a you know, one of the large or particularly you know very successful groups that that's what you are. You're the grant writing machine.
I don't offend anybody with that, I just think it's more or less true. And it's, it is kind of sad because you have, these people are excellent scientists. We've almost taken away the thing that they came to fame for. So we've structured it in an odd way.
And I mean, it's kind of related to something that, you know, seems to increasingly affect even my work, it's very hard to plan kind of in the, in the long term. So you're running a grant, that's like a three-year or five-year grad. Plus, you know, your odds of getting it are like 10 to 20%. So given all of that, stochasticity, you know, it's just really, really hard to make plans for the long-term and to take a project that really likes long-term projects that you expect to have a reasonable chance of doing. It's a very strange system.
Steve Hsu: Yeah. So let's talk about that. Cause I think that's something we, you and I are both interested in. Because I could pose it in a provocative way. I could say how far, you know, how, just exactly how suboptimal is the system right now for producing great science? You know, like how far are we from the ideal system?
Raghu Parthasarathy: Yeah. Okay. I don't know what the metric is for distance to the ideal system.
Steve Hsu: How does it feel to you?
Raghu Parthasarathy: It feels like we're far and getting worse. And I think, you know, the things that me and that kind of increasingly bother me. One is what I just said, this issue of like, kind of stochasticity or, or short short-term planning. You just can't really plan for the long term, whether it's in terms of money or people or anything like that you're making, yeah, you, you're kind of limited by all this sort of short-term turn. and the one way, I guess, around that is to be a, a giant group at Berkeley or something and helping 40 people such that there's always something working out. Uh, and so on average you're okay. But that leads to a whole kind of factory system itself. So that stochasticity is, I think , one big thing.
The other, I think, and here, you know, I am probably biased by my perspective, as a faculty member at a, not top tier institution. I mean, I don't think that it is Christmas, Oregon, cause there's some phenomenal people, both faculty and students. But overall, you know, it, it, it is not in the elite, know, we, we, being the U.S. has set up this system where we basically just produce lots and lots of graduate students. And first of all, that leads to this again, kind of a short term thing. Like us, getting rid of the kind of accumulated skills and knowledge has, has, you know, students graduate.
And then to make it worse, we don't have a good place for them to graduate to. We kind of overproduce graduate students. It's not that easy for them to find jobs. You can, you know, they do. Okay. Especially because most of them don't go into programming and solids, but it's this bizarre wasteful use of, really the prime of people's lives. And it doesn't, it doesn't serve them. I mean, some of them, for some of them, it works out extremely well, but often it doesn't serve students well, and doesn't serve any kind of science in the long-term well.
As opposed to something where we had kind of more, kind of stable, longer term positions for people, even if there's fewer of them.
Steve Hsu: Right. So, I mean, one possibility is you could make the cut much earlier. You could say, okay. Only the very best undergraduates are going to be funded to do a PhD at all. But maybe their chances of continuing in the field are double or triple. On the other hand, the professors who are not at the elite institutions, then don't have a lot of graduate students around.
Raghu Parthasarathy: Right. I mean, I think the way you could do something like this is to have, you know, like, let's say my group, which on average has four to six, let's call it like five students in
Steve Hsu: um,
Raghu Parthasarathy: And, you know, I work hard to try to be able to fund that level. if, instead of that, it was, you know, the default is there'll be like one two, let's say kind of NSF funded staff, scientist positions, which I should say like a lot of students, especially with love if these kinds of things existed. And then, you know, one or two, let's call it one like graduate students. So a smaller number of, these, you know, relatively short term graduate student positions, but more, positions designed to be long term ones. And yeah, that, that I think would be a way to make all this work.
And we don't do that. I think, I mean, you know, I'm not a historian of science either, but you know, we came to this decision decades ago to like put a lot of research into, or have a lot of research be done at universities with graduate students as a way of just rapidly deploying kind of a research workforce, you know, post-World war II, when other critical options weren't there.
Steve Hsu: Yeah. I mean, I think some people who are particularly critical, I think Eric Weinstein is a particular example. I don't know if you know who he is, but.
Raghu Parthasarathy: Yeah.
Steve Hsu: He's extremely critical of our system. And would point to particular NSF reports that pointed out that this is what we were going to do. We were basically going to like, create a very dire job situation, et cetera, et cetera.
Raghu Parthasarathy: Yeah. You can get away with it while you're undergoing exponential growth.
Steve Hsu: Yes, but we haven't had that. But we stopped having exponential growth, I mean, generations ago. Right?
Raghu Parthasarathy: Oh, yeah. Yeah, exactly.
Steve Hsu: So
Raghu Parthasarathy: We still plug along with this, with this system. It's very bizarre.
Steve Hsu: I mean, another way of describing it though is, oh, well we just let a lot of flowers bloom. We let a lot of people into graduate school. If we have a good system for picking talent, then maybe we, we, you know, we, we're able to get people, people who maybe weren't at the very top at the end of their undergraduate career, they still have a shot.
And the people, as long as they know what their odds are of succeeding in a scientific career, then it's quite fair. Right? So, you're maybe, maybe you're exploiting the youthful idealism of people, but they at least in principle know what they're getting into.
Raghu Parthasarathy: Yeah. That's a tough one because yeah, to what extent. You know, you can ask them to be aware, but it's really hard to, to really be aware of what the structure of all of this is.
Steve Hsu: I agree. I agree. And I also think for a 22 year old to be able to think about, you know, how much risk they want to take on and what they, where they want to be when they're 30. It's hard for a kid to think that through, you know, they just don't have enough life experience.
Raghu Parthasarathy: Yeah. They don't know. Yeah, have enough life experience and also, you know, a lot of the perceptions that they may develop. Like many of them, I think, do come in, at least knowing that, okay, getting an academic job is very challenging. But they don't come in knowing things like, you know, how challenging an academic job actually is in terms of you're trying to get funding and things like that, or how difficult it is actually to find an industry job that's, you know, largely research based.
That's much harder than people think.
Steve Hsu: Yes.
Raghu Parthasarathy: All these sorts of aspects of it are not well known. And, you know, I could tolerate it more if I thought we were trying to educate people about all these things, but we don't really because it's hard to do. So we have this kind of a messy situation we currently do.
Steve Hsu: Well, as you know, I'm kind of an iconoclast. So I have, since the beginning of my career, been very vocal in educating students that wanted to go into theoretical physics about what their odds were. And what their likely career path was going to be. It's So, so I try to get them, you know, the information that, you know, I would want my own son to have were he embarking on that career path.
Raghu Parthasarathy: right? Yeah. I mean, I try to talk honestly with people as well.
Steve Hsu: But, but then the point we were just making is that even though we do, they're 22, so they're just optimistic and idealistic, nothing bad has ever happened to them. So...
Raghu Parthasarathy: Well. Also, they come in quite understandably with a kind of notion that, you know, surely the system makes sense.
Steve Hsu: Exactly. Right. They could
Raghu Parthasarathy: that yeah.
Steve Hsu: grinder. Right.
Raghu Parthasarathy: Right. Yeah. Or that, you know, not even a meat grinder, but like, I think for, I mean, I include myself in this. The realization that, you know, the overall structure hasn't been well thought out, it's just this kind of historical accident in a way. It's kind of a shocking one.
Steve Hsu: That, but that's a shocking discovery just in general in life, you realize our government runs the way it runs. Right. Even the Supreme court operates the way it operates.
Raghu Parthasarathy: Right.
Steve Hsu: Not, the
Raghu Parthasarathy: You're right. Actually that is a more general lesson.
Steve Hsu: Yeah. But you know, I'll tell you something funny when, when we were both in Eugene and our kids were young, I would have said, oh, I would love it if my son or daughter really wanted to be a scientist, it would be such a joy. But just to take an example, my son who's, who's both very bright, but you know, my son is I think could be a scientist. He has the right kind of mathematical bent and it could be a physicist. Doesn't seem to be that interested in it. I think he would rather, I think he already knows he'd rather go to Wall Street or something.
And it's almost a relief for me to not, to not think that even though I was originally envisioning and when I brought them up, I was trying to kind of educate them in that direction, but it's kind of a relief that he doesn't seem more bent on a scientific career. So...
Raghu Parthasarathy: Yeah. It's kinda sad to think that though, isn't it? I mean, at least for me, I feel like I love my job. I should point out. But I think it's gotten, it's definitely not the same, even as it was, you know, 15 years ago.
Steve Hsu: To clarify that, even to be an experimentalist in biophysics, has qualitatively changed in the last 10 or 15 years.
Raghu Parthasarathy: Yeah. Or it can be a bit more broad. That being an academic researcher being an academic faculty member doing research.
Steve Hsu: And how, how has it changed?
Raghu Parthasarathy: Well, I think that these issues of, you know, the vagaries of funding and things like that, and, you know, the kind of global question of, you know, what is, what is the structure of it all? And is it something that is good for those involved who have gotten more, more pointed in recent years.
Steve Hsu: Yes. I see. I think that the general thing I've heard a lot is just, it's harder and harder to get funding, to keep your lab going.
Raghu Parthasarathy: Yep.
Steve Hsu: I think once it becomes kind of an unpleasant, you know, struggle, right. Day-to-day struggle then, like how can you possibly still be excited about science? I mean, of course you can be even more excited about the moments when you get to do science, but, but overall you're kind of, I think enthusiasm that will have to be impacted by the grind.
Raghu Parthasarathy: Yeah. Yeah, I agree.
Steve Hsu: I'm kind of glad I'm at, I'm sort of at a point in my career now having done an administrative job for a while now I'm coming back to science. Now I'm super invigorated to do science and I don't feel any pressure on myself. Just, just, you know, I'm just doing the research that I don't want to do until I retire. So I feel very lucky to be in this position.
Raghu Parthasarathy: And I might add things have gone really nicely for my lab, even though, you know, it's hard to predict the future and so on. I've been really happy with how we've done. And it is true that I do keep like a little file on my computer. If I run out of all funding, what are really cheap experiments I could do, or things like dabble in myself or whatever, like what's the, what's the rainy day scenario or something like that.
Steve Hsu: Yeah. It's unfortunate that that's what people have to do, they have to have that backup plan.
Raghu Parthasarathy: Yeah.
Steve Hsu: Great. Well, I've, I've had you on for almost 90 minutes, so I should probably, let you go. Are there any final things you want to discuss before we sign off?
Raghu Parthasarathy: Not really. I think this has been really great. And, yeah, anybody, if anybody listening to this ends up having questions about biophysics or why it matters or anything like that, you know, I'm easy to find on the internet so you can feel free to send me a note.
Steve Hsu: Great. Fantastic. So in the show notes, I'll have a link to your book and your lab webpage and your blog. And it's been great to get back in touch with you Ragu, and I wish you all, all the best in the future.
Raghu Parthasarathy: Thanks. This has been a lot of fun and thanks again for having me.
Steve Hsu: Great.
Creators and Guests
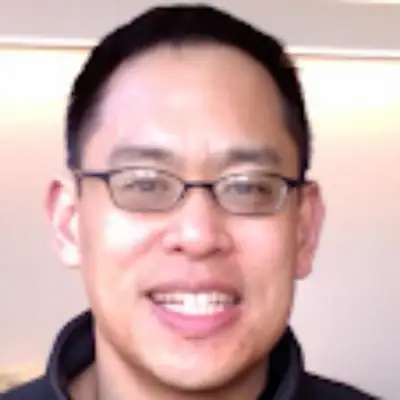